Backtracking Scspm Image Classifier For Weakly Supervised Top-Down Saliency
2016 IEEE Conference on Computer Vision and Pattern Recognition (CVPR)(2016)
摘要
Top-down saliency models produce a probability map that peaks at target locations specified by a task/goal such as object detection. They are usually trained in a supervised setting involving annotations of objects. We propose a weakly supervised top-down saliency framework using only binary labels that indicate the presence/absence of an object in an image. First, the probabilistic contribution of each image patch to the confidence of an ScSPM-based classifier produces a Reverse-ScSPM (R-ScSPM) saliency map. Neighborhood information is then incorporated through a contextual saliency map which is estimated using logistic regression learnt on patches having high R-ScSPM saliency. Both the saliency maps are combined to obtain the final saliency map. We evaluate the performance of the proposed weakly supervised top-down saliency and achieves comparable performance with fully supervised approaches. Experiments are carried out on 5 challenging datasets across 3 different applications.
更多查看译文
关键词
ScSPM image classifier,weakly supervised top-down saliency framework,binary labels,image patch,reverse-ScSPM,R-ScSPM saliency map,contextual saliency map,logistic regression,sparse coding-based spatial pyramid matching
AI 理解论文
溯源树
样例
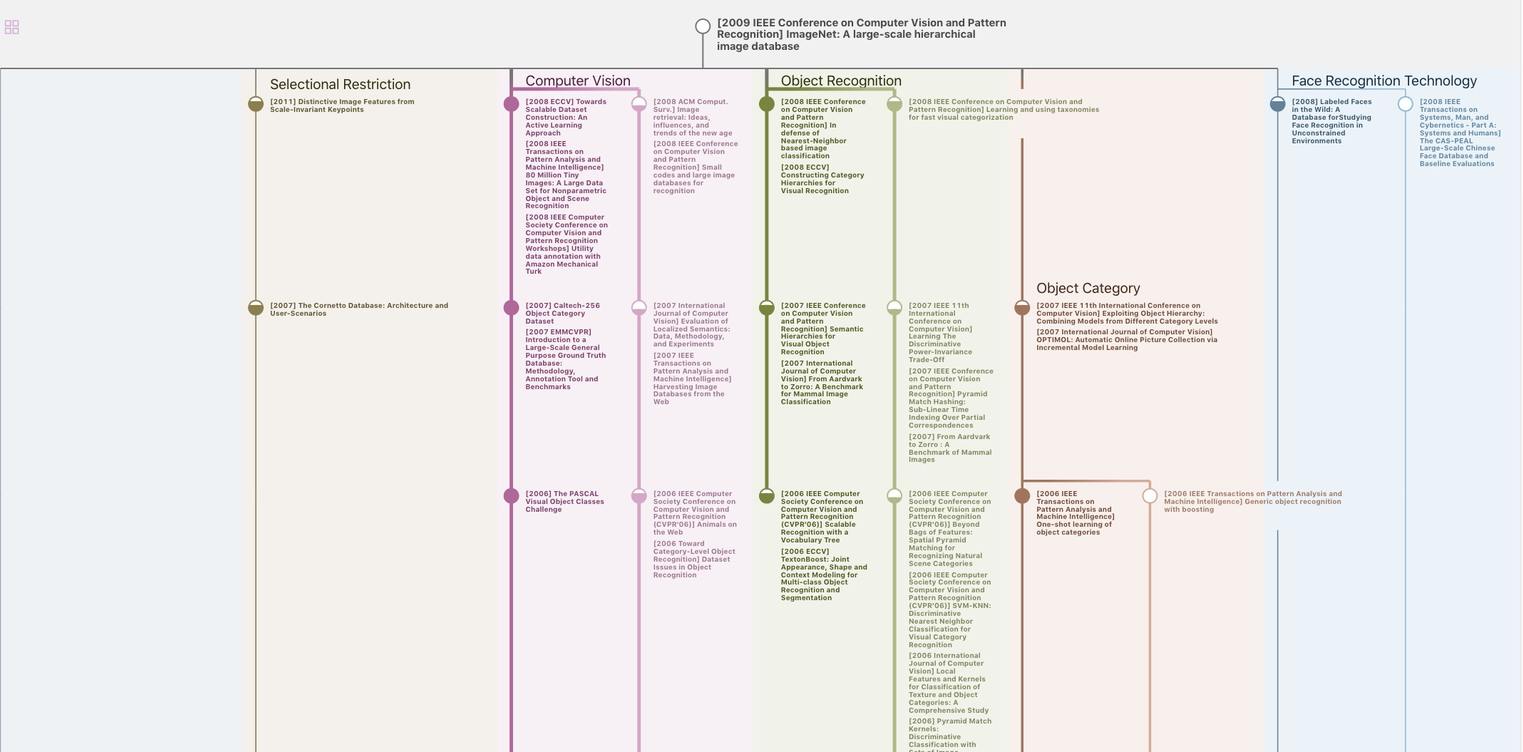
生成溯源树,研究论文发展脉络
Chat Paper
正在生成论文摘要