Recurrent Modeling Of Interaction Context For Collective Activity Recognition
30TH IEEE CONFERENCE ON COMPUTER VISION AND PATTERN RECOGNITION (CVPR 2017)(2017)
摘要
Modeling of high order interactional context, e.g., group interaction, lies in the central of collective/group activity recognition. However, most of the previous activity recognition methods do not offer a flexible and scalable scheme to handle the high order context modeling problem. To explicitly address this fundamental bottleneck, we propose a recurrent interactional context modeling scheme based on LSTM network. By utilizing the information propagation/ aggregation capability of LSTM, the proposed scheme unifies the interactional feature modeling process for single person dynamics, intra-group (e.g., persons within a group) and inter-group (e.g.,group to group) interactions. The proposed high order context modeling scheme produces more discriminative/descriptive interactional features. It is very flexible to handle a varying number of input instances (e.g.,different number of persons in a group or different number of groups) and linearly scalable to high order context modeling problem. Extensive experiments on two benchmark collective/group activity datasets demonstrate the effectiveness of the proposed method.
更多查看译文
关键词
flexible scheme,scalable scheme,high order context modeling problem,interactional feature modeling process,high order context modeling scheme,interaction context,collective activity recognition,group interaction,collective-group activity recognition,activity recognition methods,interactional context recurrent modeling scheme,discriminative-descriptive interactional features,benchmark collective-group activity datasets,single person dynamics
AI 理解论文
溯源树
样例
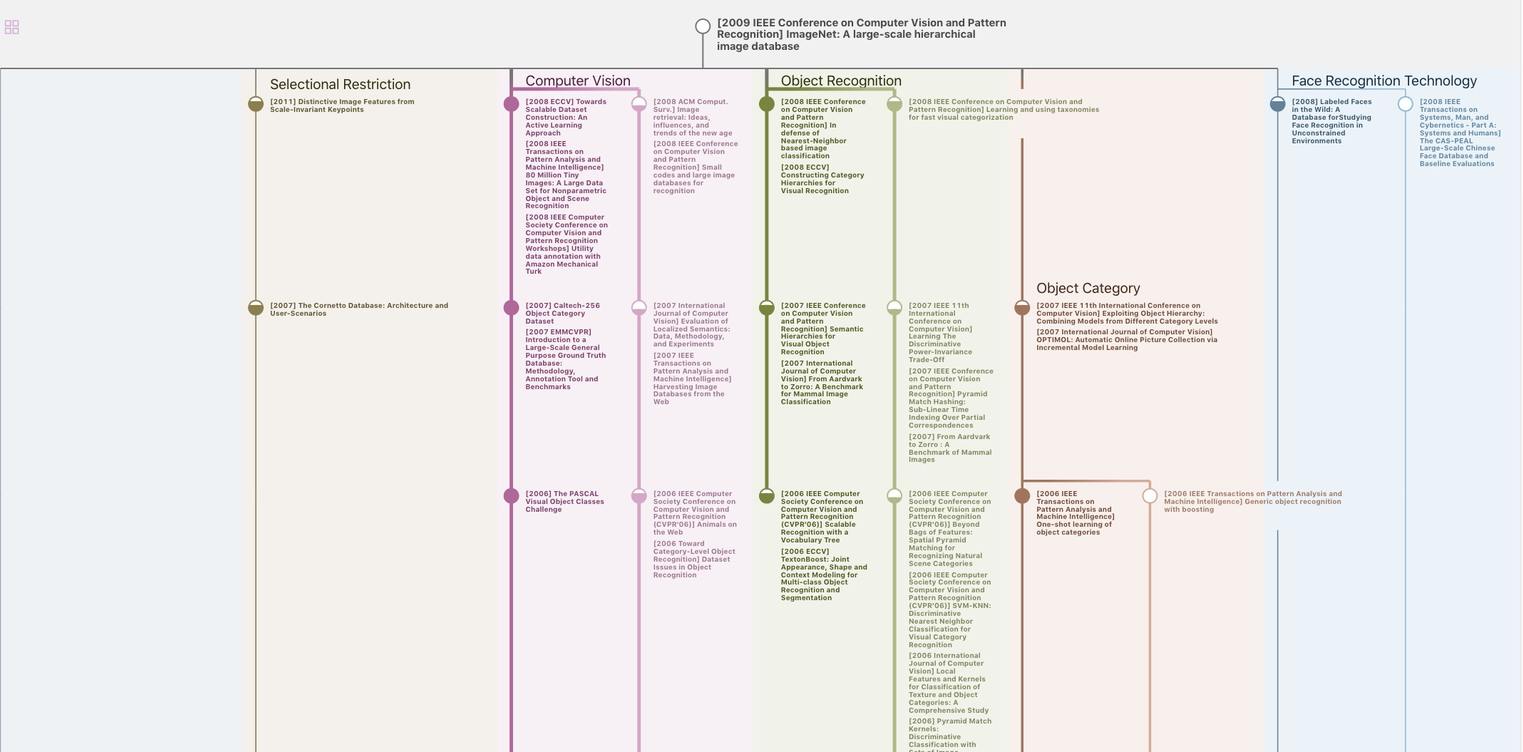
生成溯源树,研究论文发展脉络
Chat Paper
正在生成论文摘要