A Robust Strategy of Geophysical Logging for Predicting Payable Lithofacies to Forecast Sweet Spots Using Digital Intelligence Paradigms in a Heterogeneous Gas Field
Natural Resources Research(2024)
摘要
The most crucial elements in the oil and gas sector are predicting subsurface lithofacies utilizing geophysical logs for reservoir characterization and sweet spot assessment procedures. Nevertheless, accurately predicting payable lithofacies in a complex heterogeneous geological setting, such as the lower goru formation, poses considerable difficulty because conventional methods fall short in delivering highly accurate outcomes. Hence, this research proposes an advanced cost and time-saving data intelligence strategy using multiple classifiers to predict lithofacies with maximum accuracy that will aid in sweet spot evaluation in oil and gas fields globally. Geophysical log data of five wells from a mature gas field were used. The targeted reservoir formation was classified into seven facies types. We evaluated the performance of seven different models: support vector machine (SVM), K-nearest neighbors (KNN), random forest (RF), decision tree (DTr), naive Bayes (NB), adaptive boosting (AB), and ensemble (an integrated SVM, KNN, RF, and DTr classifier). RF and ensemble classifiers predicted the lithofacies with accuracies of 97.5 and 97.3
更多查看译文
关键词
Payable lithofacies,Heterogeneous reservoir,Random forest,Ensemble-based method,Sweet spot forecasting
AI 理解论文
溯源树
样例
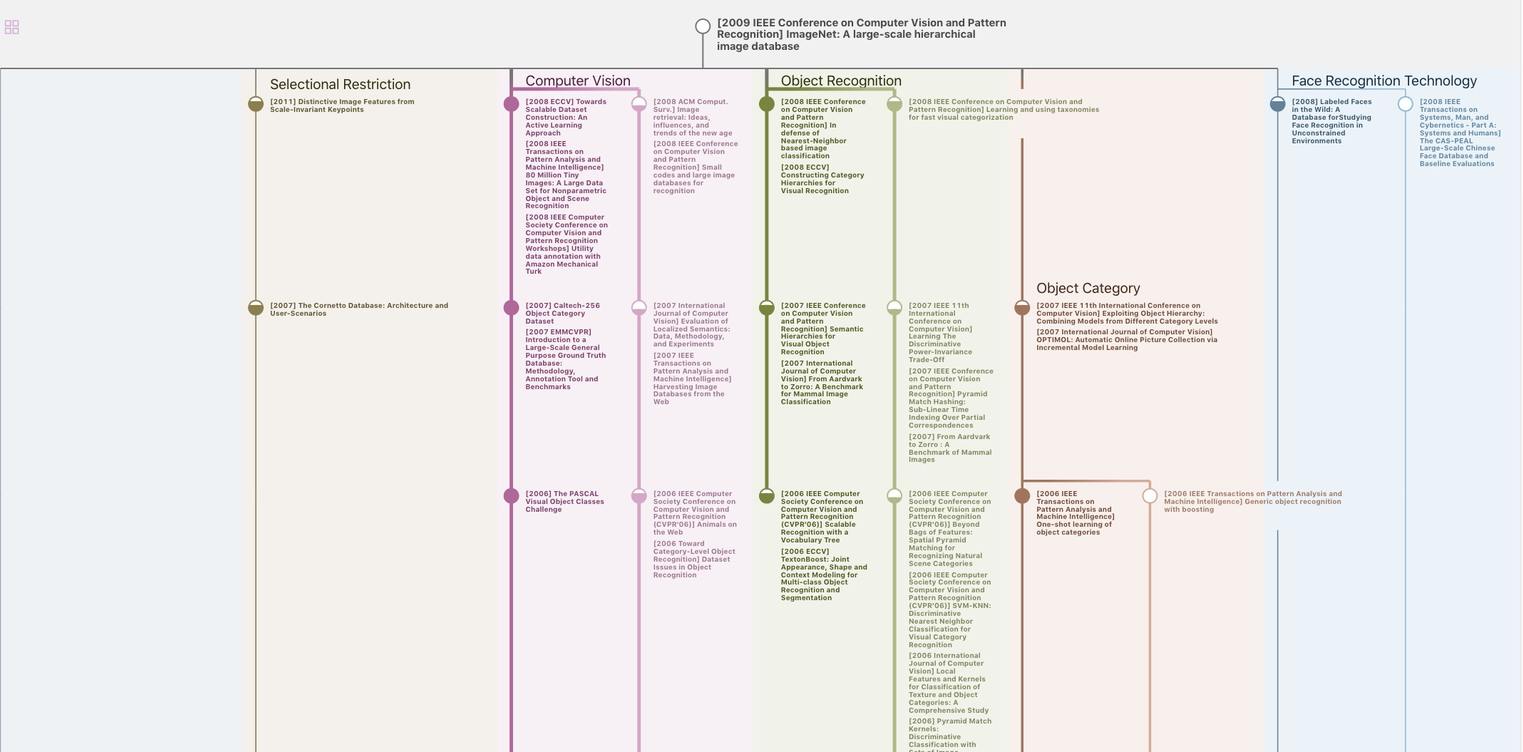
生成溯源树,研究论文发展脉络
Chat Paper
正在生成论文摘要