SemanticPaint: interactive segmentation and learning of 3D world
SIGGRAPH Talks(2015)
摘要
We present a real-time, interactive system for the geometric reconstruction, object-class segmentation and learning of 3D scenes [Valentin et al. ]. Using our system, a user can walk into a room wearing a consumer depth camera and a virtual reality headset, and both densely reconstruct the 3D scene [Nießner et al. 2013]) and interactively segment the environment into object classes such as 'chair', 'floor' and 'table'. The user interacts physically with the real-world scene, touching or pointing at objects and using voice commands to assign them appropriate labels. These user generated labels are leveraged by a new online random forest-based machine learning algorithm, which is used to predict labels for previously unseen parts of the scene. The predicted labels, together with those provided directly by the user, are incorporated into a dense 3D conditional random field model, over which we perform mean-field inference to filter out label inconsistencies. The entire pipeline runs in real time, and the user stays 'in the loop' throughout the process, receiving immediate feedback about the progress of the labelling and interacting with the scene as necessary to refine the predicted segmentation.
更多查看译文
AI 理解论文
溯源树
样例
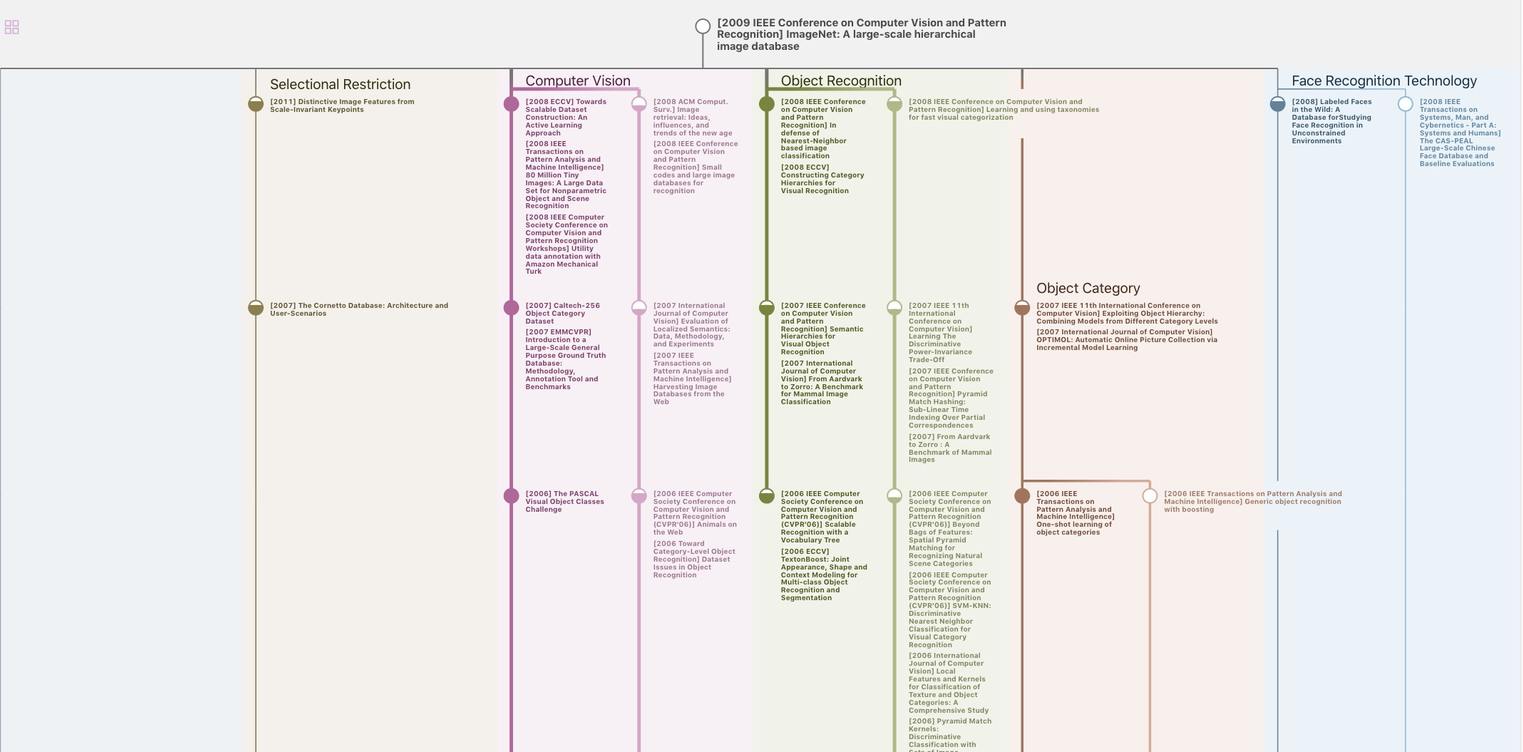
生成溯源树,研究论文发展脉络
Chat Paper
正在生成论文摘要