Selecting and Weighting N-Grams to Identify 1100 Languages.
Lecture Notes in Computer Science(2013)
摘要
This paper presents a language identification algorithm using cosine similarity against a filtered and weighted subset of the most frequent n-grams in training data with optional inter-string score smoothing, and its implementation in an open-source program. When applied to a collection of strings in 1100 languages containing at most 65 characters each, an average classification accuracy of over 99.2% is achieved with smoothing and 98.2% without. Compared to three other open-source language identification programs, the new program is both much more accurate and much faster at classifying short strings given such a large collection of languages.
更多查看译文
关键词
language identification,discriminative training,n-grams
AI 理解论文
溯源树
样例
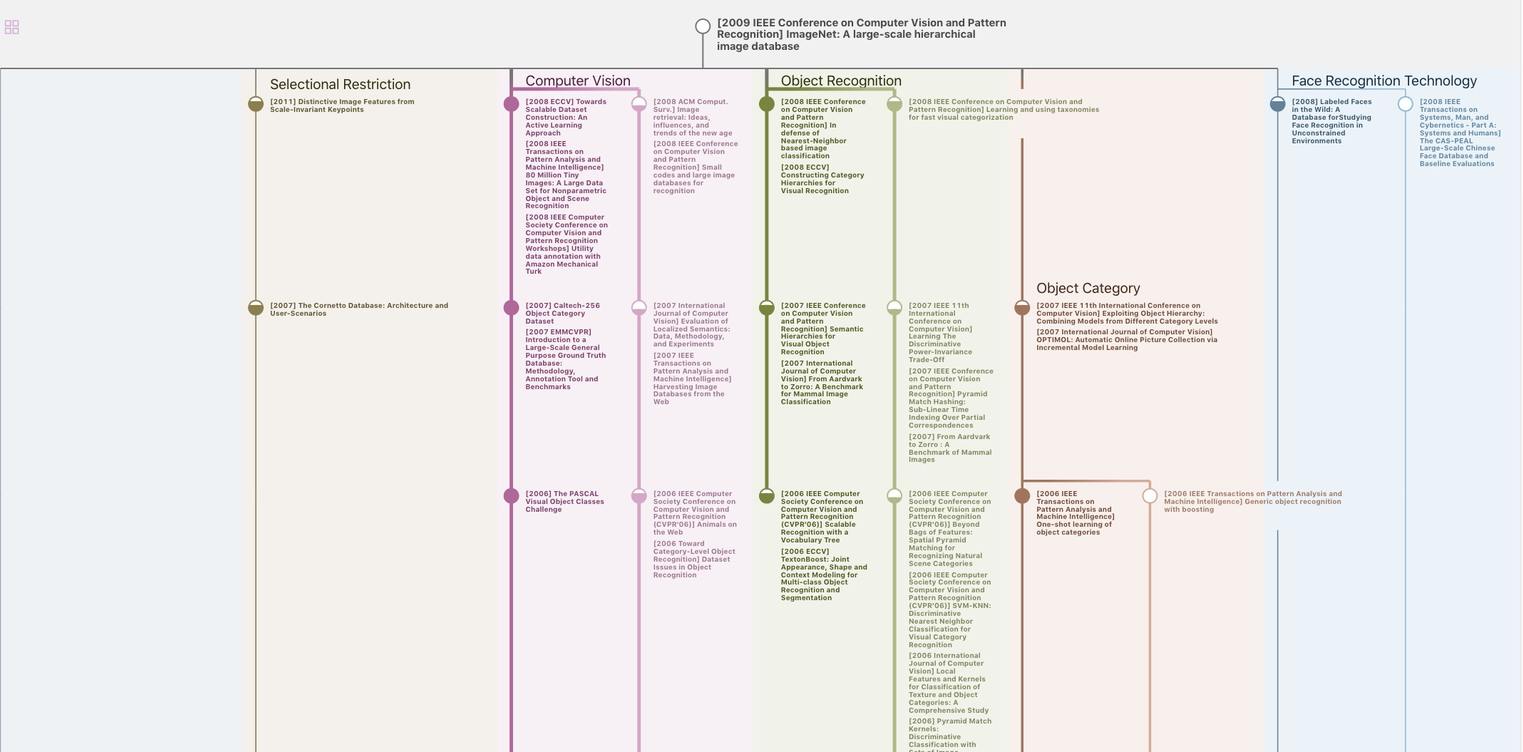
生成溯源树,研究论文发展脉络
Chat Paper
正在生成论文摘要