Learning visual co-occurrence with auto-encoder for image super-resolution
APSIPA(2014)
摘要
This paper proposes a novel neural network learning the essential mapping function between the low resolution and high resolution image for Image superresolution problem. In our approach, patch recurrence property of small patches in natural image are utilized as a prior to train the network. An autoencoder neutral network is designed to reconstruct the high resolution patches. The constraint that the output of the coding part should be similar as the corresponding high resolution patches is imposed to ameliorate the illness nature of the superresolution problem. In fact, the degeneration mapping from the high resolution image to the low resolution image is also integrated in the network. Both visual improvements and objective assessments are demonstrated on true images.
更多查看译文
关键词
visual cooccurrence learning,image coding,learning (artificial intelligence),autoencoder neutral network learning,patch recurrence property,image resolution,low image resolution,high image resolution,degeneration mapping,image superresolution problem,mapping function,neural nets,computer vision,psnr
AI 理解论文
溯源树
样例
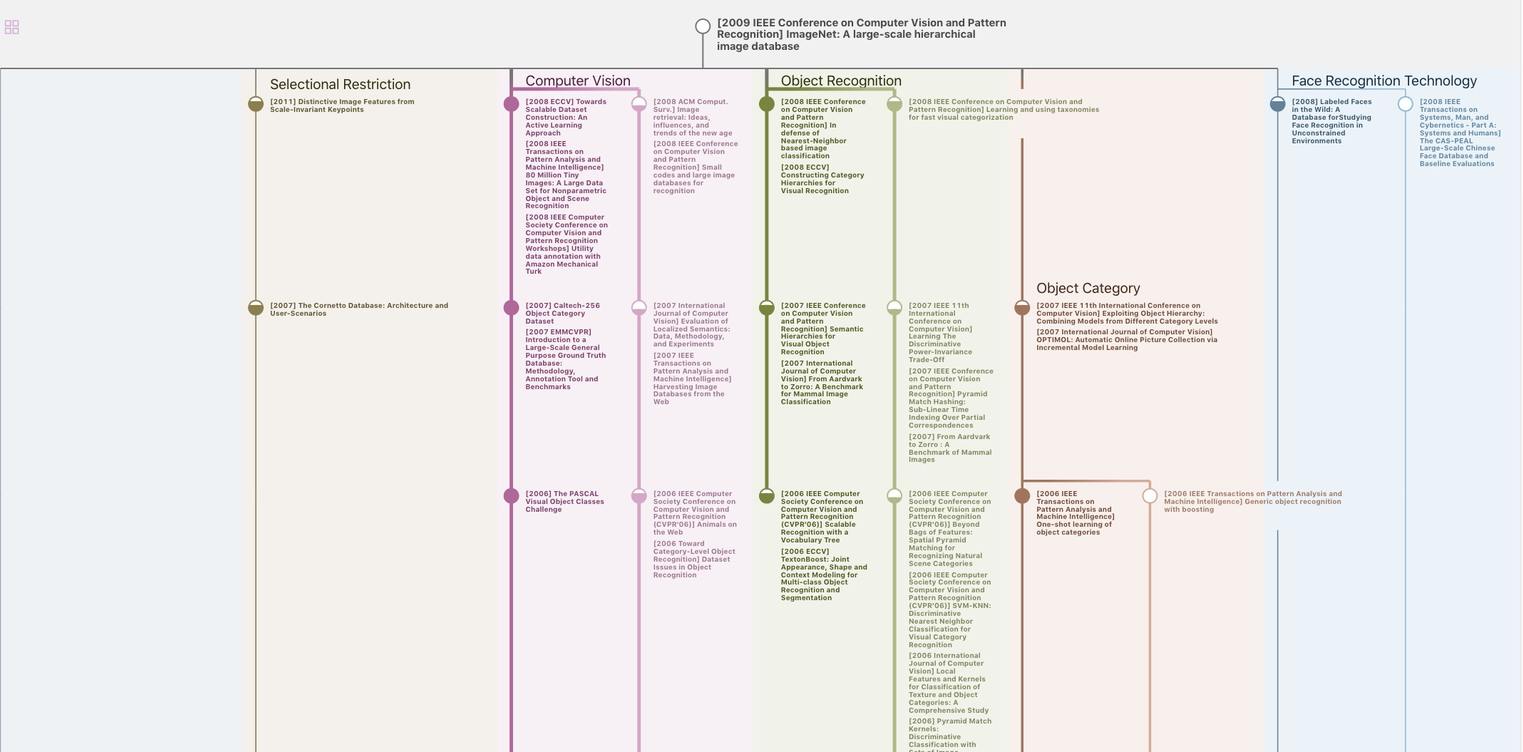
生成溯源树,研究论文发展脉络
Chat Paper
正在生成论文摘要