Combining Unsupervised and Supervised Machine Learning to Build User Models for Intelligent Learning Environments
msra(2007)
摘要
Traditional approaches to developing user models, especially for computer-based learning environments, are notoriously difficult and time-consuming because they rely heavily on expert-elicited knowledge about the target application and domain. Furthermore, because the necessary expert knowledge is application and domain specific, the entire model development process must be repeated for each new application. In this paper, we present a data-based user modeling framework that uses both unsupervised and supervised machine learning to reduce the costs of building student models, and facilitate transferability. We apply the framework to build student models for two different learning environments and using two different data sources (logged interface and eye-tracking data). Despite limitations due to the size of our datasets, we provide initial evidence that the framework can automatically identify meaningful student interaction behaviors and can be used to build user models for the online classification of new student behaviors online. We also show framework transferability across applications and data types.
更多查看译文
关键词
data mining,user modeling,intelligent learning environments this paper or a similar version is not currently under review by a journal or conference,unsupervised and supervised learning,nor will it be submitted to such within the next three months.,user model,eye tracking,data type,supervised learning
AI 理解论文
溯源树
样例
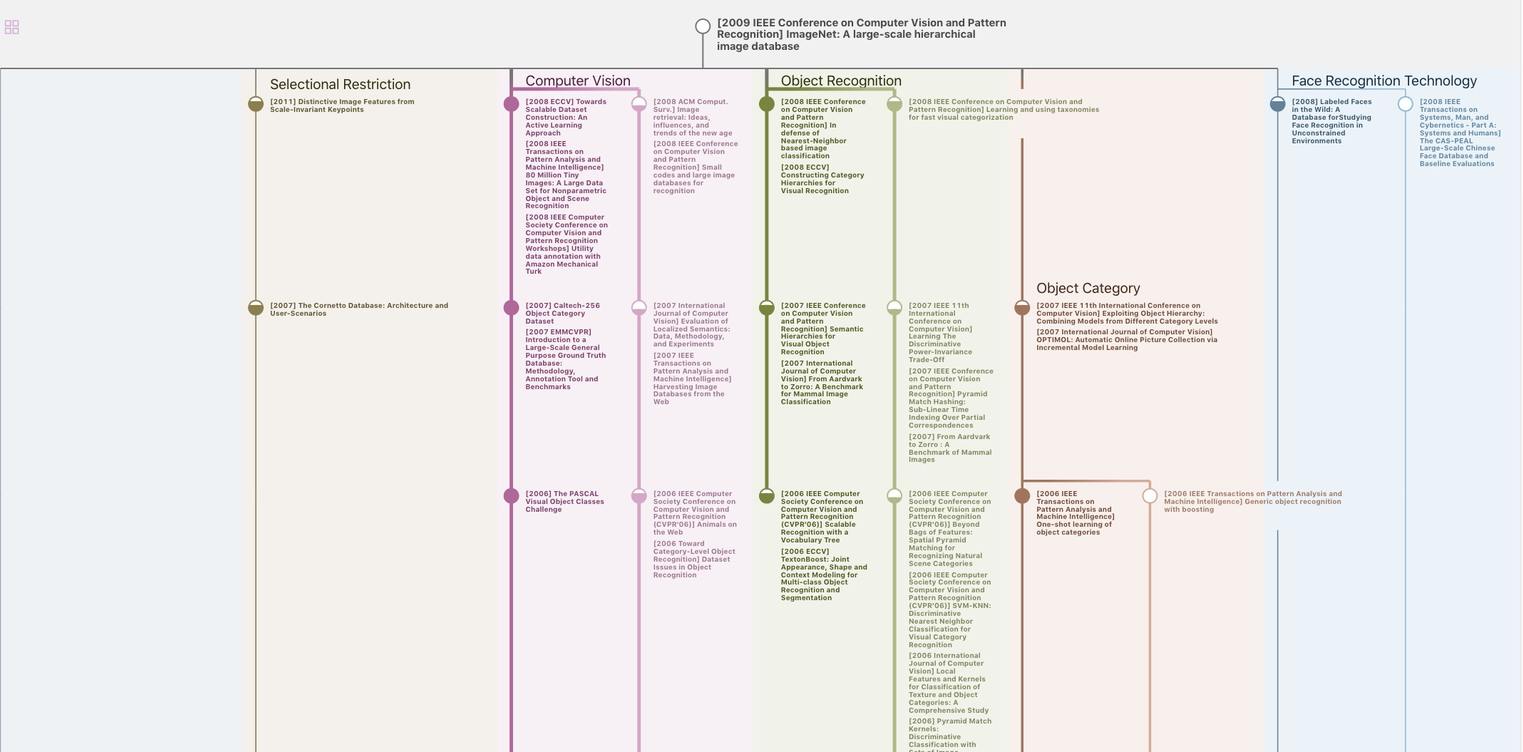
生成溯源树,研究论文发展脉络
Chat Paper
正在生成论文摘要