Simultaneous determination of pigments of spinach (Spinacia oleracea L.) leaf for quality inspection using hyperspectral imaging and multi-task deep learning regression approaches
Food Chemistry: X(2024)
摘要
Rapid and accurate determination of pigment content is important for quality inspection of spinach leaves during storage. This study aimed to use hyperspectral imaging at two spectral ranges (visible/near-infrared, VNIR: 400–1000 nm; NIR: 900–1700 nm) to simultaneously determine the pigment (chlorophyll a, chlorophyll b, total chlorophyll, and carotenoids) content in spinach stored at different durations and conditions (unpackaged and packaged). Partial least squares (PLS), back propagation neural network (BPNN) and convolutional neural network (CNN) were used to establish single-task and multi-task regression models. Single-task CNN (STCNN) models and multi-task CNN (MTCNN) models obtained better performances than the other models. The models using VNIR spectra were superior to those using NIR spectra. The overall results indicated that hyperspectral imaging with multi-task learning could predict the quality attributes of spinach simultaneously for spinach quality inspection under various storage conditions. This research will guide food quality inspection by simultaneously inspecting multiple quality attributes.
更多查看译文
关键词
Hyperspectral imaging,Spinach,Pigments,Multi-task regression,Convolutional neural networks
AI 理解论文
溯源树
样例
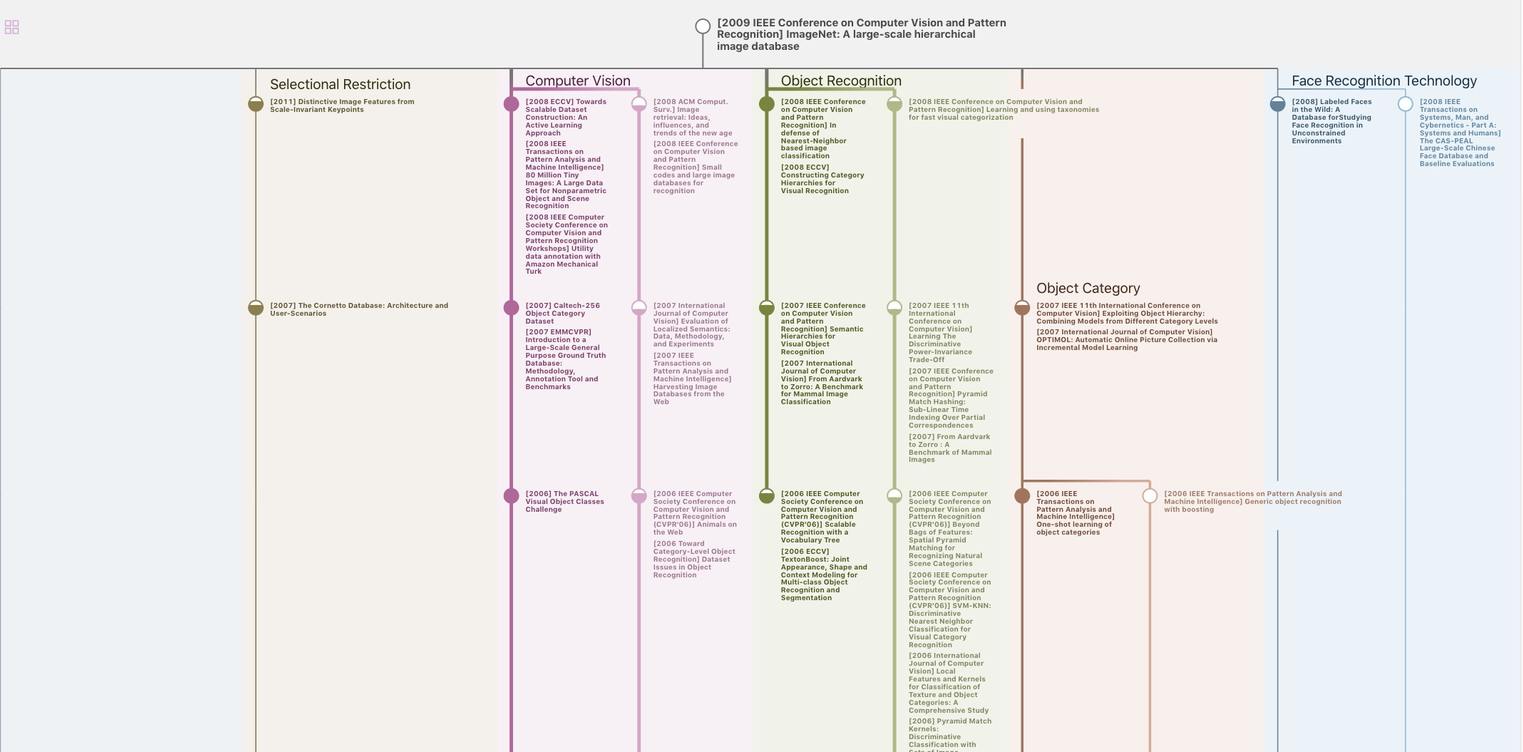
生成溯源树,研究论文发展脉络
Chat Paper
正在生成论文摘要