Learning Informative Priors from Heterogeneous Domains to Improve Recommendation in Cold-Start User Domains.
ACM Transactions on Information Systems (TOIS)(2016)
摘要
In the real-world environment, users have sufficient experience in their focused domains but lack experience in other domains. Recommender systems are very helpful for recommending potentially desirable items to users in unfamiliar domains, and cross-domain collaborative filtering is therefore an important emerging research topic. However, it is inevitable that the cold-start issue will be encountered in unfamiliar domains due to the lack of feedback data. The Bayesian approach shows that priors play an important role when there are insufficient data, which implies that recommendation performance can be significantly improved in cold-start domains if informative priors can be provided. Based on this idea, we propose a Weighted Irregular Tensor Factorization (WITF) model to leverage multi-domain feedback data across all users to learn the cross-domain priors w.r.t. both users and items. The features learned from WITF serve as the informative priors on the latent factors of users and items in terms of weighted matrix factorization models. Moreover, WITF is a unified framework for dealing with both explicit feedback and implicit feedback. To prove the effectiveness of our approach, we studied three typical real-world cases in which a collection of empirical evaluations were conducted on real-world datasets to compare the performance of our model and other state-of-the-art approaches. The results show the superiority of our model over comparison models.
更多查看译文
关键词
Recommender systems,cross-domain collaborative filtering,weighted irregular tensor factorization,probabilistic matrix factorization,multi-task learning
AI 理解论文
溯源树
样例
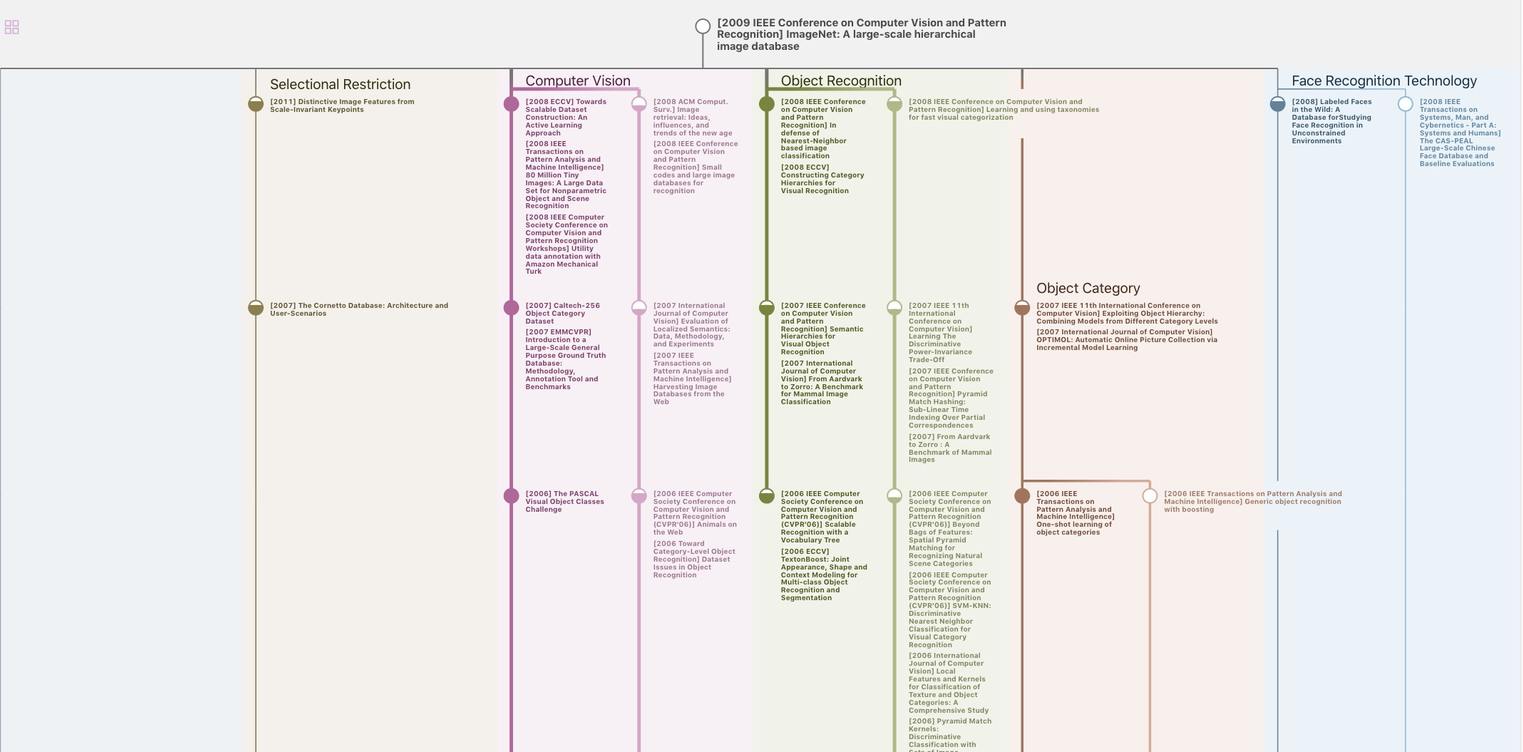
生成溯源树,研究论文发展脉络
Chat Paper
正在生成论文摘要