Instance-dependent Regret Bounds for Dueling Bandits.
COLT(2016)
摘要
We study the multi-armed dueling bandit problem in which feedback is provided in the form of relative comparisons between pairs of actions, with the goal of eventually learning to select actions that are close to the best. Following Dud´ ik et al. (2015), we aim for algorithms whose performance approaches that of the optimal randomized choice of actions, the von Neumann winner, expressly avoiding more restrictive assumptions, for instance, regarding the existence of a single best action (a Condorcet winner). In this general setting, the best known algorithms achieve regret ~ O( p KT ) inT rounds withK actions. In this paper, we present the first instance-dependent regret bounds for the general problem, focusing particularly on when the von Neumann winner is sparse. Specifically, we propose a new algorithm whose regret, relative to a unique von Neumann winner with sparsitys, is at most ~ O( p sT ), plus an instance-dependent constant. Thus, when the sparsity is much smaller
更多查看译文
AI 理解论文
溯源树
样例
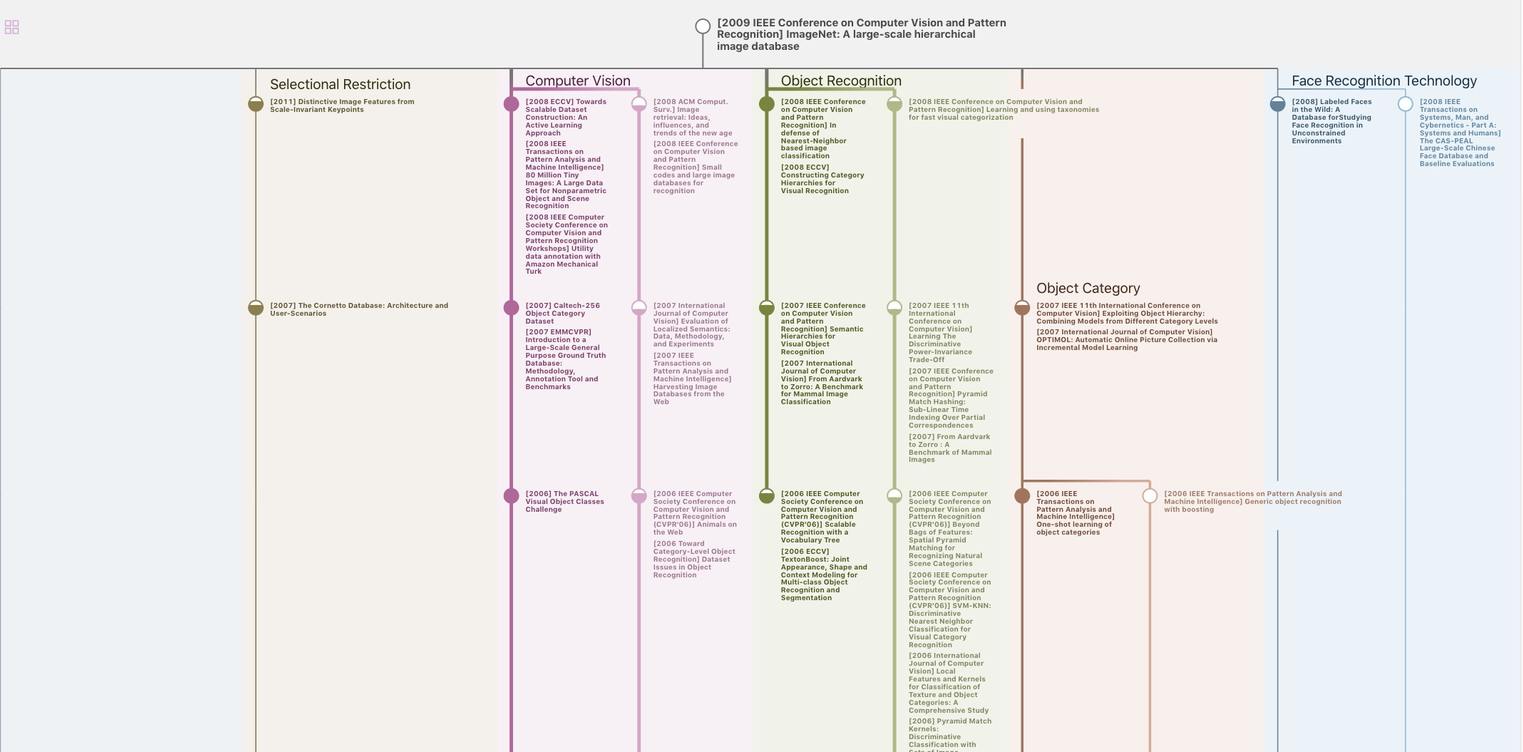
生成溯源树,研究论文发展脉络
Chat Paper
正在生成论文摘要