Multilateral surgical pattern cutting in 2D orthotropic gauze with deep reinforcement learning policies for tensioning.
ICRA(2017)
摘要
In the Fundamentals of Laparoscopic Surgery (FLS) standard medical training regimen, the Pattern Cutting task requires residents to demonstrate proficiency by maneuvering two tools, surgical scissors and tissue gripper, to accurately cut a circular pattern on surgical gauze suspended at the corners. Accuracy of cutting depends on tensioning, wherein the gripper pinches a point on the gauze in R 3 and pulls to induce and maintain tension in the material as cutting proceeds. An automated tensioning policy maps the current state of the gauze to output a direction of pulling as an action. The optimal tensioning policy depends on both the choice of pinch point and cutting trajectory. We explore the problem of learning a tensioning policy conditioned on specific cutting trajectories. Every timestep, we allow the gripper to react to the deformation of the gauze and progress of the cutting trajectory with a translation unit vector along an allowable set of directions. As deformation is difficult to analytically model and explicitly observe, we leverage deep reinforcement learning with direct policy search methods to learn tensioning policies using a finite-element simulator and then transfer them to a physical system. We compare the Deep RL tensioning policies with fixed and analytic (opposing the error vector with a fixed pinch point) policies on a set of 17 open and closed curved contours in simulation and 4 patterns in physical experiments with the da Vinci Research Kit (dVRK). Our simulation results suggest that learning to tension with Deep RL can significantly improve performance and robustness to noise and external forces.
更多查看译文
关键词
multilateral surgical pattern cutting,2D orthotropic gauze,deep reinforcement learning policies,fundamentals-of-laparoscopic surgery standard medical training regimen,FLS standard medical training regimen,surgical scissors,tissue gripper,surgical gauze,automated optimal tensioning policy,pinch point,cutting trajectory,tensioning policy conditioned learning,grippers,gauze deformation,translation unit vector,analytical model,explicit model,direct policy search methods,finite-element simulator,physical system,deep RL tensioning policies,open curved contours,closed curved contours,da Vinci Research Kit,dVRK,performance improvement,noise robustness improvement,external force robustness improvement
AI 理解论文
溯源树
样例
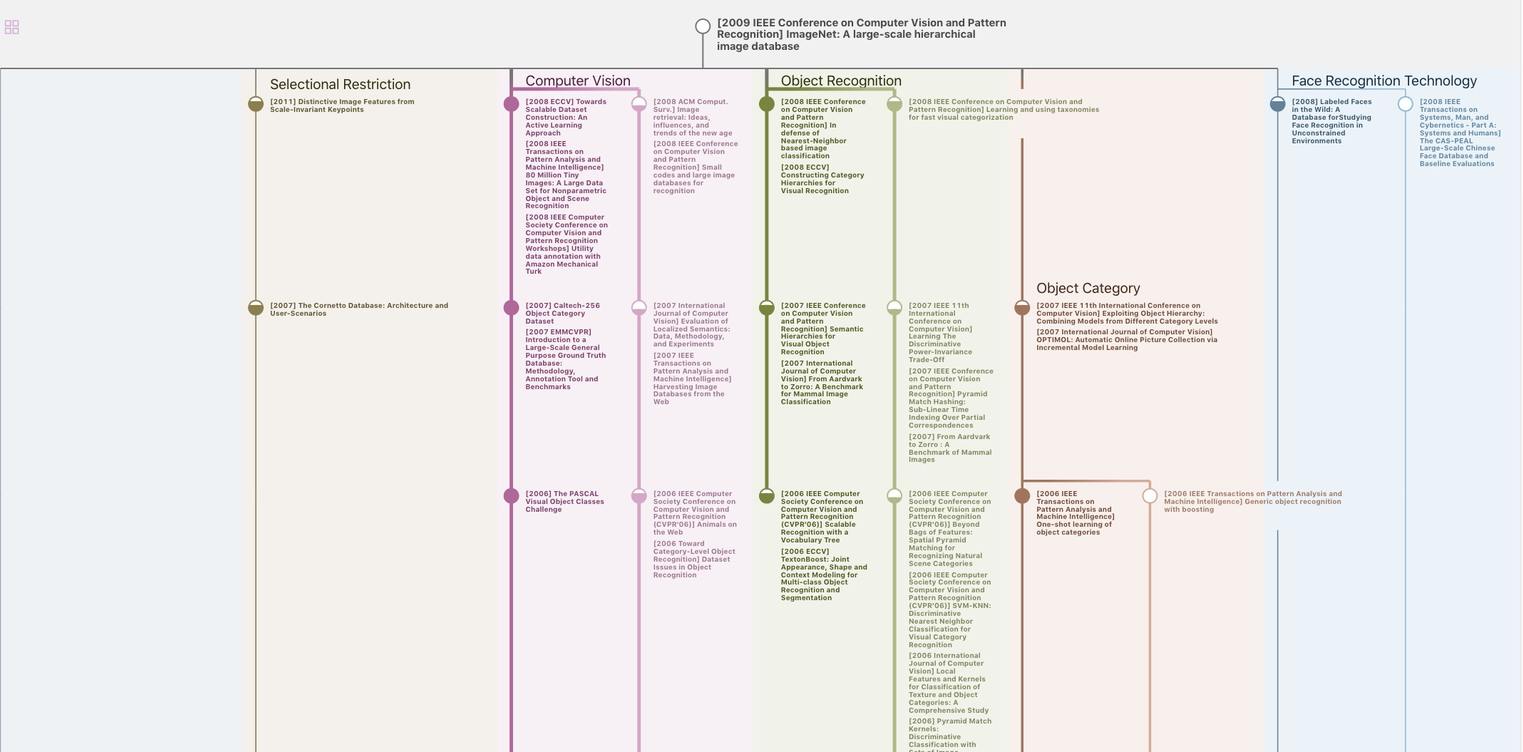
生成溯源树,研究论文发展脉络
Chat Paper
正在生成论文摘要