Guilt-free data reuse.
Commun. ACM(2017)
摘要
Existing approaches to ensuring the validity of inferences drawn from data assume a fixed procedure to be performed, selected before the data are examined. Yet the practice of data analysis is an intrinsically interactive and adaptive process: new analyses and hypotheses are proposed after seeing the results of previous ones, parameters are tuned on the basis of obtained results, and datasets are shared and reused. In this work, we initiate a principled study of how to guarantee the validity of statistical inference in adaptive data analysis. We demonstrate new approaches for addressing the challenges of adaptivity that are based on techniques developed in privacy-preserving data analysis. As an application of our techniques we give a simple and practical method for reusing a holdout (or testing) set to validate the accuracy of hypotheses produced adaptively by a learning algorithm operating on a training set.
更多查看译文
AI 理解论文
溯源树
样例
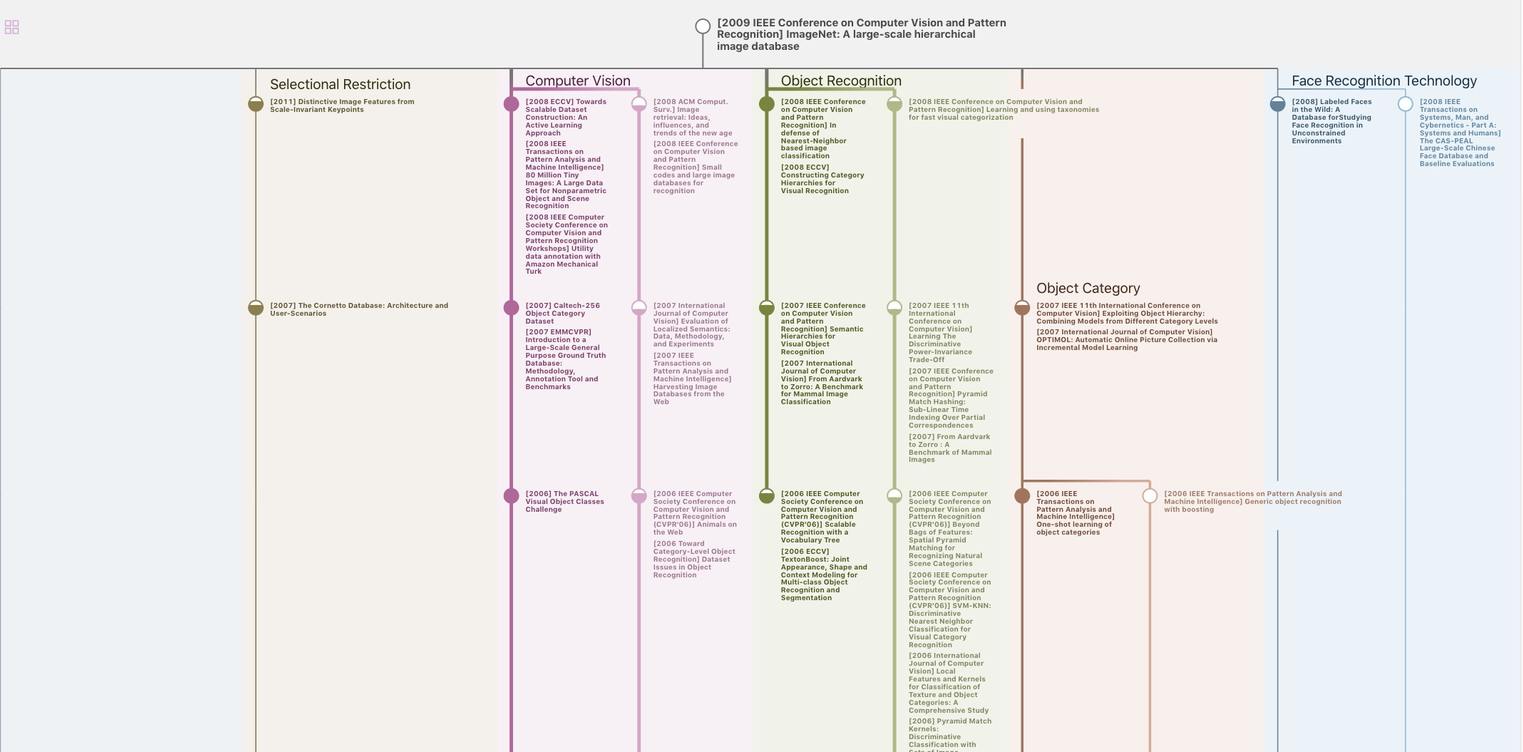
生成溯源树,研究论文发展脉络
Chat Paper
正在生成论文摘要