Safe Multiagent Learning With Soft Constrained Policy Optimization in Real Robot Control
IEEE TRANSACTIONS ON INDUSTRIAL INFORMATICS(2024)
Abstract
Due to a lack of safety considerations, a wide range of multiagent reinforcement learning (MARL) applications are limited in real-world environments. Thus, ensuring MARL safety is essential and urgent in the domain. However, merely a few studies consider the safe MARL problem, and the investigation of real-world applications using safe MARL algorithms still needs to be improved. To fill this gap, we provide a framework with soft constrained policy optimization, in which we develop practical algorithms to address the problem in a cooperative game setting. First, the problem formulation of safe MARL is introduced. Second, the safe policy optimization of safe MARL algorithms based on soft constrained optimization is analyzed, and we further propose a safe learning framework for safe MARL. The framework can be plugged into MARL algorithms without manually fine-tuning safety bounds. Third, we investigate the sim-to-real problems, and conduct simulation and real-world experiments to evaluate the effectiveness of our algorithms. Finally, the comprehensive experimental results indicate that our method has significant benefits regarding the balance between reward and safety performance and outperforms several strong baselines.
MoreTranslated text
Key words
Multiagent systems,real-robot control,safe reinforcement learning
AI Read Science
Must-Reading Tree
Example
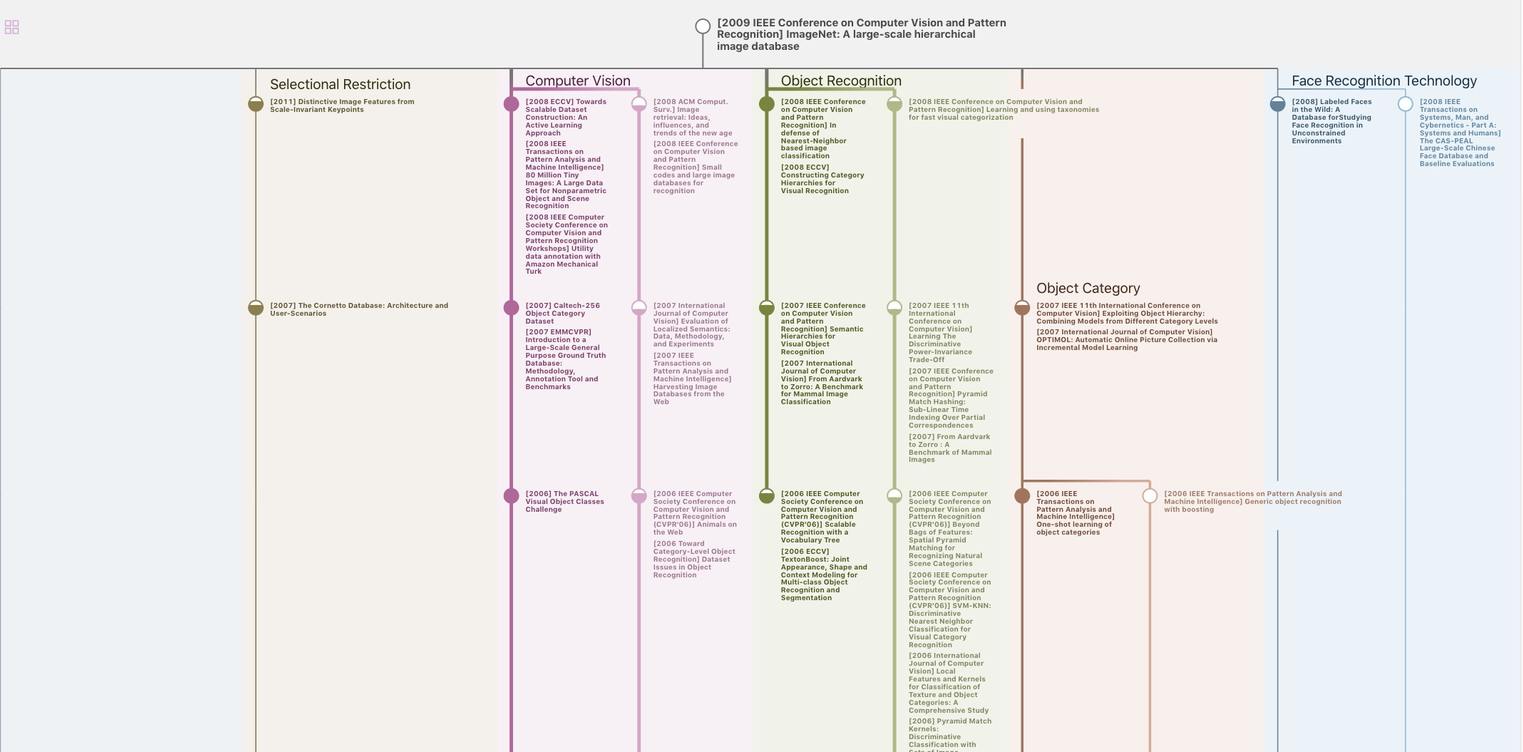
Generate MRT to find the research sequence of this paper
Chat Paper
Summary is being generated by the instructions you defined