Predicting uniaxial compressive strength of rocks using ANN models: Incorporating porosity, compressional wave velocity, and schmidt hammer data
Ultrasonics(2024)
摘要
The unconfined compressive strength (UCS) of intact rocks is crucial for engineering applications, but traditional laboratory testing is often impractical, especially for historic buildings lacking sufficient core samples. Non-destructive tests like the Schmidt hammer rebound number and compressional wave velocity offer solutions, but correlating these with UCS requires complex mathematical models. This paper introduces a novel approach using an artificial neural network (ANN) to simultaneously correlate UCS with three non-destructive test indexes: Schmidt hammer rebound number, compressional wave velocity, and open-effective porosity. The proposed ANN model outperforms existing methods, providing accurate UCS predictions for various rock types. Contour maps generated from the model offer practical tools for geotechnical and geological engineers, facilitating decision-making in the field and enhancing educational resources. This integrated approach promises to streamline UCS estimation, improving efficiency and accuracy in engineering assessments of intact rock materials.
更多查看译文
关键词
Artificial Neural Networks,Machine learning,Non-destructive testing,Rocks,Unconfined compressive strength
AI 理解论文
溯源树
样例
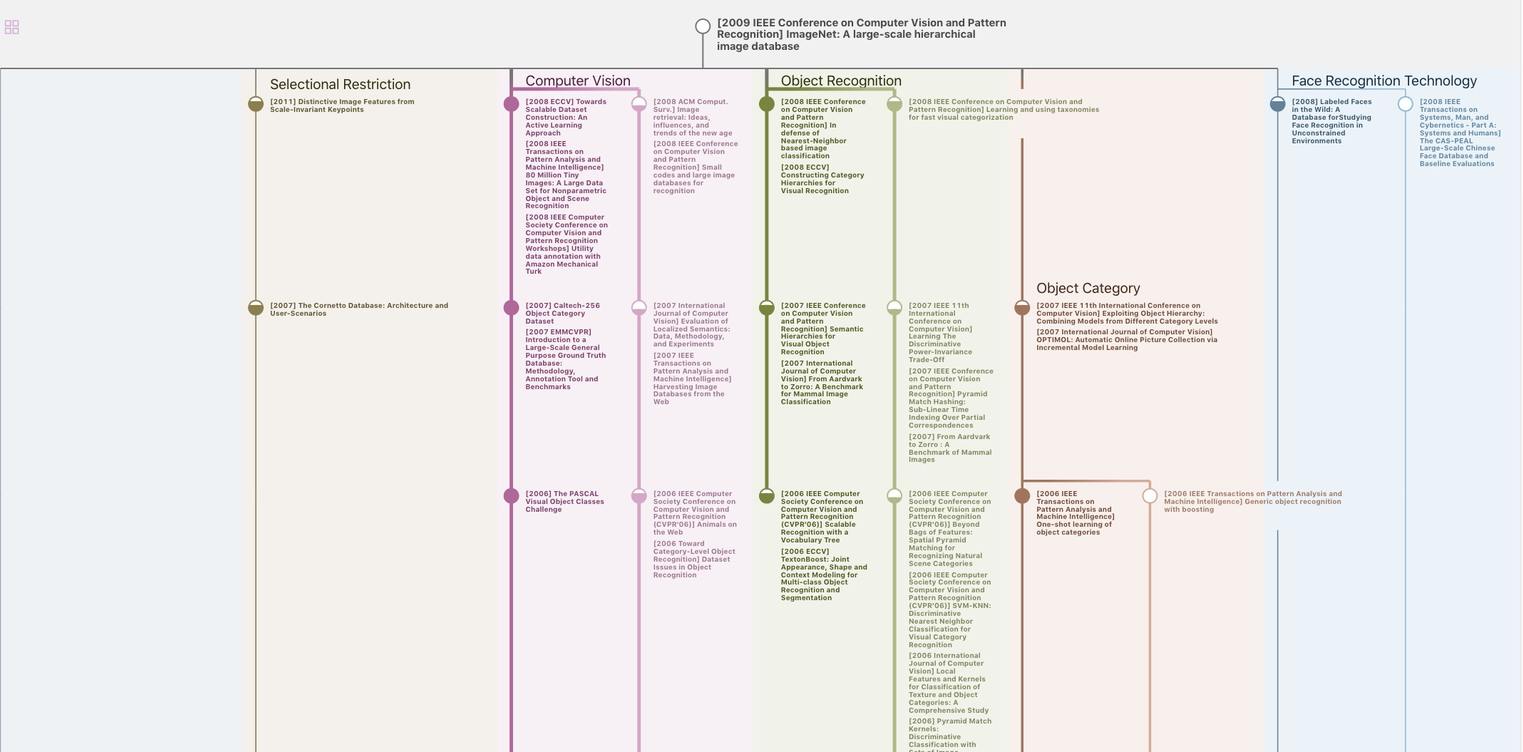
生成溯源树,研究论文发展脉络
Chat Paper
正在生成论文摘要