Predicting the Spectroscopic Features of Galaxies by Applying Manifold Learning on Their Broadband Colors: Proof of Concept and Potential Applications for Euclid, Roman, and Rubin LSST
The Astrophysical Journal(2024)
摘要
Entering the era of large-scale galaxy surveys, which will deliver unprecedented amounts of photometric and spectroscopic data, there is a growing need for more efficient, data-driven, and less model-dependent techniques to analyze the spectral energy distribution of galaxies. In this work, we demonstrate that by taking advantage of manifold learning approaches, we can estimate spectroscopic features of large samples of galaxies from their broadband photometry when spectroscopy is available only for a fraction of the sample. This will be done by applying the self-organizing map algorithm on broadband colors of galaxies and mapping partially available spectroscopic information into the trained maps. In this pilot study, we focus on estimating the 4000 Å break in a magnitude-limited sample of galaxies in the Cosmic Evolution Survey (COSMOS) field. We also examine this method to predict the H δ _A index given our available spectroscopic measurements. We use observed galaxy colors ( u,g,r,i,z,Y,J,H ), as well as spectroscopic measurements for a fraction of the sample from the LEGA-C and z COSMOS spectroscopic surveys to estimate this feature for our parent photometric sample. We recover the D4000 feature for galaxies that only have broadband colors with uncertainties about twice the uncertainty of the employed spectroscopic surveys. Using these measurements, we observe a positive correlation between D4000 and the stellar mass of the galaxies in our sample with weaker D4000 features for higher-redshift galaxies at fixed stellar masses. These can be explained by the downsizing scenario for the formation of galaxies and the decrease in their specific star formation rate as well as the aging of their stellar populations over this time period.
更多查看译文
关键词
Broad band photometry,Spectroscopy,Galaxy colors
AI 理解论文
溯源树
样例
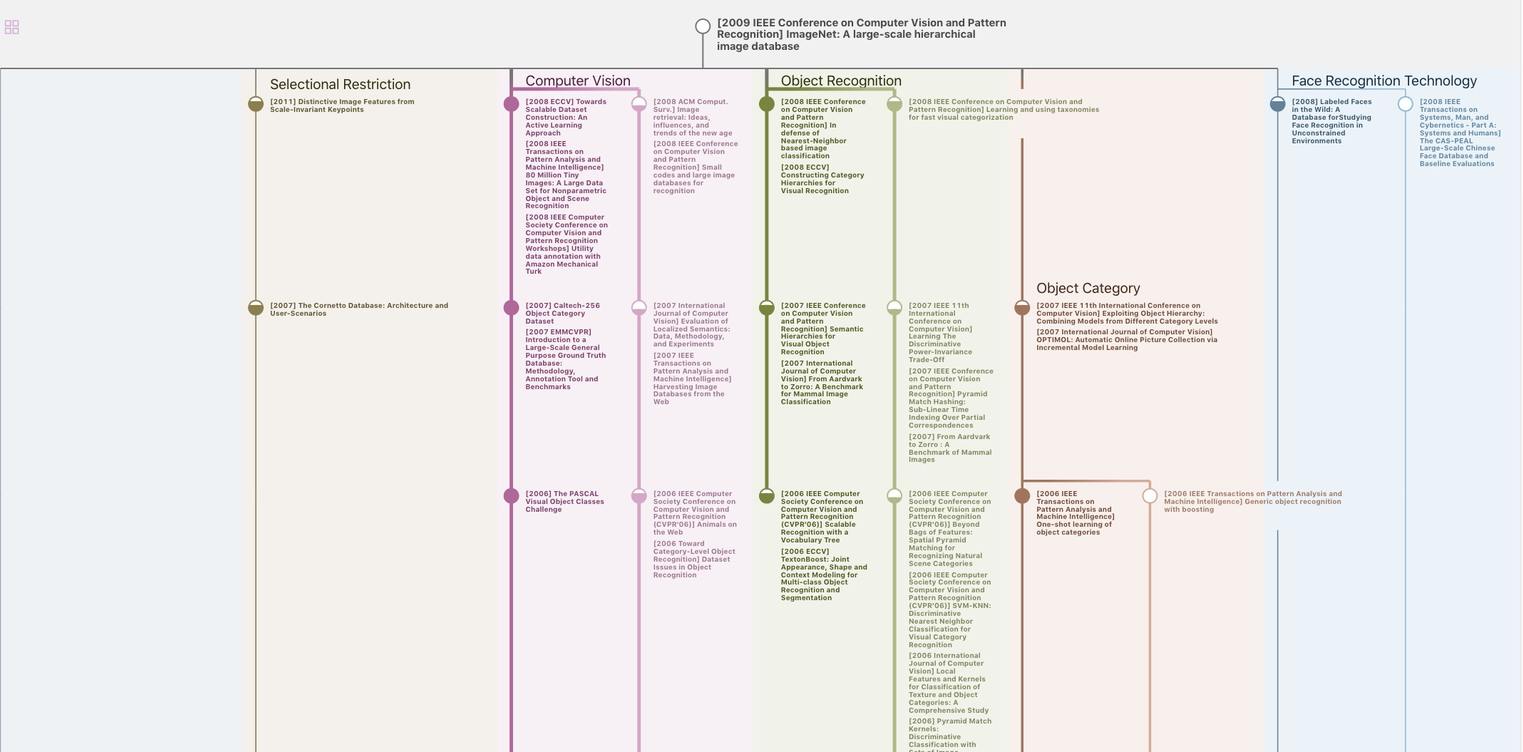
生成溯源树,研究论文发展脉络
Chat Paper
正在生成论文摘要