Distributed Balanced Clustering Via Mapping Coresets
ADVANCES IN NEURAL INFORMATION PROCESSING SYSTEMS 27 (NIPS 2014)(2014)
摘要
Large-scale clustering of data points in metric spaces is an important problem in mining big data sets. For many applications, we face explicit or implicit size constraints for each cluster which leads to the problem of clustering under capacity constraints or the "balanced clustering" problem. Although the balanced clustering problem has been widely studied, developing a theoretically sound distributed algorithm remains an open problem. In this paper we develop a new framework based on "mapping coresets" to tackle this issue. Our technique results in first distributed approximation algorithms for balanced clustering problems for a wide range of clustering objective functions such as k-center, k-median, and k-means.
更多查看译文
AI 理解论文
溯源树
样例
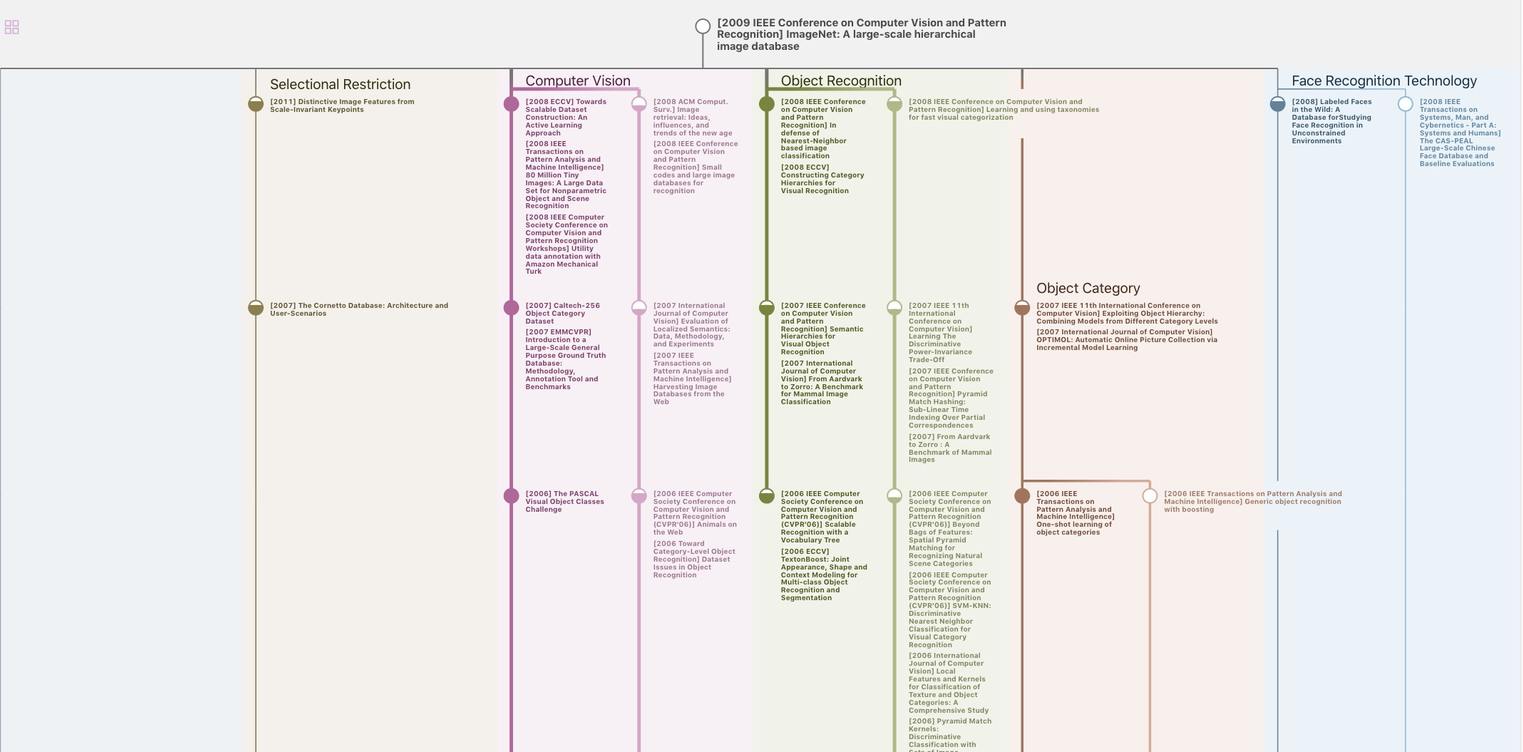
生成溯源树,研究论文发展脉络
Chat Paper
正在生成论文摘要