Mixed Membership Subspace Clustering
Data Mining(2013)
摘要
Clustering is one of the fundamental data mining tasks. While traditional clustering techniques assign each object to a single cluster only, in many applications it has been observed that objects might belong to multiple clusters with different degrees. In this work, we present a Bayesian framework to tackle the challenge of mixed membership clustering for vector data. We exploit the ideas of subspace clustering where the relevance of dimensions might be different for each cluster. Combining the relevance of the dimensions with the cluster membership degree of the objects, we propose a novel type of mixture model able to represent data containing mixed membership subspace clusters. For learning our model, we develop an efficient algorithm based on variational inference allowing easy parallelization. In our empirical study on synthetic and real data we show the strengths of our novel clustering technique.
更多查看译文
关键词
Bayes methods,belief networks,data mining,inference mechanisms,pattern clustering,variational techniques,Bayesian framework,data mining,mixed membership subspace clustering technique,object cluster membership degree,real data,synthetic data,variational inference,vector data,mixed membership clustering,model based clustering,subspace clustering,variational inference
AI 理解论文
溯源树
样例
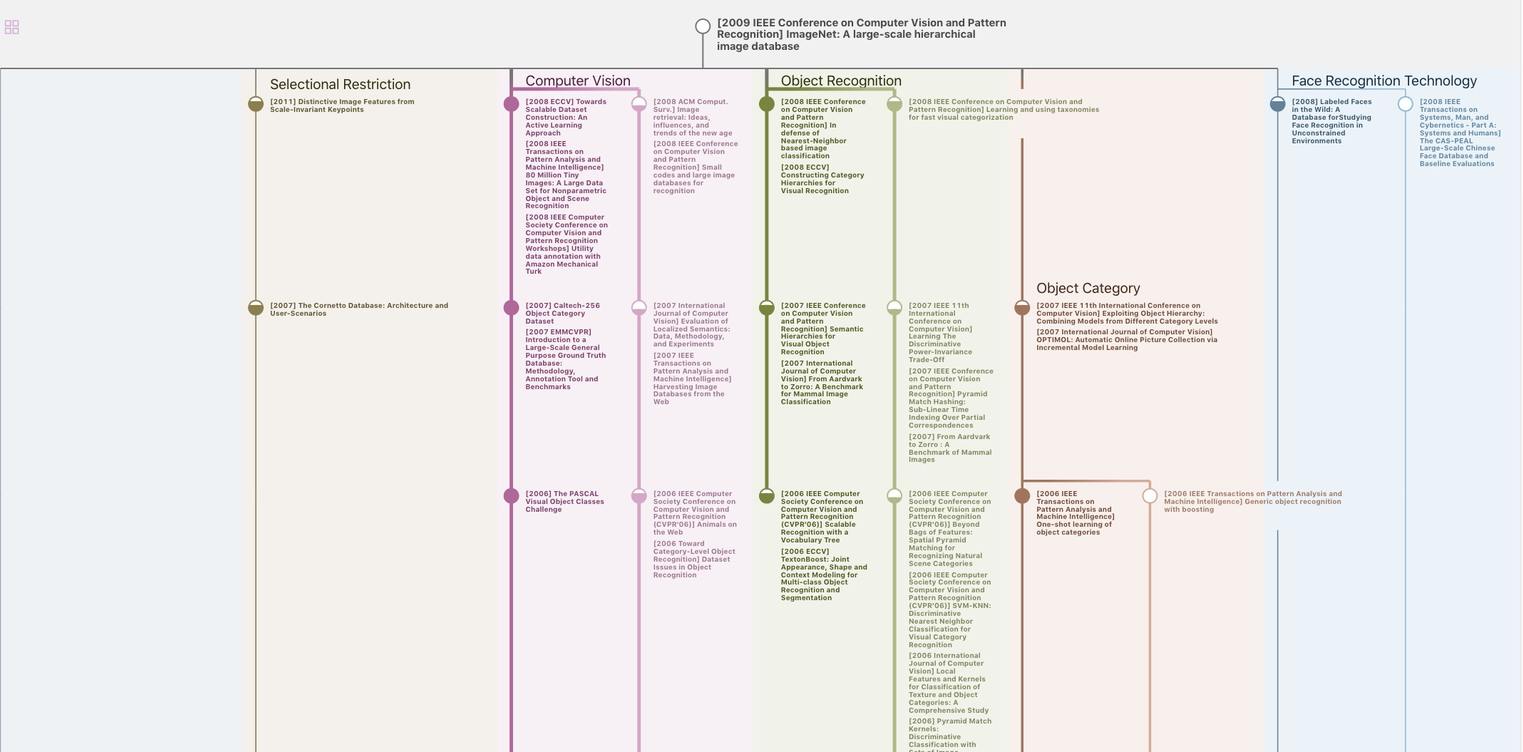
生成溯源树,研究论文发展脉络
Chat Paper
正在生成论文摘要