Recognition Oriented Facial Image Quality Assessment Via Deep Convolutional Neural Network
ICIMCS(2019)
摘要
Quality of facial images significantly impacts the performance of face recognition algorithms. Being able to predict "which facial image is good for recognition" is of great importance for real application scenarios, where a sequence of facial images are always presented and one should select the image frame with "best quality" for the subsequent matching and recognition task. To this end, we introduce a novel facial image quality automatic assessment framework directly targeting at "selecting better face image for better face recognition". For such as purpose, a deep convolutional neural network (DCNN) is trained to output a general facial quality metric which comprehensively considers various quality factors including brightness, contrast, blurriness, occlusion, and pose etc. Based on this trained facial quality metric network, we are able to sort the input face images accordingly and "select" good face images for recognition. Our method is comprehensively evaluated on Color FERET and KinectFace face datasets. Results show that the proposed facial image quality metric network works end-to-end and it well distinguishes "good" images from "bad" ones, which is highly correlated with the final recognition performance. (C) 2019 Published by Elsevier B.V.
更多查看译文
关键词
Face image quality,Face selection,Face recognition,Convolutional network
AI 理解论文
溯源树
样例
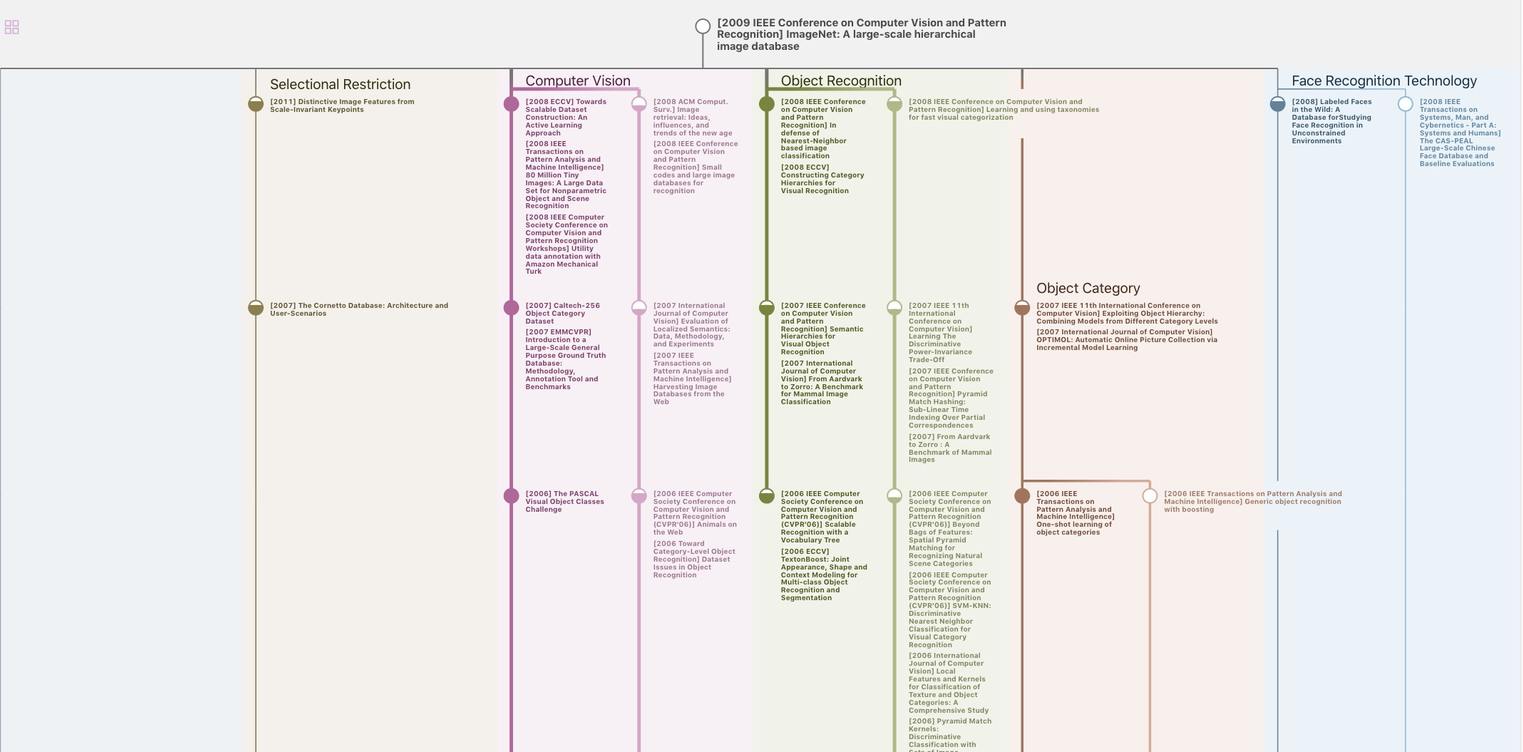
生成溯源树,研究论文发展脉络
Chat Paper
正在生成论文摘要