Global machine learning for spatial ontology population
Web Semantics: Science, Services and Agents on the World Wide Web(2015)
摘要
Understanding spatial language is important in many applications such as geographical information systems, human computer interaction or text-to-scene conversion. Due to the challenges of designing spatial ontologies, the extraction of spatial information from natural language still has to be placed in a well-defined framework. In this work, we propose an ontology which bridges between cognitive-linguistic spatial concepts in natural language and multiple qualitative spatial representation and reasoning models. To make a mapping between natural language and the spatial ontology, we propose a novel global machine learning framework for ontology population. In this framework we consider relational features and background knowledge which originate from both ontological relationships between the concepts and the structure of the spatial language. The advantage of the proposed global learning model is the scalability of the inference, and the flexibility for automatically describing text with arbitrary semantic labels that form a structured ontological representation of its content. The machine learning framework is evaluated with SemEval-2012 and SemEval-2013 data from the spatial role labeling task.
更多查看译文
关键词
Spatial information extraction,Spatial role labeling,Text mining,Structured output learning,Ontology population,Natural language processing
AI 理解论文
溯源树
样例
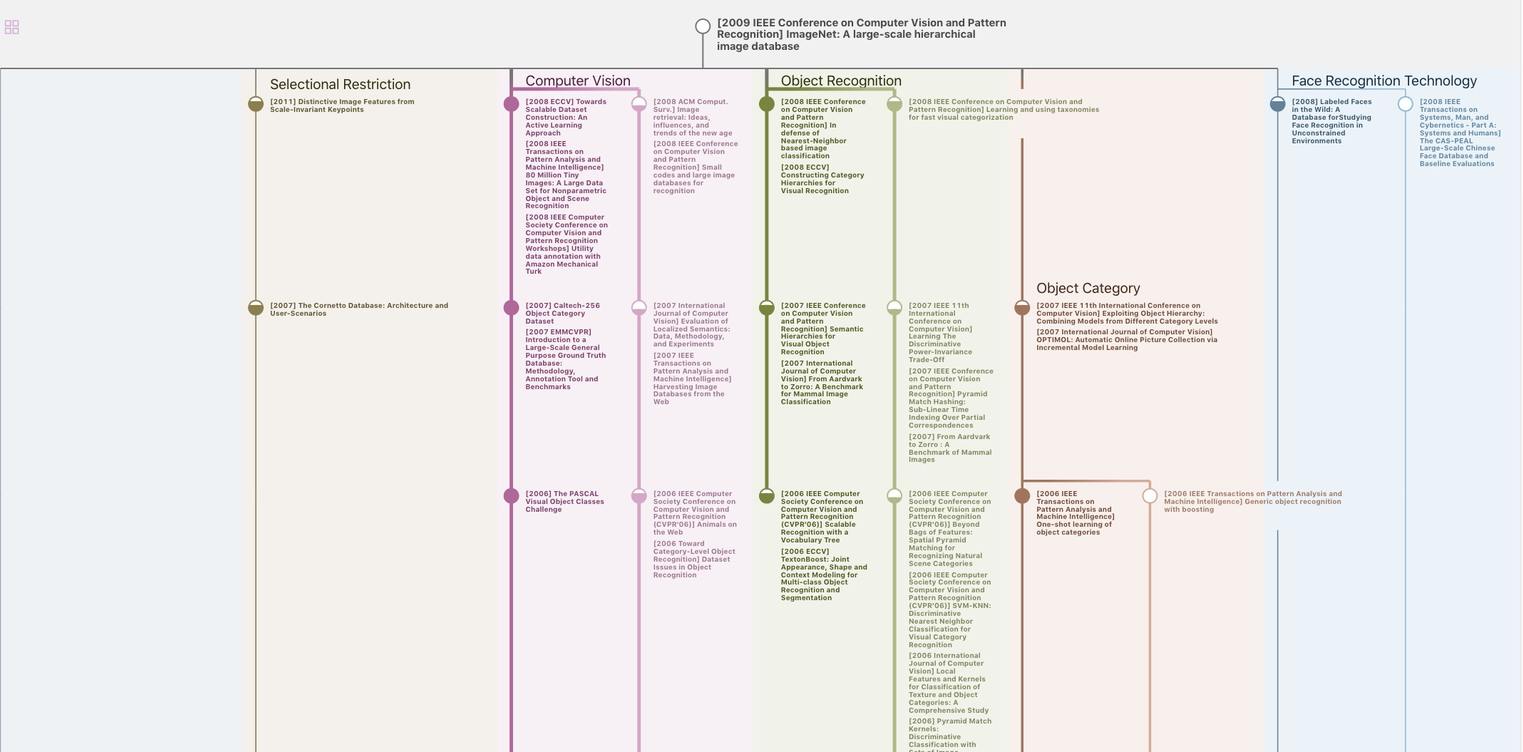
生成溯源树,研究论文发展脉络
Chat Paper
正在生成论文摘要