Rule-Based Anomaly Detection On Ip Flows
IEEE INFOCOM 2009 - IEEE CONFERENCE ON COMPUTER COMMUNICATIONS, VOLS 1-5(2009)
摘要
Rule-based packet classification is a powerful method for identifying traffic anomalies, with network security as a key application area. While popular systems like Snort are used in many network locations, comprehensive deployment across Tier-1 service provider networks is costly due to the need for high-speed monitors at many network ingress points. Since ISPs already collect flow statistics ubiquitously, can we use it for detecting the same anomalies as the packet based rules in spite of aggregation and absence of payload information? We exploit correlations between packet and flow level information via a machine learning (ML) approach to associate packet level alarms with a feature vector derived from flow records on the same traffic. We describe a system architecture for network-wide flow-alarming and describe the steps required to establish a proof-of-concept. We evaluate prediction accuracy of candidate ML algorithms on actual packet traces. The duration of prediction effectiveness is an issue for ML approaches and more so in resource intensive network applications. Initial results show little impairment of performance over periods of one or two weeks.
更多查看译文
关键词
feature vector,rule based,accuracy,computer worms,statistics,learning artificial intelligence,machine learning,protocols,system architecture,testing,anomaly detection,payloads,service provider,network security,proof of concept,intrusion detection,buffer overflow,power method,inspection
AI 理解论文
溯源树
样例
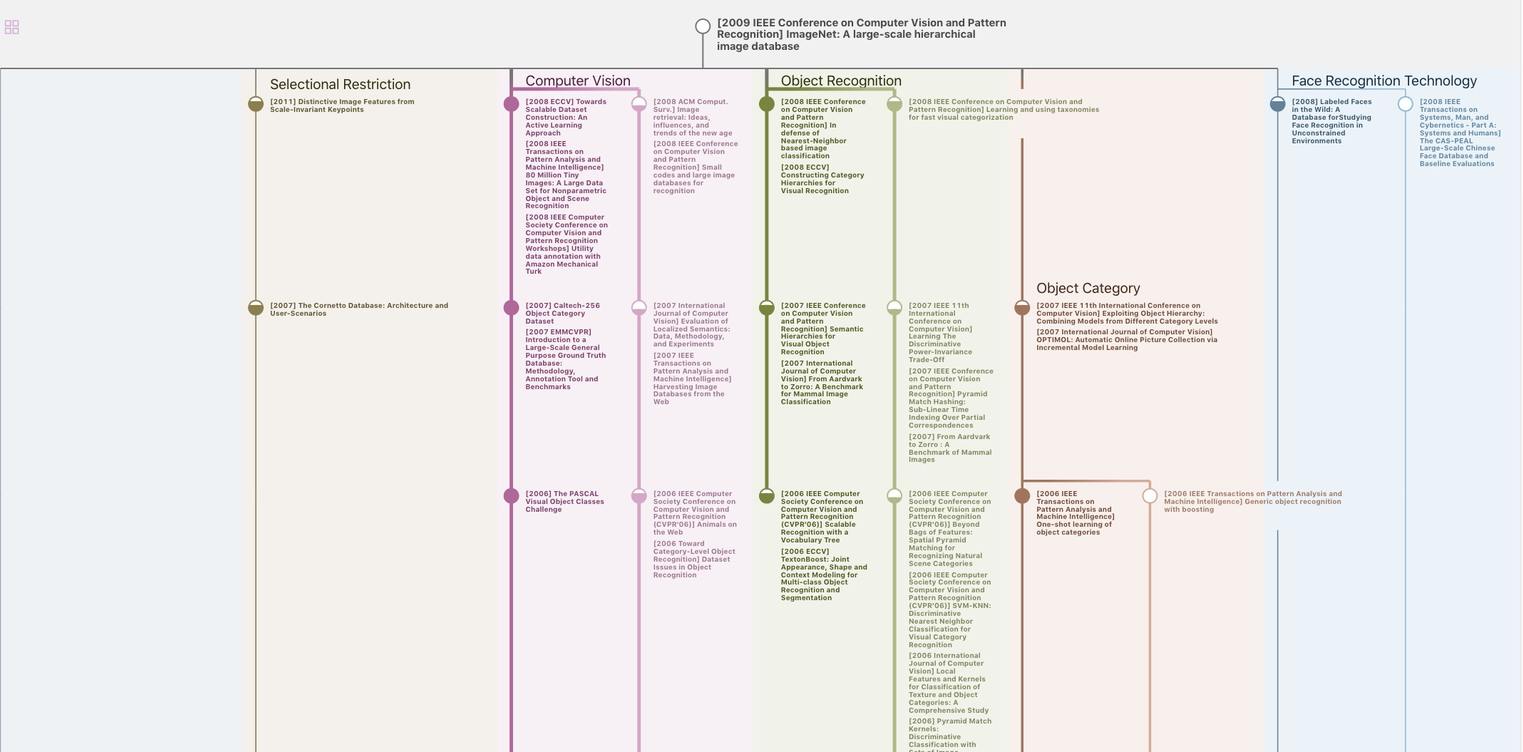
生成溯源树,研究论文发展脉络
Chat Paper
正在生成论文摘要