Learning Parametric Models For Context-Aware Query Auto-Completion Via Hawkes Processes
WSDM(2017)
摘要
Query auto completion (QAC) is a prominent feature in modern search engines. High quality QAC substantially improves search experiences by helping users in typing less while submitting the queries. Many studies have been proposed to improve quality and relevance of the QAC methods from different perspectives, including leveraging contexts in long term and short term query histories, investigating the temporal information for time-sensitive QAC, and analyzing user behaviors. Although these studies have shown the context, temporal, and user behavior data carry valuable information, most existing QAC approaches do not fully exploit or even completely ignore these information. We propose a novel Hawkes process based QAC algorithm, comprehensively taking into account the context, temporal, and position of the clicked recommended query completions (a type of user behavior data), for reliable query completion prediction. Our understanding of ranking query completions is consistent with the mathematical rationale of Hawke process; such a coincidence in turn validates our motivation of using Hawkes process for QAC. We also develop an efficient inference algorithm to compute the optimal solutions of the proposed QAC algorithm. The proposed method is evaluated on two real-world benchmark data in comparison with state-of-art methods, and the obtained experiments clearly demonstrate their effectiveness.
更多查看译文
关键词
query autocompletion,Hawkes process,contextual data
AI 理解论文
溯源树
样例
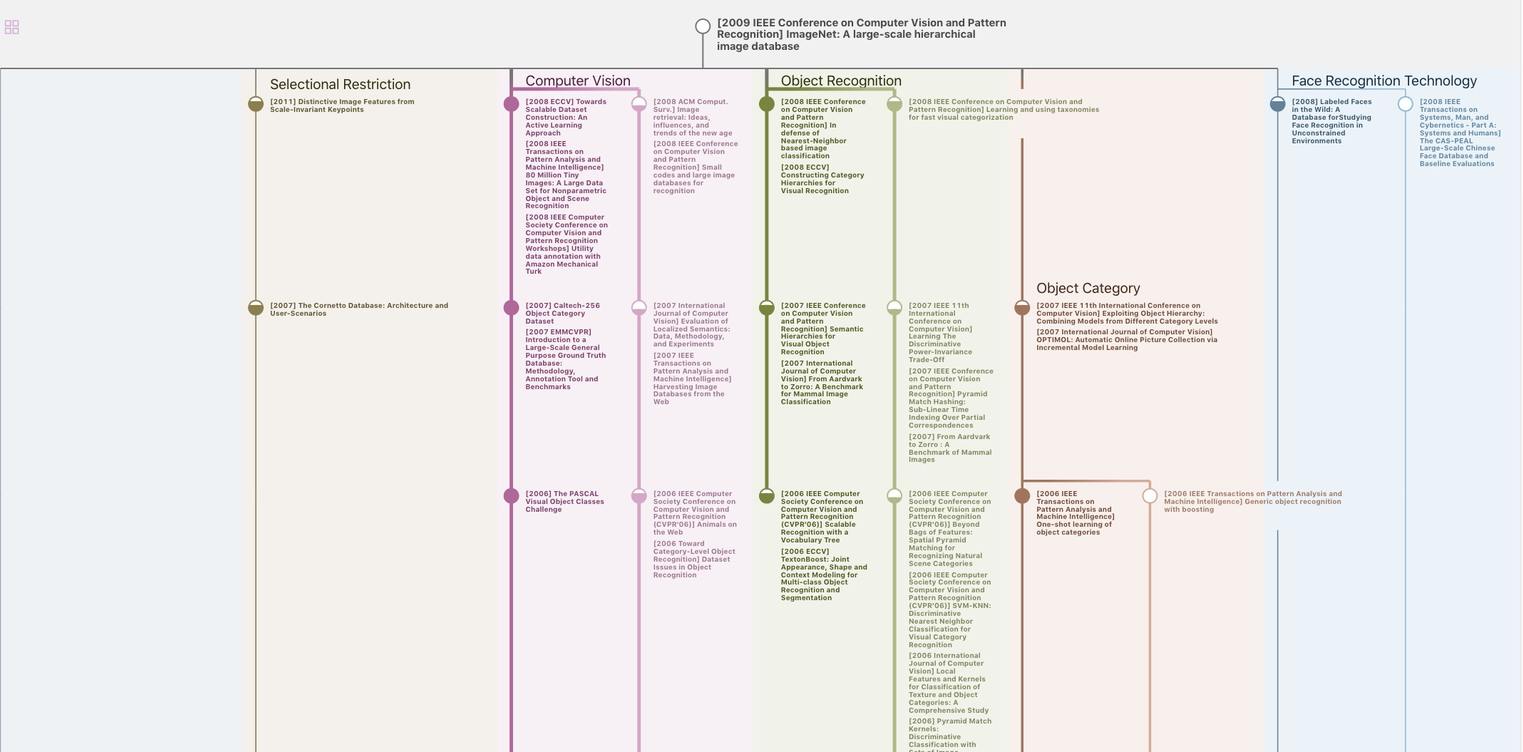
生成溯源树,研究论文发展脉络
Chat Paper
正在生成论文摘要