Probability-one homotopy maps for tracking constrained clustering solutions
SpringSim (HPC)(2013)
摘要
Modern machine learning problems typically have multiple criteria, but there is currently no systematic mathematical theory to guide the design of formulations and exploration of alternatives. Homotopy methods are a promising approach to characterize solution spaces by smoothly tracking solutions from one formulation (typically an "easy" problem) to another (typically a "hard" problem). New results in constructing homotopy maps for constrained clustering problems are here presented, which combine quadratic loss functions with discrete evaluations of constraint violations are presented. These maps balance requirements of locality in clusters as well as those of discrete must-link and must-not-link constraints. Experimental results demonstrate advantages in tracking solutions compared to state-of-the-art constrained clustering algorithms.
更多查看译文
关键词
homotopy method,discrete evaluation,maps balance requirement,probability-one homotopy map,multiple criterion,must-not-link constraint,homotopy map,constraint violation,modern machine,discrete must-link,constrained clustering,machine learning
AI 理解论文
溯源树
样例
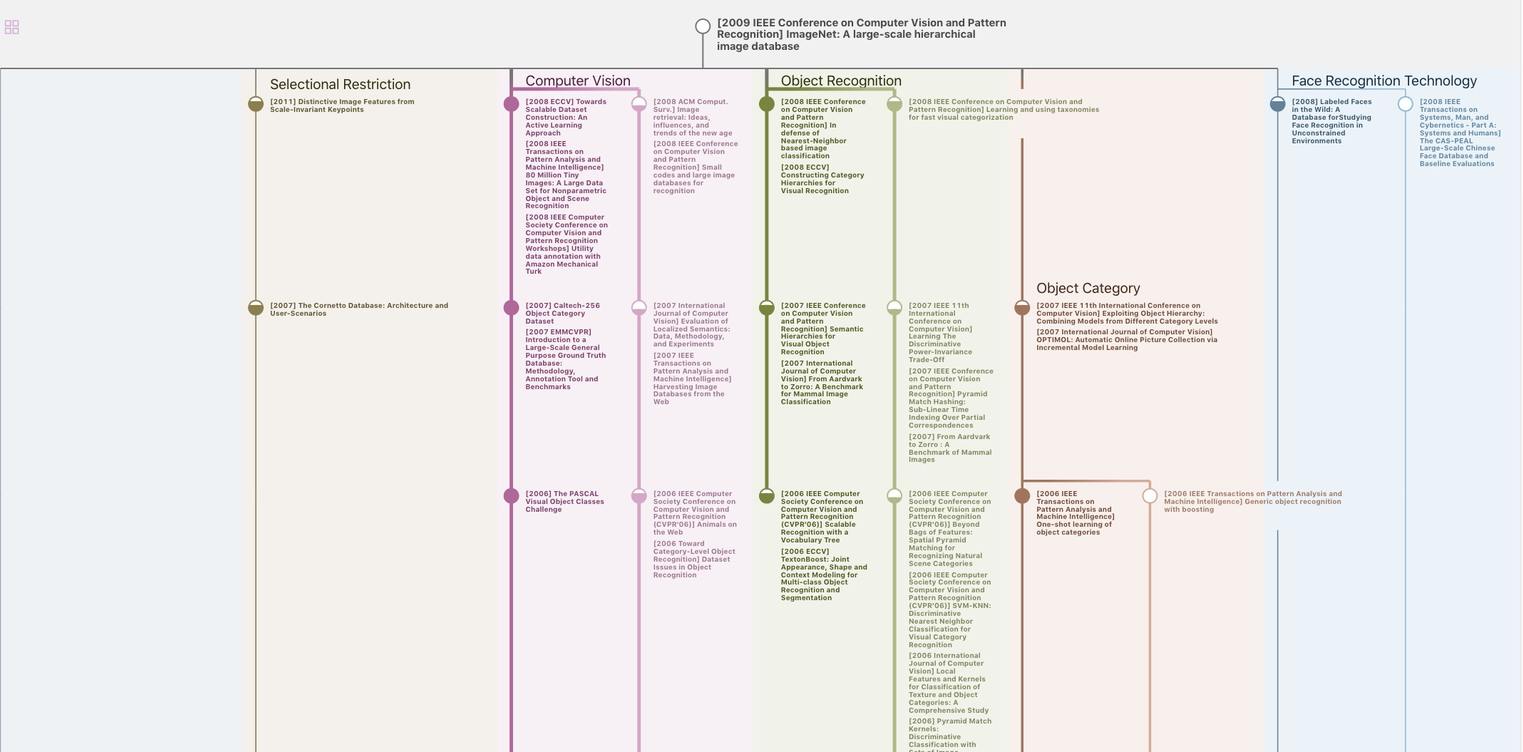
生成溯源树,研究论文发展脉络
Chat Paper
正在生成论文摘要