Granularity of Co-evolution Patterns in Dynamic Attributed Graphs.
ADVANCES IN INTELLIGENT DATA ANALYSIS XIII(2014)
摘要
Many applications see huge demands for discovering relevant patterns in dynamic attributed graphs, for instance in the context of social interaction analysis. It is often possible to associate a hierarchy on the attributes related to graph vertices to explicit prior knowledge. For example, considering the study of scientific collaboration networks, conference venues and journals can be grouped with respect to types or topics. We propose to extend a recent constraint-based mining method by exploiting such hierarchies on attributes. We define an algorithm that enumerates all multi-level co-evolution sub-graphs, i.e., induced sub-graphs that satisfy a topologic constraint and whose vertices follow the same evolution on a set of attributes during some timestamps. Experiments show that hierarchies make it possible to return more concise collections of patterns without information loss in a feasible time.
更多查看译文
关键词
dynamic attributed graphs,patterns,co-evolution
AI 理解论文
溯源树
样例
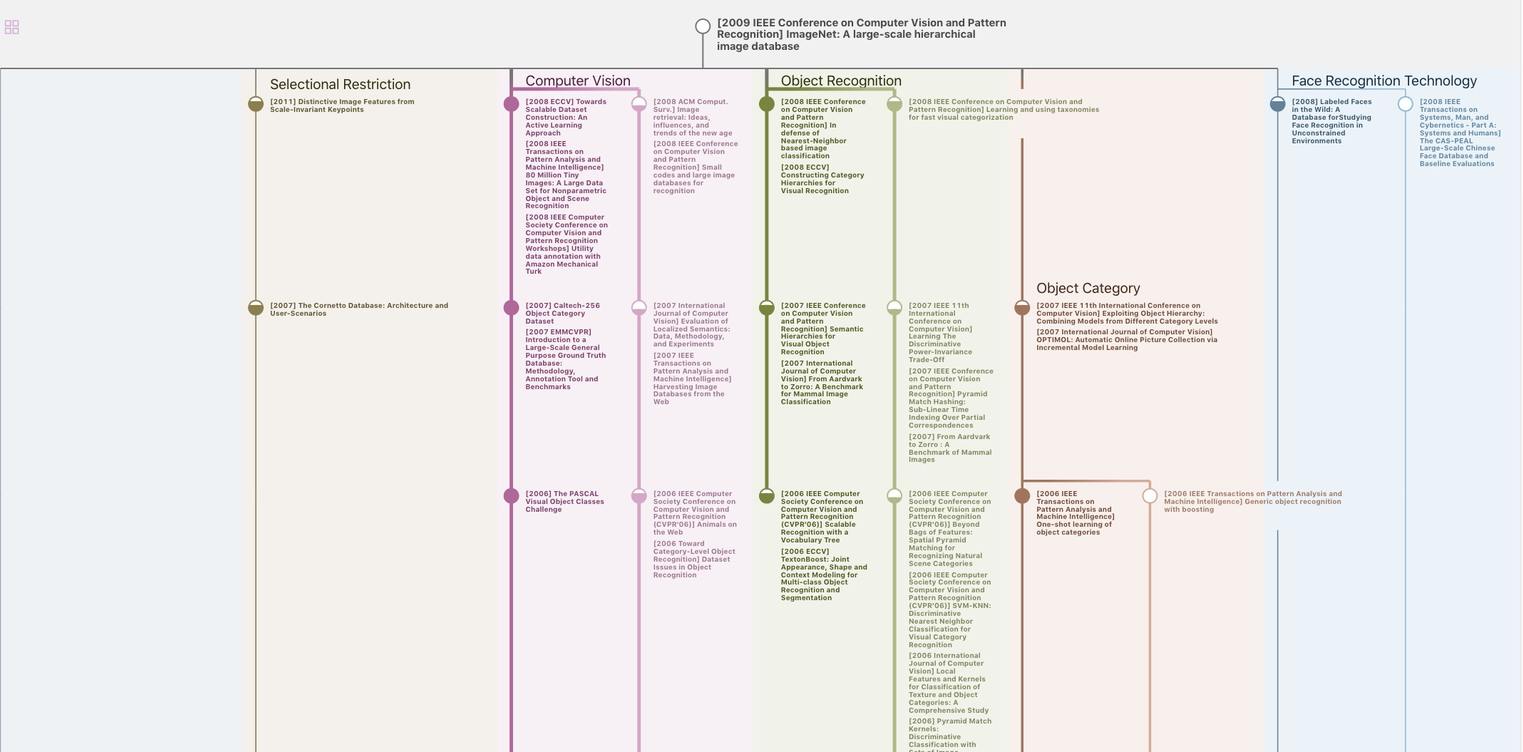
生成溯源树,研究论文发展脉络
Chat Paper
正在生成论文摘要