Combined feature extraction method for classification of EEG signals
Neural Computing and Applications(2016)
摘要
Classification of electroencephalogram (EEG) signals is an important task in brain–computer interfaces applications. This paper combines autoregressive (AR) model and sample entropy and presents a combination strategy of feature extraction. Each feature vector obtained from the combination strategy contains two parts: AR coefficients and sample entropy values. In the classification phase, this paper employs support vector machine (SVM) with RBF kernel as the classifier. The proposed method is used in the five mental task experiments. Experimental results show that the SVM classifier performs very well in classifying EEG signals using the combination strategy of feature extraction. It obtains a better accuracy in comparison with AR-based method. The results also indicate that the combination strategy of AR model and sample entropy can effectively improve the classification performance of EEG signals.
更多查看译文
关键词
EEG signal,Feature extraction,Autoregressive model,Sample entropy,Support vector classification,Brain–computer interfaces
AI 理解论文
溯源树
样例
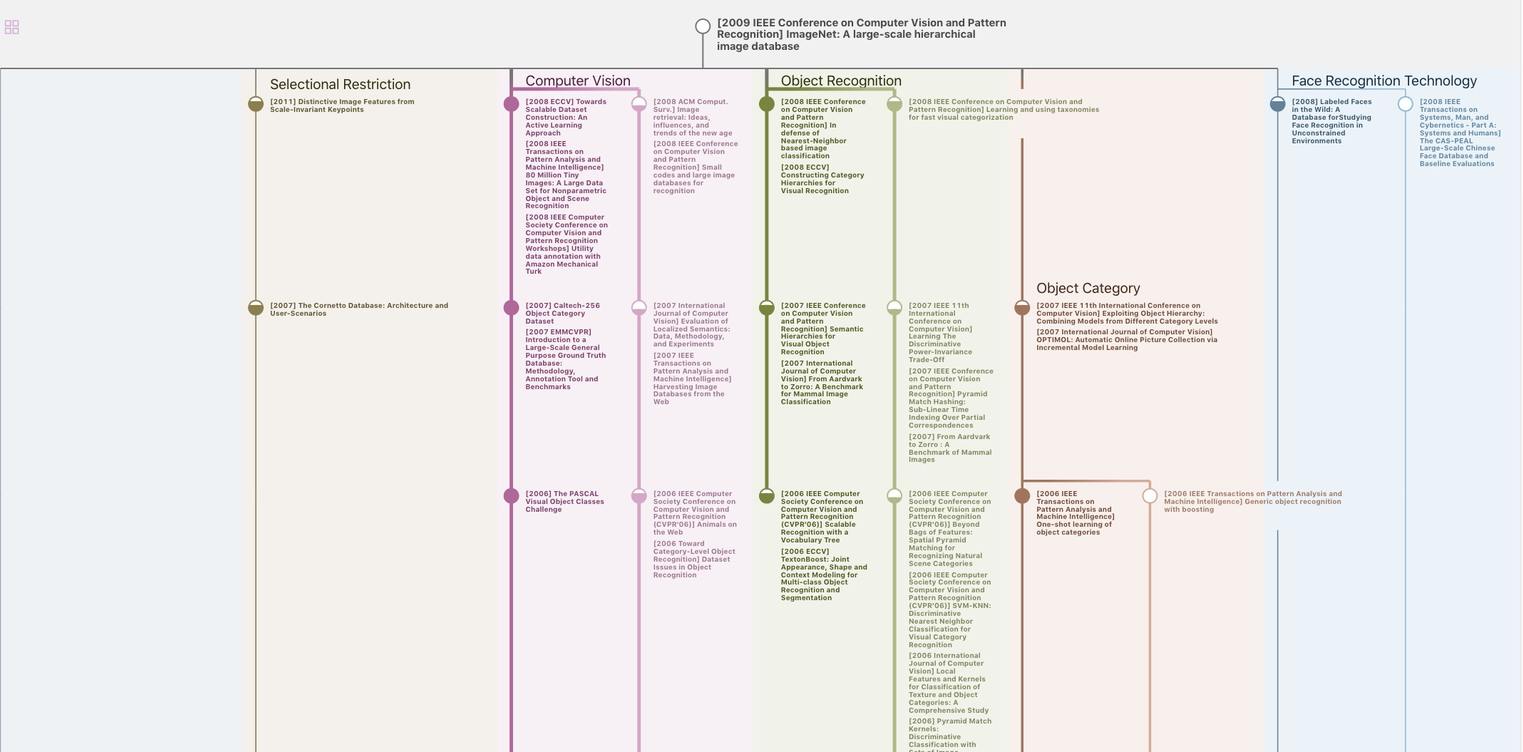
生成溯源树,研究论文发展脉络
Chat Paper
正在生成论文摘要