Exploiting Music Play Sequence for Music Recommendation.
IJCAI(2017)
摘要
Users leave digital footprints when interacting with various music streaming services. Music play sequence, which contains rich information about personal music preference and song similarity, has been largely ignored in previous music recom-mender systems. In this paper, we explore the effects of music play sequence on developing effective personalized music recommender systems. Towards the goal, we propose to use word embedding techniques in music play sequences to estimate the similarity between songs. The learned similarity is then embedded into matrix factorization to boost the latent feature learning and discovery. Furthermore, the proposed method only considers the k-nearest songs (e.g., k = 5) in the learning process and thus avoids the increase of time complexity. Experimental results on two public datasets demonstrate that our methods could significantly improve the performance on both rating prediction and top-n recommendation tasks.
更多查看译文
AI 理解论文
溯源树
样例
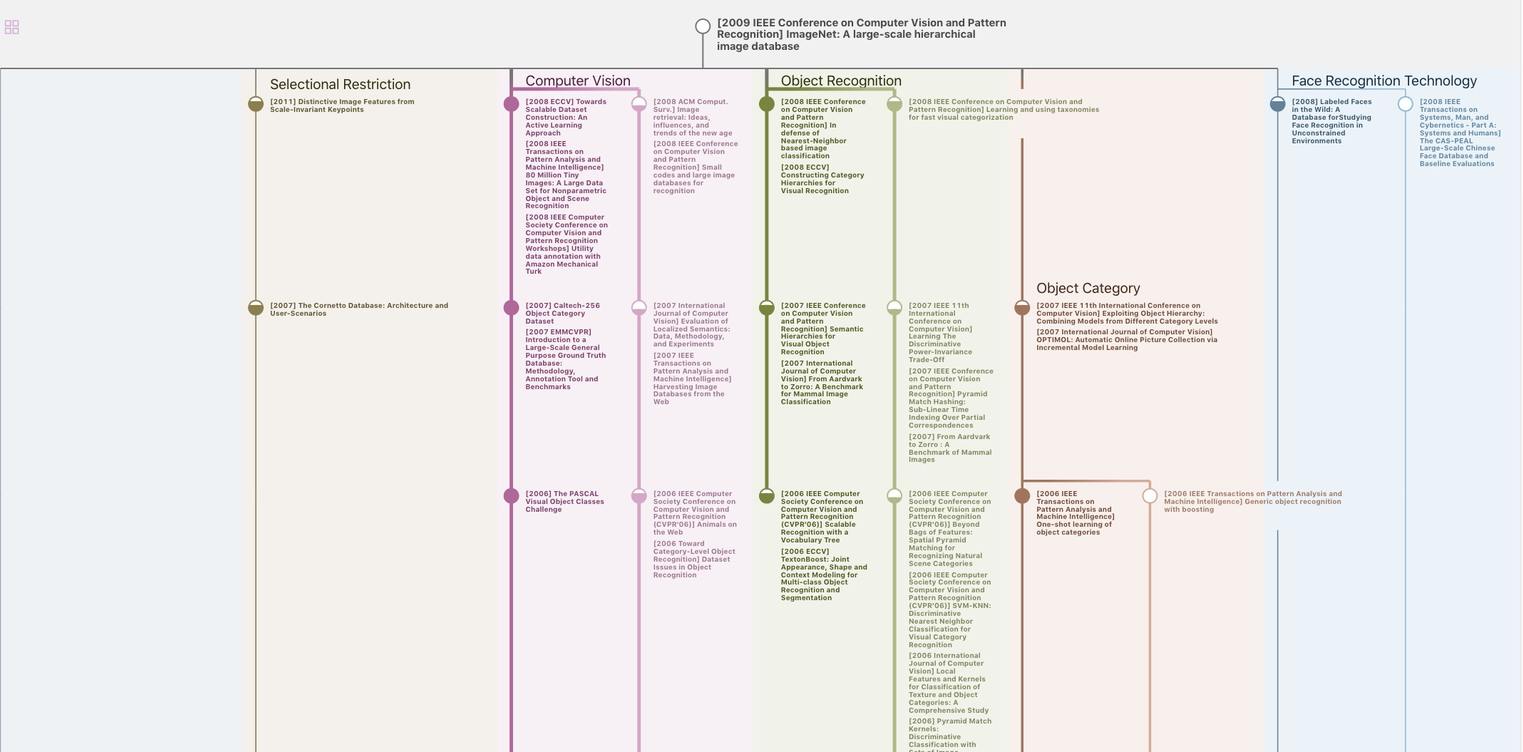
生成溯源树,研究论文发展脉络
Chat Paper
正在生成论文摘要