Manipulated Object Proposal: A Discriminative Object Extraction and Feature Fusion Framework for First-Person Daily Activity Recognition
CoRR(2015)
摘要
Detecting and recognizing objects interacting with humans lie in the center of first-person (egocentric) daily activity recognition. However, due to noisy camera motion and frequent changes in viewpoint and scale, most of the previous egocentric action recognition methods fail to capture and model highly discriminative object features. In this work, we propose a novel pipeline for first-person daily activity recognition, aiming at more discriminative object feature representation and object-motion feature fusion. Our object feature extraction and representation pipeline is inspired by the recent success of object hypotheses and deep convolutional neural network based detection frameworks. Our key contribution is a simple yet effective manipulated object proposal generation scheme. This scheme leverages motion cues such as motion boundary and motion magnitude (in contrast, camera motion is usually considered as "noise" for most previous methods) to generate a more compact and discriminative set of object proposals, which are more closely related to the objects which are being manipulated. Then, we learn more discriminative object detectors from these manipulated object proposals based on region-based convolutional neural network (R-CNN). Meanwhile, we develop a network based feature fusion scheme which better combines object and motion features. We show in experiments that the proposed framework significantly outperforms the state-of-the-art recognition performance on a challenging first-person daily activity benchmark.
更多查看译文
AI 理解论文
溯源树
样例
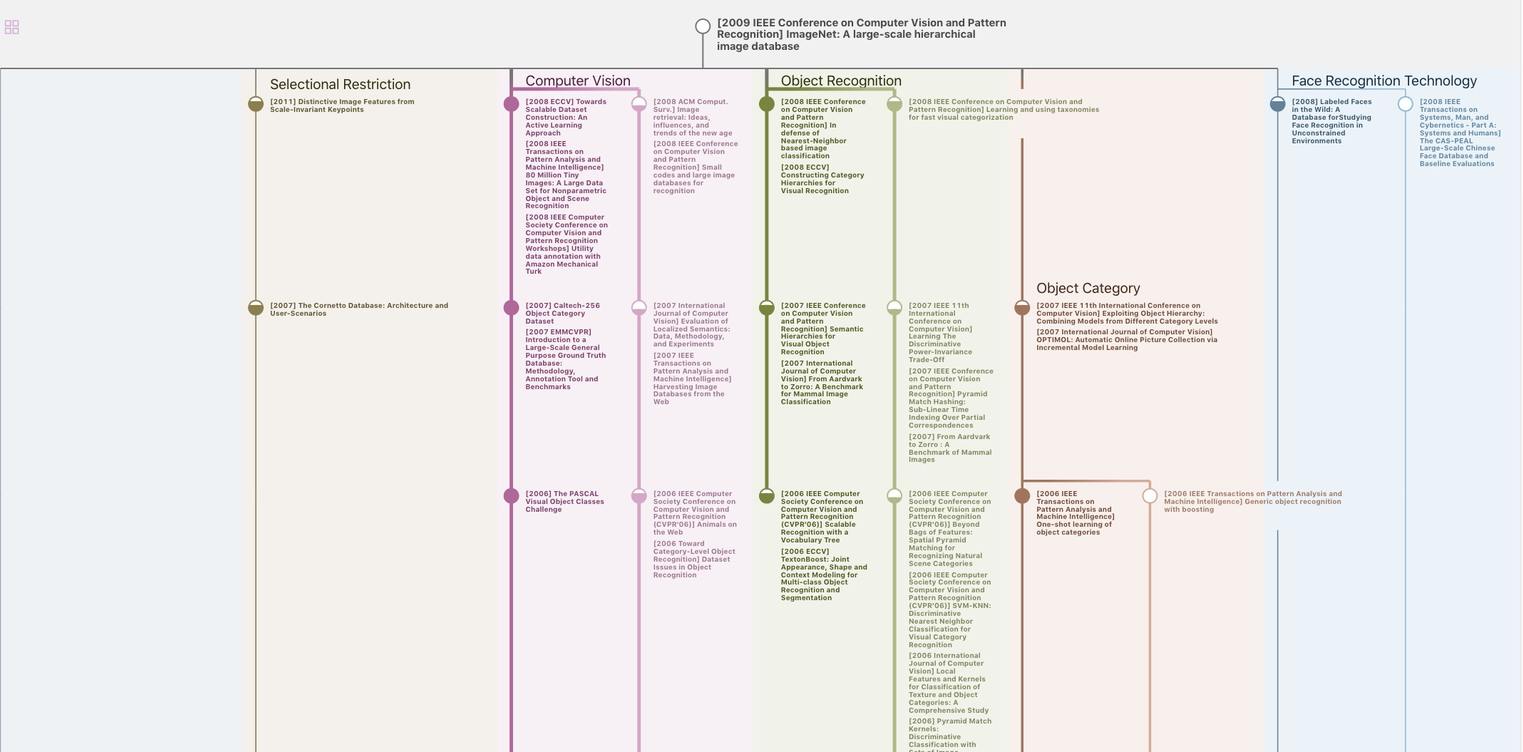
生成溯源树,研究论文发展脉络
Chat Paper
正在生成论文摘要