CHOMP: Covariant Hamiltonian optimization for motion planning
I. J. Robotic Res.(2013)
摘要
In this paper, we present CHOMP (covariant Hamiltonian optimization for motion planning), a method for trajectory optimization invariant to reparametrization. CHOMP uses functional gradient techniques to iteratively improve the quality of an initial trajectory, optimizing a functional that trades off between a smoothness and an obstacle avoidance component. CHOMP can be used to locally optimize feasible trajectories, as well as to solve motion planning queries, converging to low-cost trajectories even when initialized with infeasible ones. It uses Hamiltonian Monte Carlo to alleviate the problem of convergence to high-cost local minima (and for probabilistic completeness), and is capable of respecting hard constraints along the trajectory. We present extensive experiments with CHOMP on manipulation and locomotion tasks, using seven-degree-of-freedom manipulators and a rough-terrain quadruped robot.
更多查看译文
关键词
Motion planning,constrained optimization,distance fields
AI 理解论文
溯源树
样例
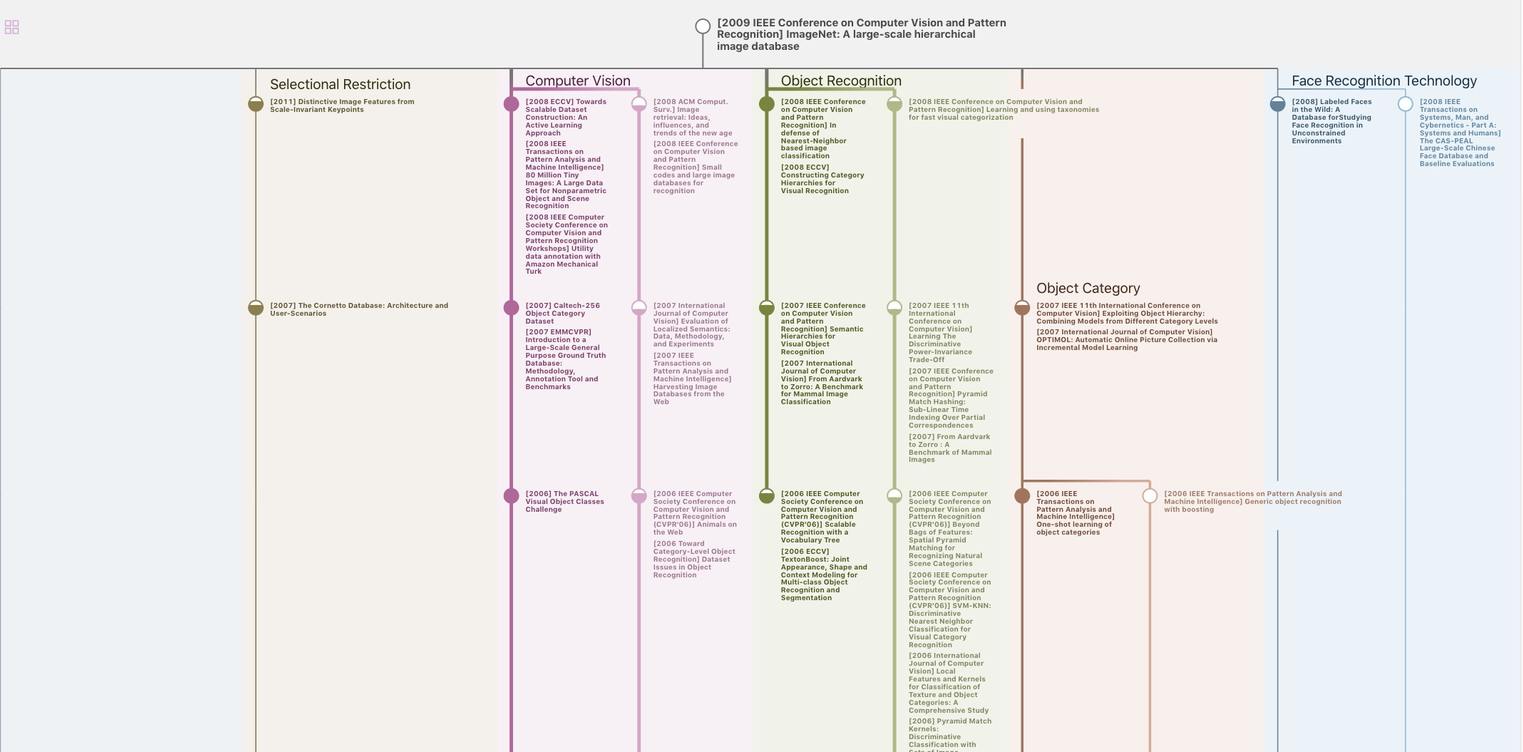
生成溯源树,研究论文发展脉络
Chat Paper
正在生成论文摘要