The Identity Management Kalman Filter (IMKF)
Robotics: Science and Systems(2006)
摘要
Tracking posterior estimates for problems with data association uncertainty is one of the big open problems in the literature on filtering and tracking. This paper presents a new filter for the on-line tracking of many individual objects with data association ambiguities. It tightly integrates the continuous aspects of the problem (locating the objects) with the discrete aspects (the data association ambiguities). The key innovation is a probabilistic information matrix that efficiently encodes identity information by linking world entities with internal tracks of the filter, enabling it to maintain a full posterior over the system amid data association uncertainties. The filter scales quadratically in complexity, just like a conventional Kalman filter, avoiding the exponential explosion of the association space. We derive the algorithm formally and present large-scale simulation results. I. INTRODUCTION
更多查看译文
关键词
identity management,information matrix,kalman filter
AI 理解论文
溯源树
样例
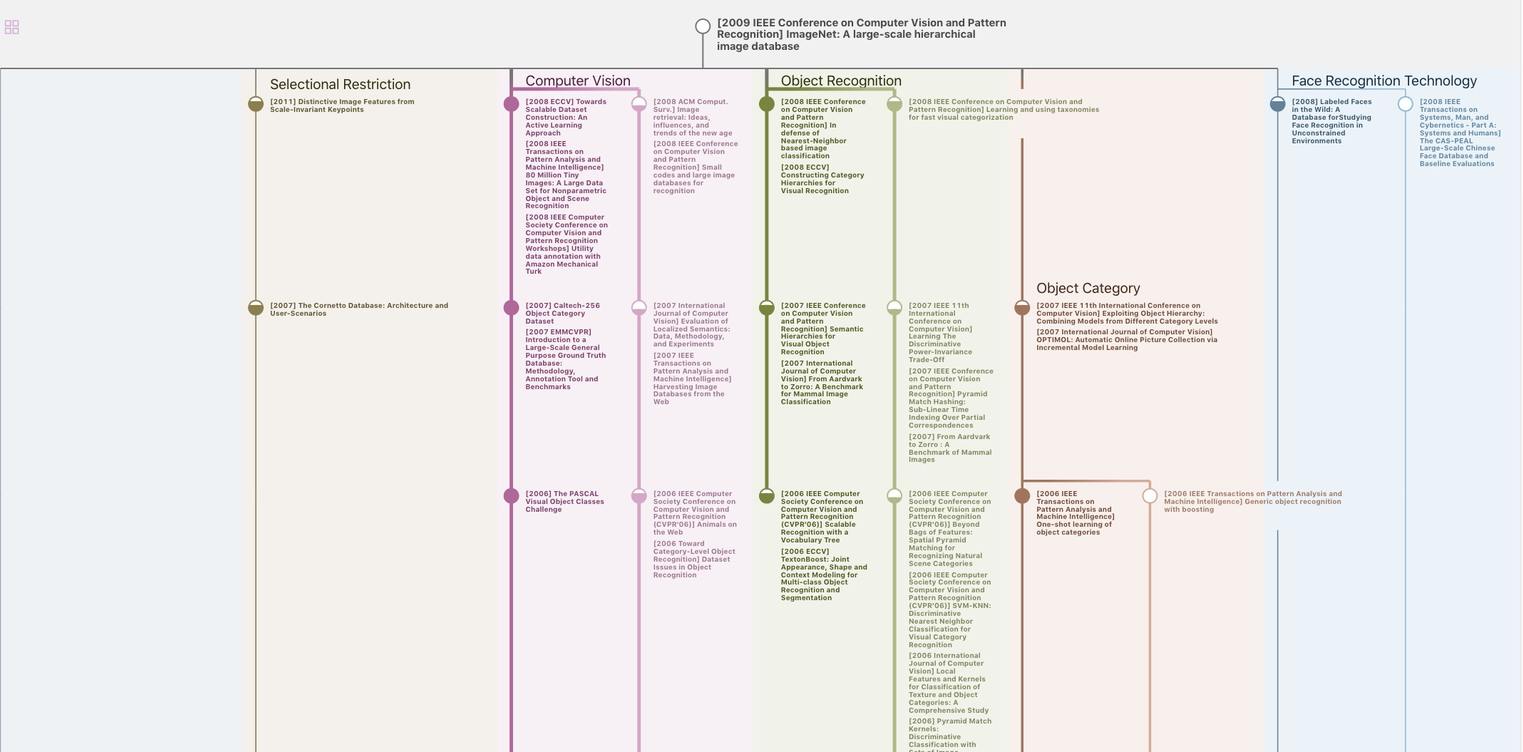
生成溯源树,研究论文发展脉络
Chat Paper
正在生成论文摘要