An N log N Parallel Fast Direct Solver for Kernel Matrices
2017 IEEE International Parallel and Distributed Processing Symposium (IPDPS)(2017)
摘要
Kernel matrices appear in machine learning and non-parametric statistics. Given N points in d dimensions and a kernel function that requires 𝒪(d) work to evaluate, we present an 𝒪(dNlog N)-work algorithm for the approximate factorization of a regularized kernel matrix, a common computational bottleneck in the training phase of a learning task. With this factorization, solving a linear system with a kernel matrix can be done with 𝒪(Nlog N) work. Our algorithm only requires kernel evaluations and does not require that the kernel matrix admits an efficient global low rank approximation. Instead our factorization only assumes low-rank properties for the off-diagonal blocks under an appropriate row and column ordering. We also present a hybrid method that, when the factorization is prohibitively expensive, combines a partial factorization with iterative methods. As a highlight, we are able to approximately factorize a dense 11M×11M kernel matrix in 2 minutes on 3,072 x86 "Haswell" cores and a 4.5M×4.5M matrix in 1 minute using 4,352 "Knights Landing" cores.
更多查看译文
关键词
parallel algorithms,machine learning,kernel methods,linear solvers,treecodes
AI 理解论文
溯源树
样例
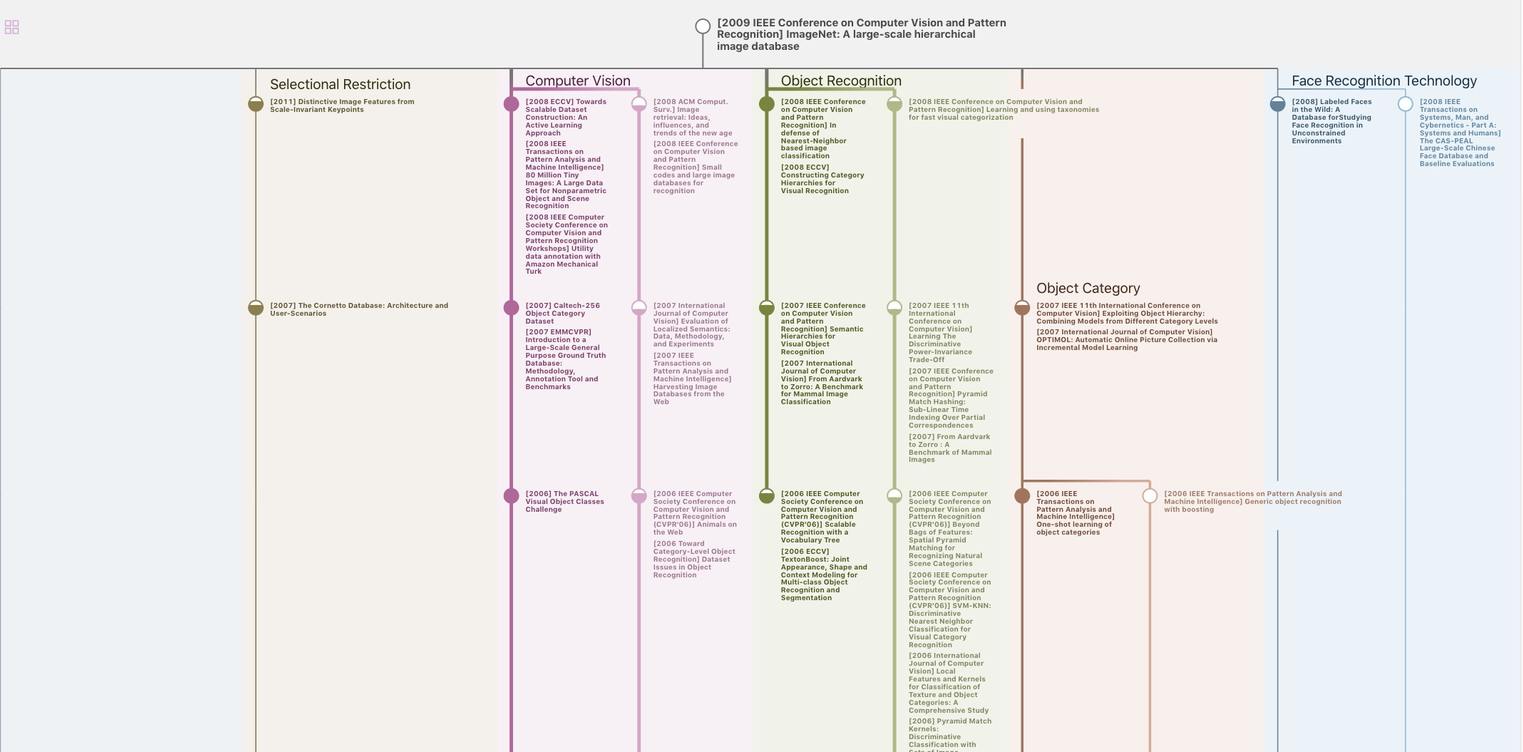
生成溯源树,研究论文发展脉络
Chat Paper
正在生成论文摘要