基本信息
浏览量:193
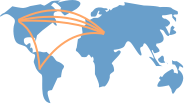
个人简介
I am broadly interested in statistical inference, informally defined as the computational process of turning data into statistics, prediction and understanding. I work with richly structured data, such as those extracted from texts, images and other spatiotemporal signals. In recent years I have gravitated toward a field in statistics known as Bayesian nonparametrics, which provides a fertile and powerful mathematical framework for the development of many computational and statistical modeling ideas. The spirit of Bayesian nonparametric statistics is to enable the kind of inferential processes according to which both the statistical modeling and computational complexity may adapt to increasingly large and complex data patterns in a graceful and effective way. In this framework, stochastic processes and random measures, along with latent variable models such as mixture, hierarchical and graphical models figure prominently. My motivation for all this came originally from an interest in machine learning, which continues to be a major source of research interest. A primary focus in my machine learning research is to develop more effective inference algorithms using variational, stochastic and geometric viewpoints.
研究兴趣
论文共 12 篇作者统计合作学者相似作者
按年份排序按引用量排序主题筛选期刊级别筛选合作者筛选合作机构筛选
时间
引用量
主题
期刊级别
合作者
合作机构
加载更多
作者统计
合作学者
合作机构
D-Core
- 合作者
- 学生
- 导师
数据免责声明
页面数据均来自互联网公开来源、合作出版商和通过AI技术自动分析结果,我们不对页面数据的有效性、准确性、正确性、可靠性、完整性和及时性做出任何承诺和保证。若有疑问,可以通过电子邮件方式联系我们:report@aminer.cn