A Kernel-Independent Fmm In General Dimensions
SC15: The International Conference for High Performance Computing, Networking, Storage and Analysis Austin Texas November, 2015(2015)
摘要
We introduce a general-dimensional, kernel-independent, algebraic fast multipole method and apply it to kernel regression. The motivation for this work is the approximation of kernel matrices, which appear in mathematical physics, approximation theory, non-parametric statistics, and machine learning. Existing fast multipole methods are asymptotically optimal, but the underlying constants scale quite badly with the ambient space dimension. We introduce a method that mitigates this shortcoming; it only requires kernel evaluations and scales well with the problem size, the number of processors, and the ambient dimension-as long as the intrinsic dimension of the dataset is small. We test the performance of our method on several synthetic datasets. As a highlight, our largest run was on an image dataset with 10 million points in 246 dimensions.
更多查看译文
关键词
Machine learning,kernel methods,N-body problems,parallel algorithms,data mining,kernel ridge regression
AI 理解论文
溯源树
样例
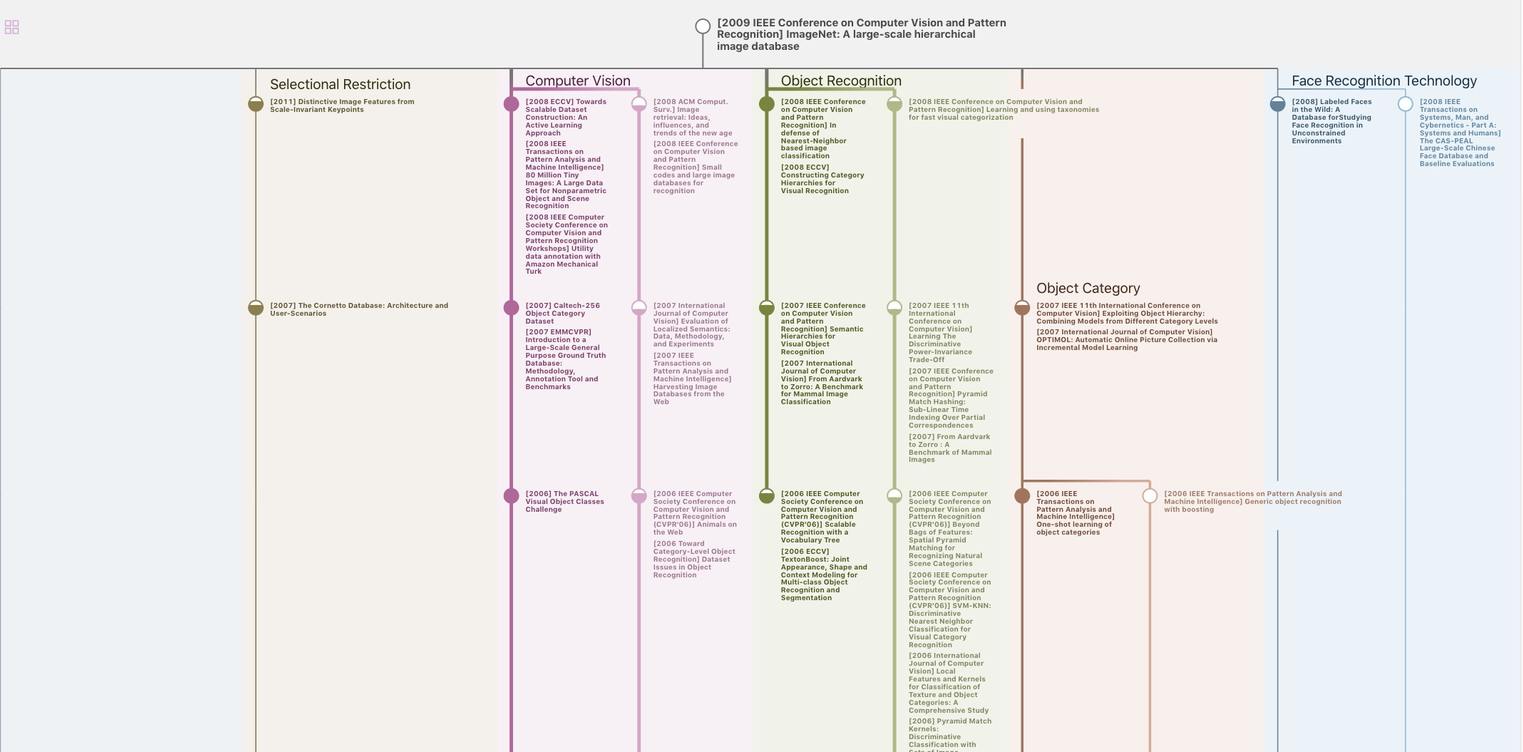
生成溯源树,研究论文发展脉络
Chat Paper
正在生成论文摘要