Boosted Tree Classifier For In Vivo Identification Of Early Cervical Cancer Using Multispectral Digital Colposcopy
PROCEEDINGS OF THE 10TH INTERNATIONAL JOINT CONFERENCE ON BIOMEDICAL ENGINEERING SYSTEMS AND TECHNOLOGIES, VOL 2: BIOIMAGING(2017)
摘要
Background: Cervical cancer develops over several years; screening and early diagnosis have decreased the incidence and mortality threefold over the last fifty years. Opportunities for the application of imaging and automation in the screening process exist in settings where resources are limited. Methods: Patients with high-grade squamous intraepithelial lesions (SIL) underwent imaging with a Multispectral Digital Colposcopy (MDC) prior to have a loop excision of the cervix. The image taken with white light was annotated by a clinician. The excised specimen was mapped by the study histopathologist blinded to the MDC data. This map was used to define areas of high grade in the excised tissue. Eleven reviewers mapped the histopathologic data into the MDC images. The reviewers' maps were analyzed and areas of agreement were calculated. We compared the result of a boosted tree classifier with a previously developed ensemble classifier. Results: Using a boosted tree classifier we obtained a sensitivity of 95%, a specificity of 96%, and an accuracy of 96% on the training sets. When we applied the classifier to a test set, we obtained a sensitivity of 82%, a specificity of 81%, and an accuracy of 81%. The boosted tree classifier performed better than the previously developed ensemble classifier. Conclusion: Here we presented promising results which show that a boosted tree analysis on MDC images is a method that could be used as an adjunct to colposcopy and would result in greater diagnostic accuracy compared to existing methods.
更多查看译文
关键词
Boosted Tree Classifier, Machine Learning, Image Processing, Multispectral Digital Colposcopy, Cervical Cancer
AI 理解论文
溯源树
样例
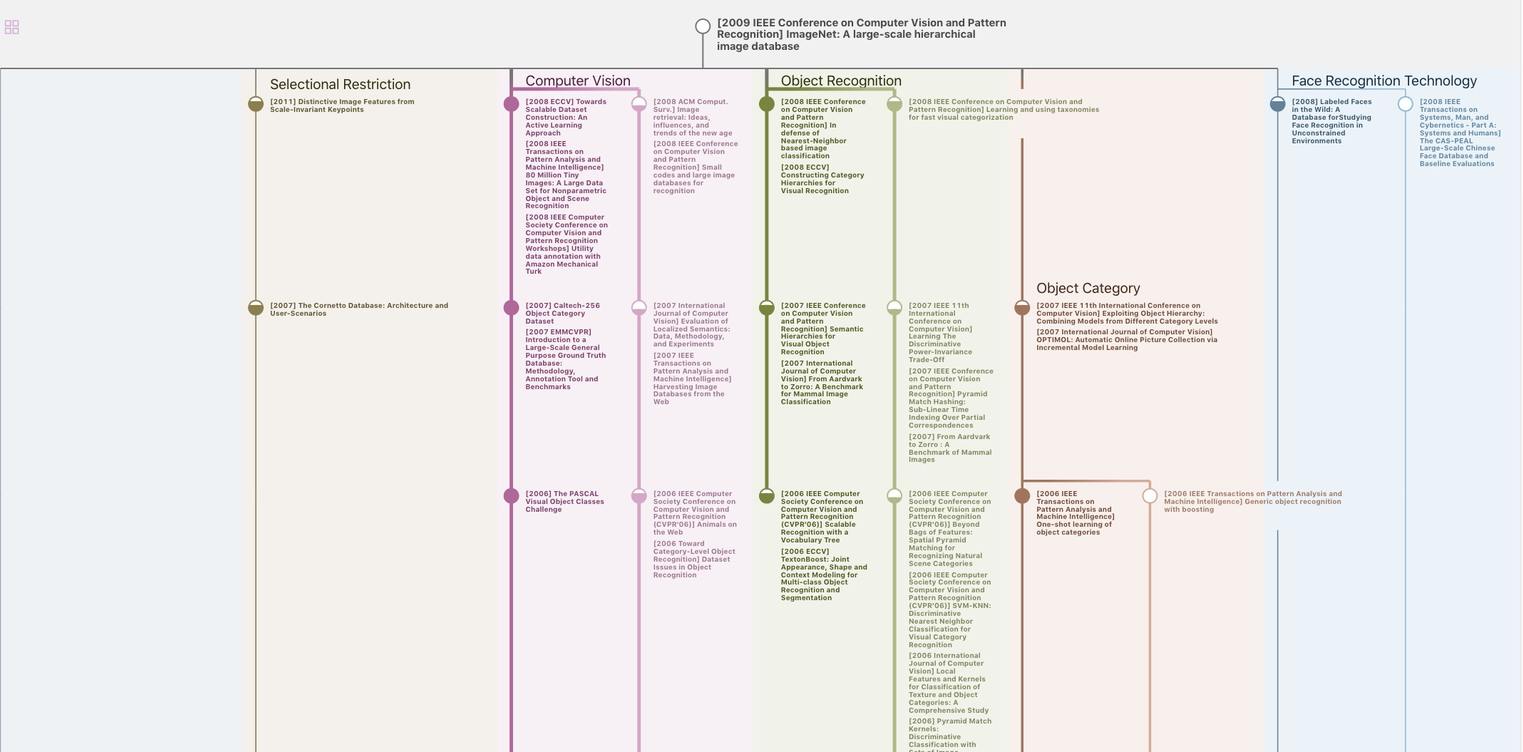
生成溯源树,研究论文发展脉络
Chat Paper
正在生成论文摘要