HIRL: Hierarchical Inverse Reinforcement Learning for Long-Horizon Tasks with Delayed Rewards.
arXiv: Robotics(2016)
摘要
Reinforcement Learning (RL) struggles in problems with delayed rewards, and one approach is to segment the task into sub-tasks with incremental rewards. propose a framework called Hierarchical Inverse Reinforcement Learning (HIRL), which is a model for learning sub-task structure from demonstrations. HIRL decomposes the task into sub-tasks based on transitions that are consistent across demonstrations. These transitions are defined as changes in local linearity w.r.t to a kernel function. Then, HIRL uses the inferred structure to learn reward functions local to the sub-tasks but also handle any global dependencies such as sequentiality. We have evaluated HIRL on several standard RL benchmarks: Parallel Parking with noisy dynamics, Two-Link Pendulum, 2D Noisy Motion Planning, and a Pinball environment. In the parallel parking task, we find that rewards constructed with HIRL converge to a policy with an 80% success rate in 32% fewer time-steps than those constructed with Maximum Entropy Inverse RL (MaxEnt IRL), and with partial state observation, the policies learned with IRL fail to achieve this accuracy while HIRL still converges. further find that that the rewards learned with HIRL are robust to environment noise where they can tolerate 1 stdev. of random perturbation in the poses in the environment obstacles while maintaining roughly the same convergence rate. find that HIRL rewards can converge up-to 6x faster than rewards constructed with IRL.
更多查看译文
AI 理解论文
溯源树
样例
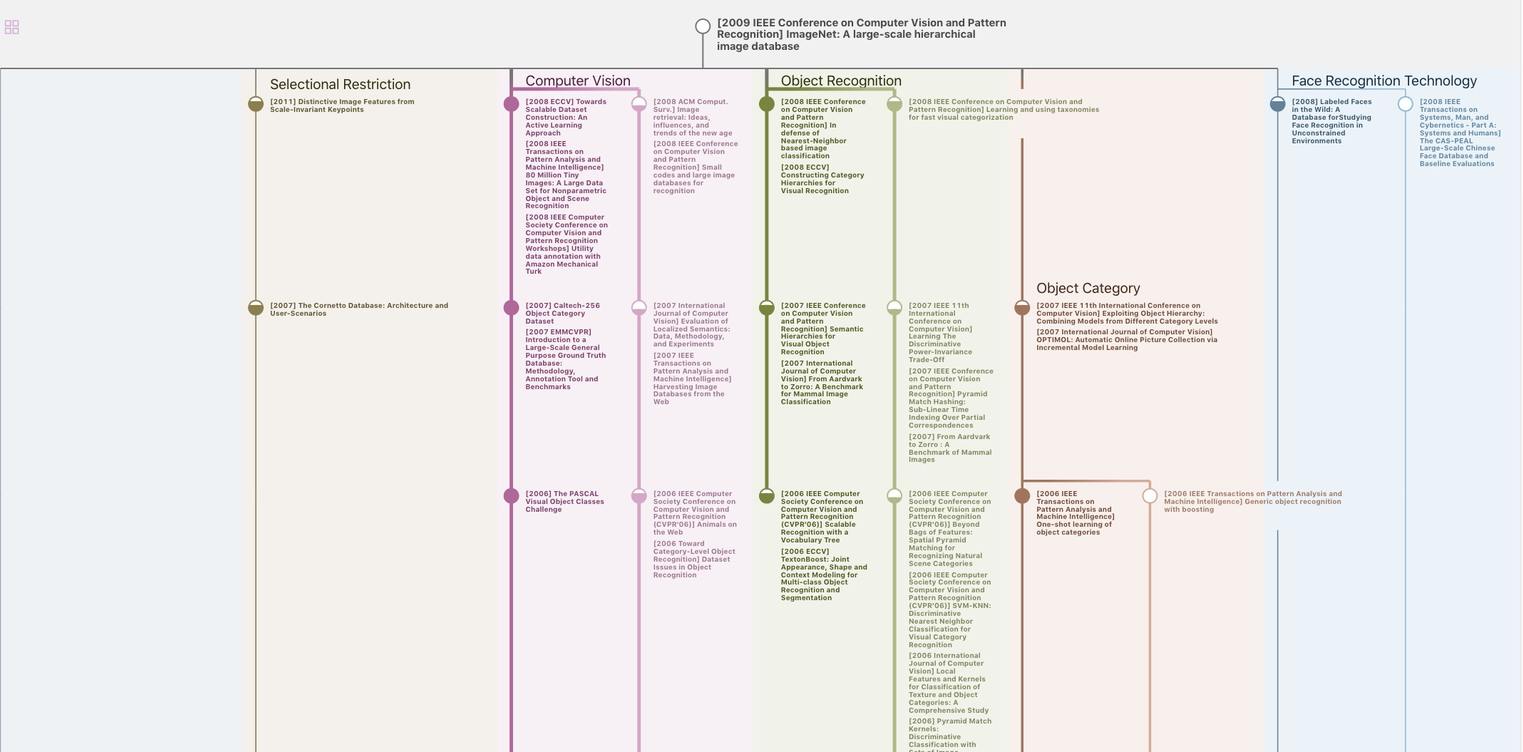
生成溯源树,研究论文发展脉络
Chat Paper
正在生成论文摘要