Variable selection for Fisher linear discriminant analysis using the modified sequential backward selection algorithm for the microarray data.
Applied Mathematics and Computation(2014)
摘要
One of the major challenges is small sample size as compared to large features number for microarray data. Variable selection is an important step for improving diagnostics of cancer or the classification according to the phenotypes via gene expression data. In this study, we propose a modified sequential backward selection (SBS) algorithm to deal with the case where the covariance matrix is singular. Then we propose a variable selection algorithm based on the weighted Mahalanobis distance and modified SBS methods. Furthermore, based on the proposed variable selection algorithm, a Fisher linear discriminant method is proposed to improve the accuracy of tumor classification through simultaneously taking into account genes’ joint discriminatory power. To validate the efficiency, we apply the proposed discriminant method to two different DNA microarray data sets for experiment investigation. The empirical results show that our method for tumor classification can obtain better classification effectiveness than Markov random field method and independent variable group analysis I methods, which demonstrates that the proposed variable selection method can obtain more correct and informative gene subset if taking into account the joint discriminatory power of genes for tumor classification.
更多查看译文
关键词
Variable selection,Fisher linear discriminant analysis,Modified SBS,Weighted Mahalanobis distance,Microarray data
AI 理解论文
溯源树
样例
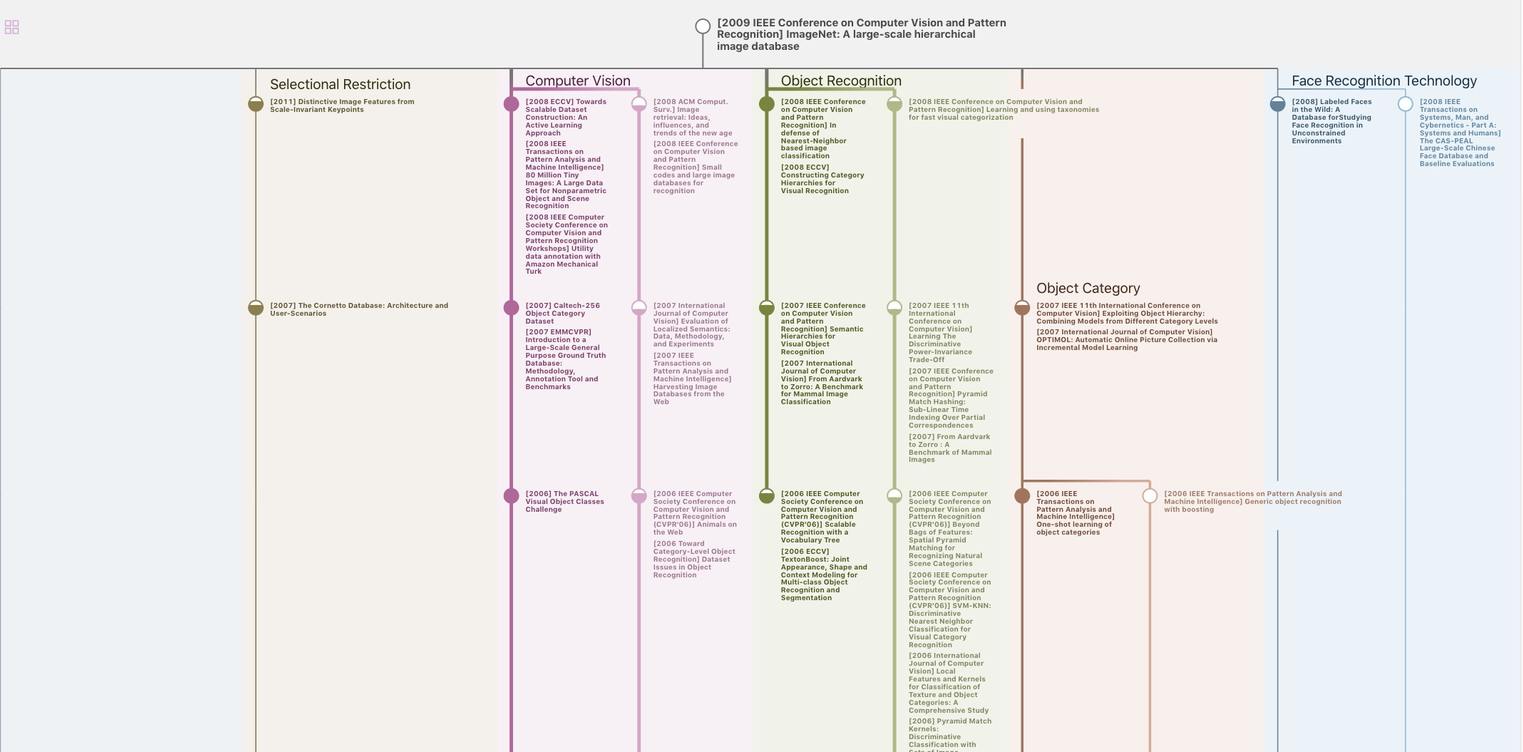
生成溯源树,研究论文发展脉络
Chat Paper
正在生成论文摘要