Machine learning approaches for network-based prediction of disease outcomes and protein functions
Machine learning approaches for network-based prediction of disease outcomes and protein functions(2012)
摘要
In this dissertation, we address two major problems in bioinformatics: disease outcome prediction and protein function prediction. To solve the issues, we develop network-based machine learning frameworks. For predicting sample disease status, the statistical and computational challenge to construct such a predictor is that thousands of genes can be used to predict for the disease categories, but only a small number of samples are available. We propose a gene network modular-based linear discriminant analysis approach by integrating `essential' correlation structure among genes into the predictor in order that the modules or cluster structures of genes, which are related to the diagnostic classes we look for, can have potential biological interpretation. For predicting protein functions, we devise a relaxation labeling procedure to find its maximally likely labeling. We also address the problem of multi-label classification of protein functions by taking the relationship of gene ontology terms into account. Our algorithms have significantly advanced the state-of-the-art computational methods for functional characterization of proteins using the integrated function association network. For both machine learning frameworks, we present detailed empirical studies of our algorithms and compare them with state-of-art techniques on gene and protein networks. We show significant performance gains in comparison with other state-of-the-art techniques.
更多查看译文
关键词
network-based prediction,integrated function association network,sample disease status,protein function prediction,protein function,gene ontology term,gene network,protein network,disease outcome prediction,computational challenge,disease category
AI 理解论文
溯源树
样例
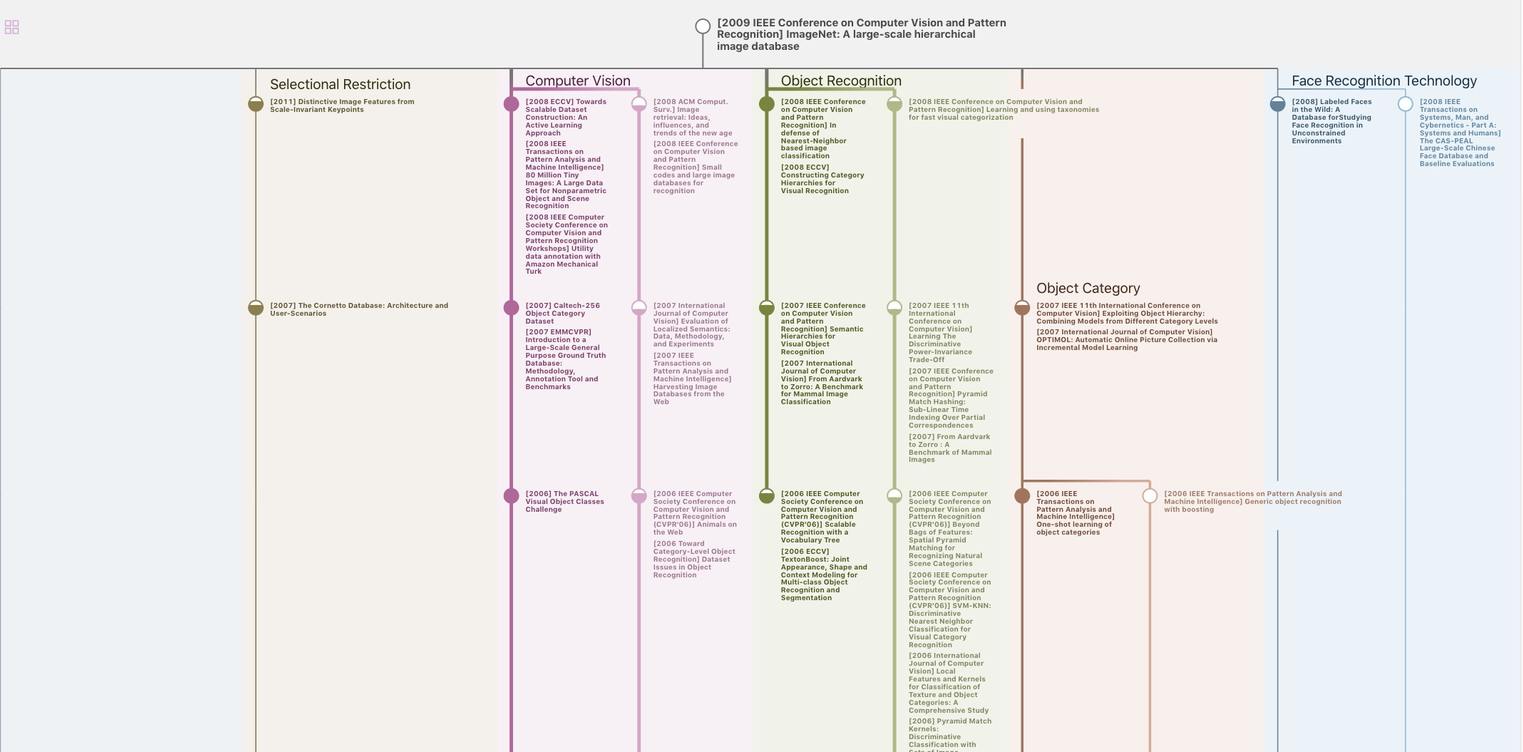
生成溯源树,研究论文发展脉络
Chat Paper
正在生成论文摘要