Label Ranking with Partial Abstention based on Thresholded Probabilistic Models.
NIPS(2012)
摘要
Several machine learning methods allow for abstaining from uncertain predictions. While being common for settings like conventional classification, abstention has been studied much less in learning to rank. We address abstention for the label ranking setting, allowing the learner to declare certain pairs of labels as being incomparable and, thus, to predict partial instead of total orders. In our method, such predictions are produced via thresholding the probabilities of pairwise preferences between labels, as induced by a predicted probability distribution on the set of all rankings. We formally analyze this approach for the Mallows and the Plackett-Luce model, showing that it produces proper partial orders as predictions and characterizing the expressiveness of the induced class of partial orders. These theoretical results are complemented by experiments demonstrating the practical usefulness of the approach.
更多查看译文
关键词
label,partial abstention
AI 理解论文
溯源树
样例
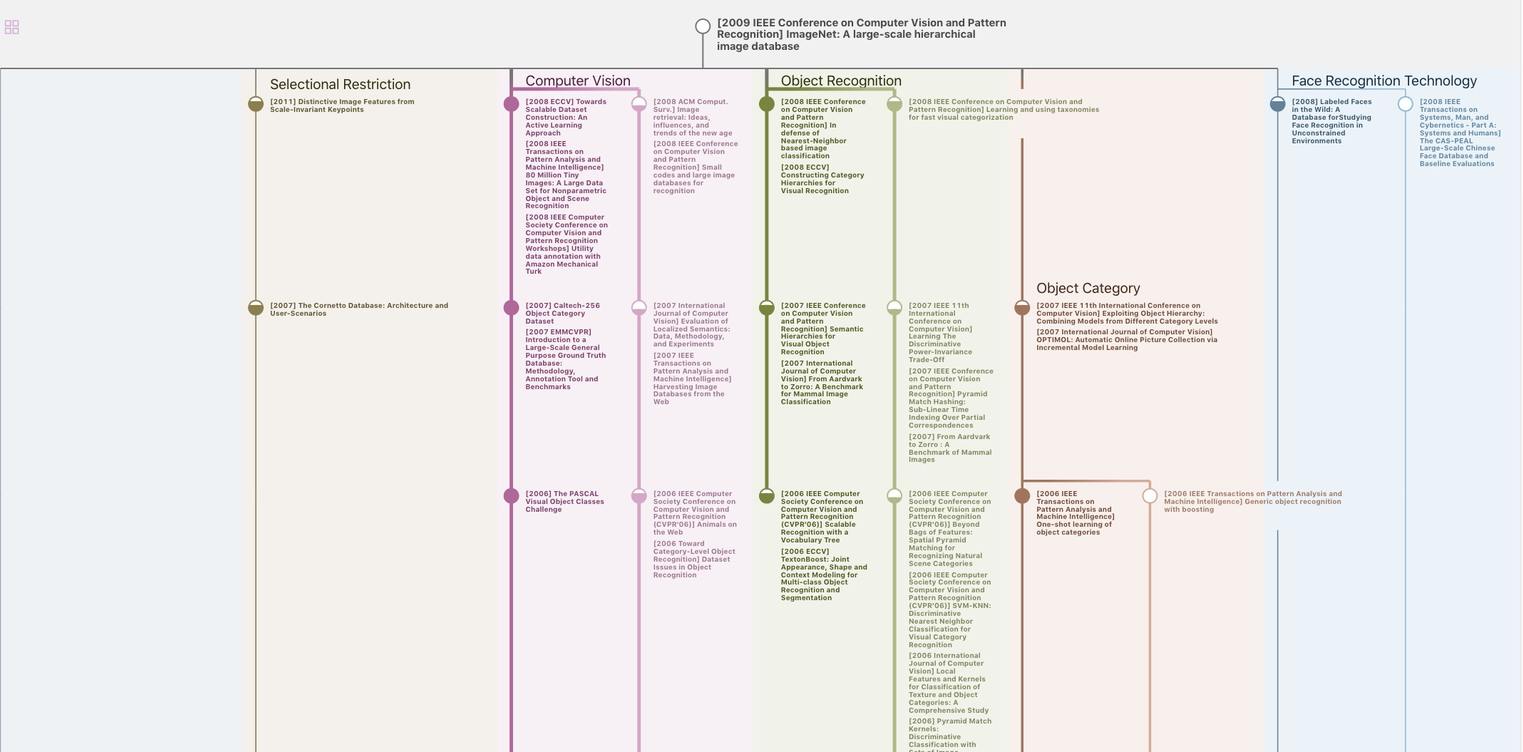
生成溯源树,研究论文发展脉络
Chat Paper
正在生成论文摘要