Phrasal Equivalence Classes For Generalized Corpus-Based Machine Translation
CICLing'11 Proceedings of the 12th international conference on Computational linguistics and intelligent text processing - Volume Part II(2011)
摘要
Generalizations of sentence-pairs in Example-based Machine Translation (EBMT) have been shown to increase coverage and translation quality in the past. These template-based approaches (G-EBMT) find common patterns in the bilingual corpus to generate generalized templates. In the past, patterns in the corpus were found by only few of the following ways: finding similar or dissimilar portions of text in groups of sentence-pairs, finding semantically similar words, or use dictionaries and parsers to find syntactic correspondences. This paper combines all the three aspects for generating templates. In this paper, the boundaries for aligning and extracting members (phrase-pairs) for clustering are found using chunkers (hence, syntactic information) trained independently on the two languages under consideration. Then semantically related phrase-pairs are grouped based on the contexts in which they appear. Templates are then constructed by replacing these clustered phrase-pairs by their class labels. We also perform a filtration step by simulating human labelers to obtain only those phrase-pairs that have high correspondences between the source and the target phrases that make up the phrase-pairs. Templates with English-Chinese and English-French language pairs gave significant improvements over a baseline with no templates.
更多查看译文
关键词
Generalized Example-based Machine Translation (G-EBMT),Template Induction,Unsupervised Clustering,data sparsity
AI 理解论文
溯源树
样例
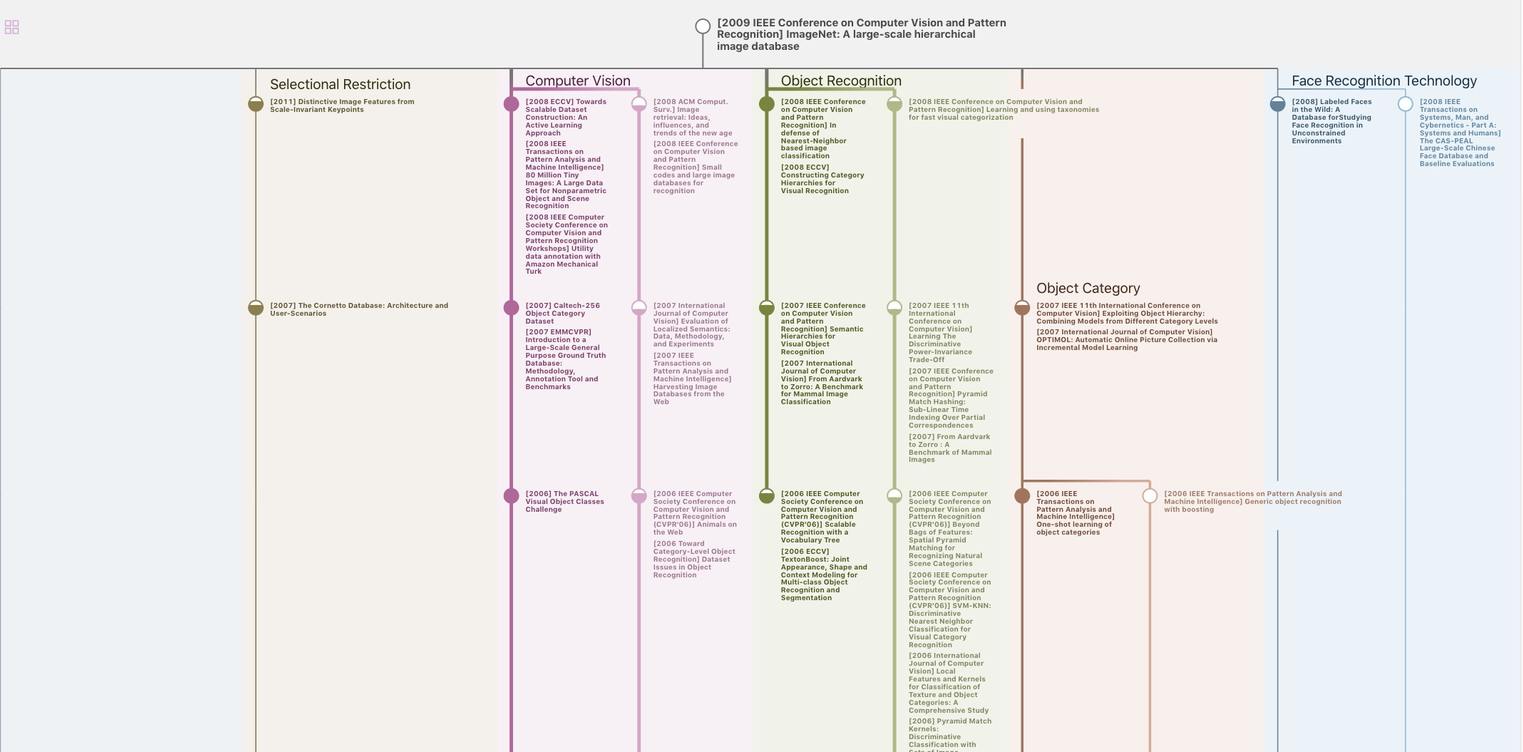
生成溯源树,研究论文发展脉络
Chat Paper
正在生成论文摘要