Query-dependent cross-domain ranking in heterogeneous network
Knowl. Inf. Syst.(2012)
摘要
Traditional learning-to-rank problem mainly focuses on one single type of objects. However, with the rapid growth of the Web 2.0, ranking over multiple interrelated and heterogeneous objects becomes a common situation, e.g., the heterogeneous academic network. In this scenario, one may have much training data for some type of objects (e.g. conferences) while only very few for the interested types of objects (e.g. authors). Thus, the two important questions are: (1) Given a networked data set, how could one borrow supervision from other types of objects in order to build an accurate ranking model for the interested objects with insufficient supervision? (2) If there are links between different objects, how can we exploit their relationships for improved ranking performance? In this work, we first propose a regularized framework called HCDRank to simultaneously minimize two loss functions related to these two domains. Then, we extend the approach by exploiting the link information between heterogeneous objects. We conduct a theoretical analysis to the proposed approach and derive its generalization bound to demonstrate how the two related domains could help each other in learning ranking functions. Experimental results on three different genres of data sets demonstrate the effectiveness of the proposed approaches.
更多查看译文
关键词
cross-domain ranking,heterogeneous network,latent space,learning to rank
AI 理解论文
溯源树
样例
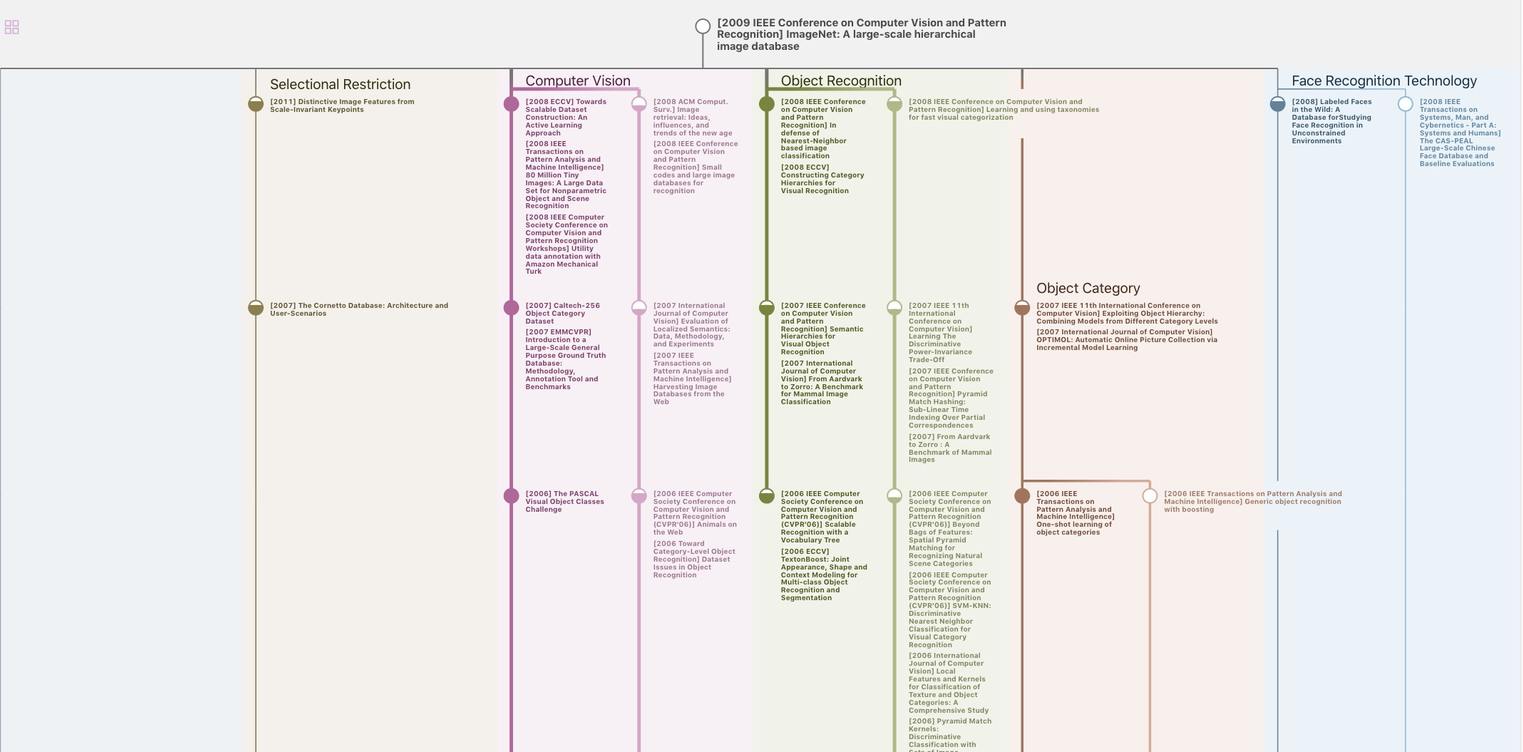
生成溯源树,研究论文发展脉络
Chat Paper
正在生成论文摘要