BiCycle: Item Recommendation with Life Cycles
2017 IEEE International Conference on Data Mining (ICDM)(2017)
摘要
Recommender systems have attracted much attention in last decades, which can help the users explore new items in many applications. As a popular technique in recommender systems, item recommendation works by recommending items to users based on their historical interactions. Conventional item recommendation methods usually assume that users and items are stationary, which is not always the case in real-world applications. Many time-aware item recommendation models have been proposed to take the temporal effects into the considerations based on the absolute time stamps associated with observed interactions. We show that using absolute time to model temporal effects can be limited in some circumstances. In this work, we propose to model the temporal dynamics of both users and items in item recommendation based on their life cycles. This problem is very challenging to solve since the users and items can co-evolve in their life cycles and the sparseness of the data become more severe when we consider the life cycles of both users and items. A novel time-aware item recommendation model called BiCycle is proposed to address these challenges. BiCycle is designed based on two important observations: 1) correlated users or items usually share similar patterns in the similar stages of their life cycles. 2) user preferences and item characters can evolve gradually over different stages of their life cycles. Extensive experiments conducted on three real-world datasets demonstrate the proposed approach can significantly improve the performance of recommendation tasks by considering the inner life cycles of both users and items.
更多查看译文
关键词
Item Recommendation,Collaborative Filtering,Life Cycle,Evolution Inference,Data Mining
AI 理解论文
溯源树
样例
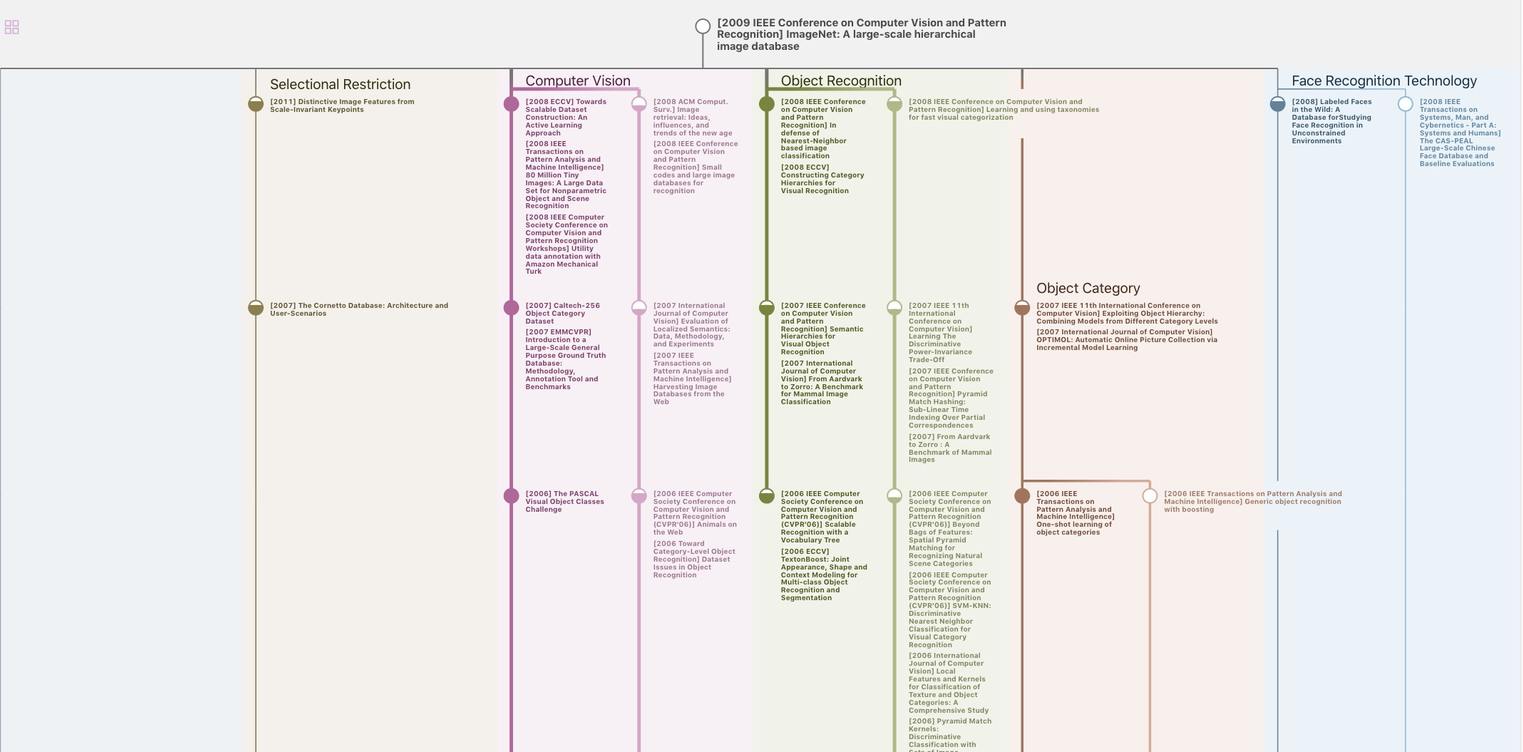
生成溯源树,研究论文发展脉络
Chat Paper
正在生成论文摘要