Object recognition using boosted discriminants
Computer Vision and Pattern Recognition, 2001. CVPR 2001. Proceedings of the 2001 IEEE Computer Society Conference(2001)
摘要
We approach the task of object discrimination as that of learning efficient "codes" for each object class in terms of responses to a set of chosen discriminants. We formulate this approach in an energy minimization framework. The "code" is built incrementally by successively constructing discriminants that focus on pairs of training images of ob- jects that are currently hard to classify. The particular dis- criminants that we use partition the set of objects of interest into two well-separated groups. We find the optimal dis- criminant as well as partition by formulating an objective criteria that measures the well-separateness of the parti- tion. We derive an iterative solution that alternates between the solutions for two generalized eigenproblems, one for the discriminant parameters and the other for the indica- tor variables denoting the partition. We show how the op- timization can easily be biased to focus on hard to classify pairs, which enables us to choose new discriminants one by one in a sequential manner. We validate our approach on a challenging face discrimination task using parts as features and show that it compares favorably with the performance of an eigenspace method.
更多查看译文
关键词
face recognition,learning (artificial intelligence),object recognition,discriminants,eigenproblems,energy minimization,face discrimination,learning,object class,object discrimination,object recognition,training images
AI 理解论文
溯源树
样例
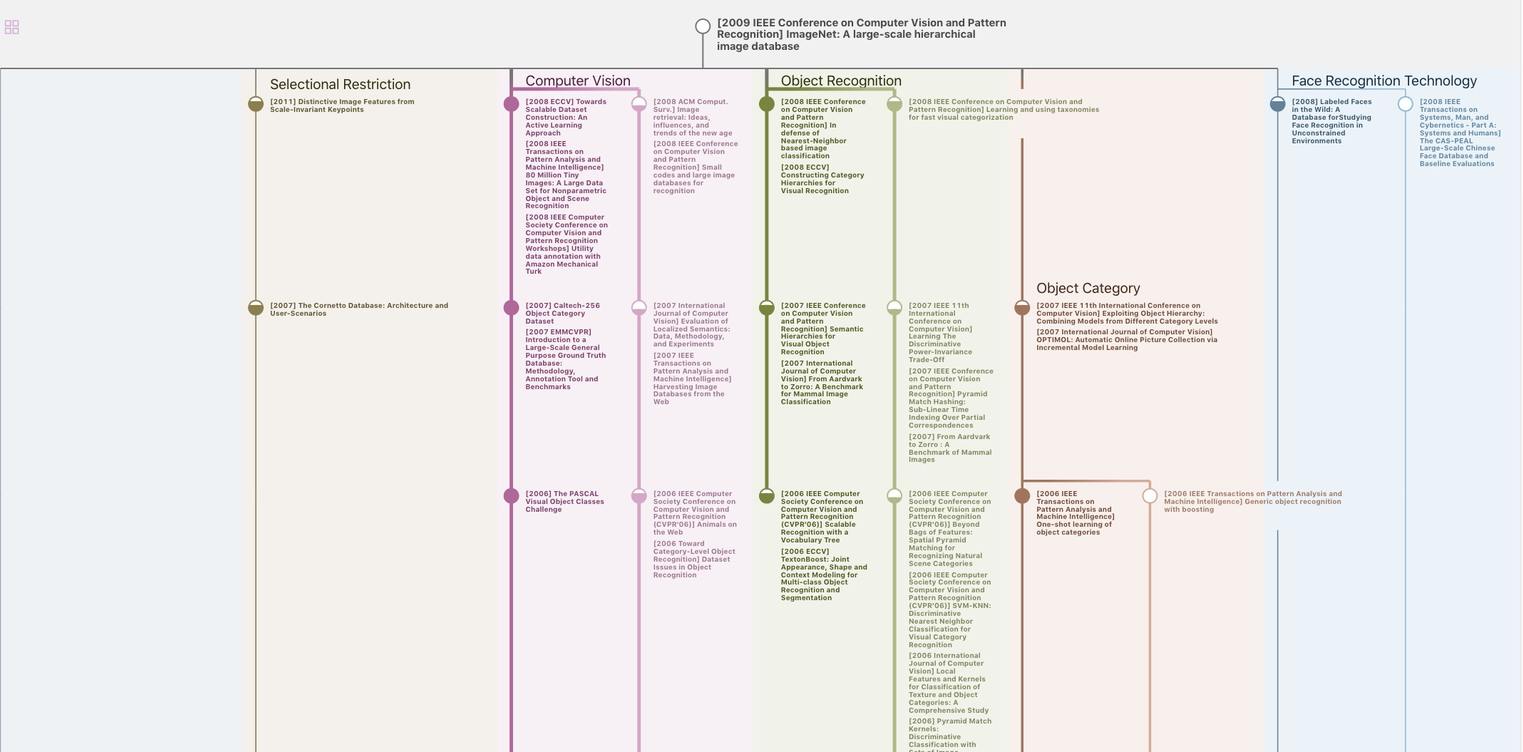
生成溯源树,研究论文发展脉络
Chat Paper
正在生成论文摘要