Learning to Measure Influence in a Scientific Social Network.
ADVANCES IN ARTIFICIAL INTELLIGENCE, CANADIAN AI 2014(2014)
摘要
In research, influence is often synonymous with importance; the researcher that is judged to be influential is often chosen for the grants, distinctions and promotions that serve as fuel for research programs. The influence of a researcher is often measured by how often he or she is cited, yet as a measure of influence, we show that citation frequency is only weakly correlated with influence ratings collected from peers. In this paper, we use machine learning to enable a new system that provides a better measure of researcher influence. This system predicts the influence of one researcher on another via a range of novel social, linguistic, psychological, and bibliometric features. To collect data for training and testing this approach, we conducted a survey of 74 researchers in the field of computational linguistics, and collected thousands of influence ratings. Our results on this data show that our approach significantly outperforms measures based on citations alone, improving prediction accuracy by 56%. We also perform a detailed analysis of the key features in our model, and make some important observations about the scientific and non-scientific factors that most predict researcher influence.
更多查看译文
AI 理解论文
溯源树
样例
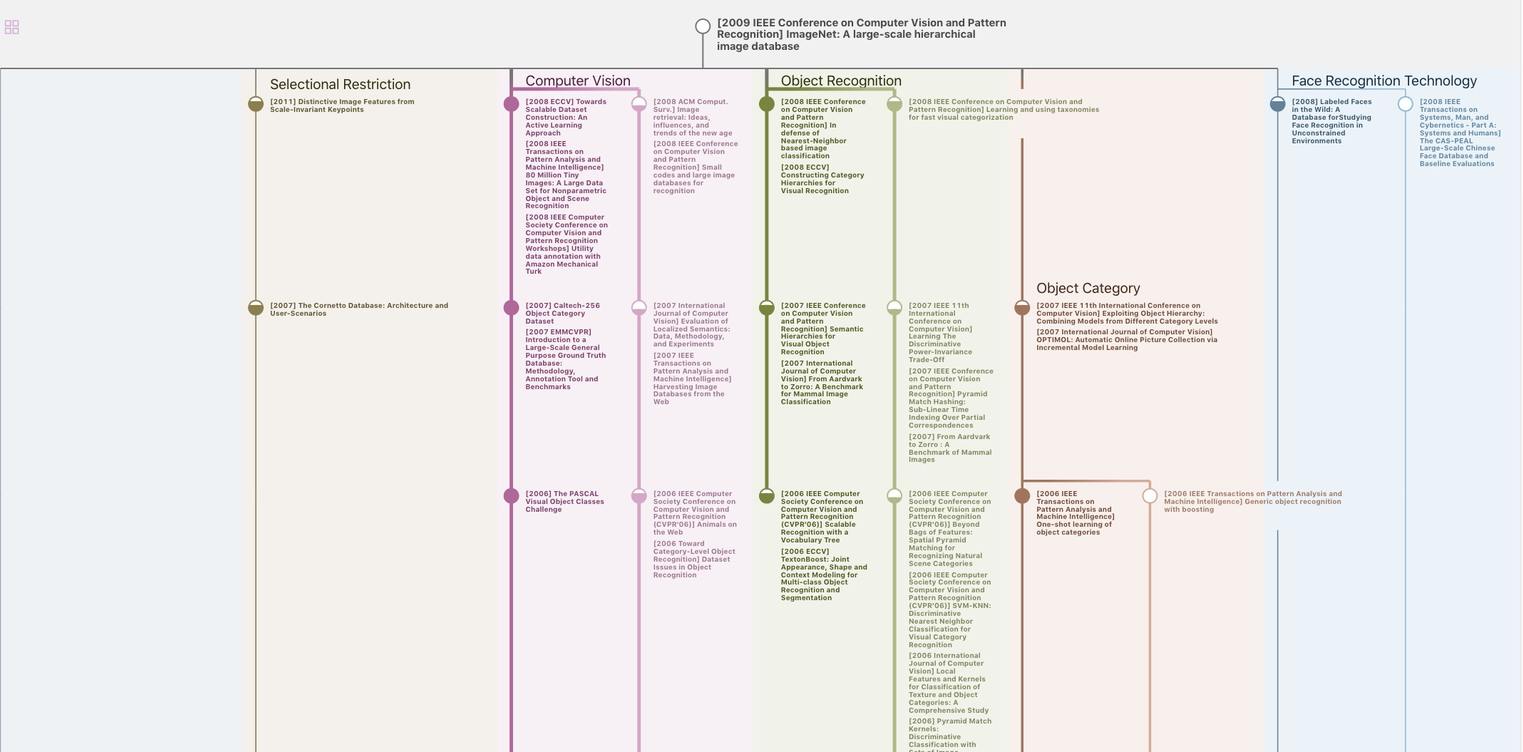
生成溯源树,研究论文发展脉络
Chat Paper
正在生成论文摘要