PopGR: Popularity reweighting for debiasing in group recommendation
World Wide Web(2024)
摘要
Like common recommender systems, group recommendation usually suffers from popularity bias where popular items are more likely to be suggested and exposed to users over long-tailed ones. The skewed data distribution caused discrimination against a great amount of unpopular items, which will be further intensified during the group decision-making process. Despite previous studies devoted to addressing popularity bias issue in recommendations, rarely have other works concentrated on such problem in group recommender systems. In this paper, we identify the negative impact of item popularity in a causality manner and propose a Popularity Reweighting Framework for Group Recommendation (PopGR). Specifically, a popularity-aware weighting function is adopted to mitigate the bias problem by incorporating the popularity level of items along with their intrinsic characteristics into group modeling. Experiments conducted on two real world benchmark datasets justify the effectiveness of our model to alleviate bias while maintaining reasonable ranking accuracy.
更多查看译文
关键词
Recommender systems,Group recommendation,Popularity bias,Fairness
AI 理解论文
溯源树
样例
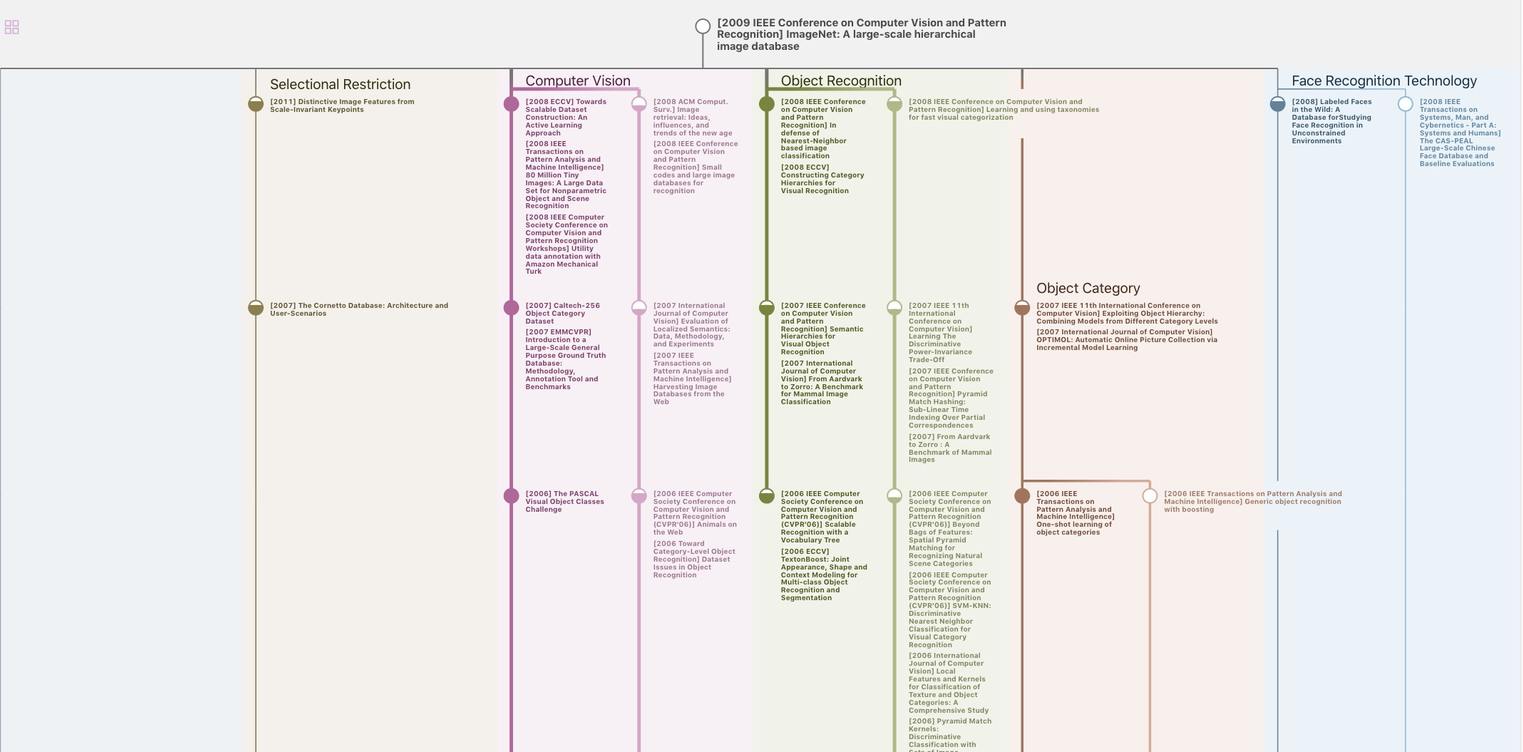
生成溯源树,研究论文发展脉络
Chat Paper
正在生成论文摘要