Robust Synthetic-to-Real Ensemble Dehazing Algorithm With the Intermediate Domain
IEEE Transactions on Computational Social Systems(2024)
摘要
Learning-based dehazing methods using synthetic datasets cannot generalize well on real-world hazy images due to the large domain discrepancy. To tackle this issue, we propose a robust synthetic-to-real dehazing framework with the construction of an intermediate domain and ensemble learning strategy. First, by mapping all examples to the intermediate domain, the bidirectional match strategy with adversarial training and the constraint of intermediated results is proposed to suppress the rich domain-specific information, which can facilitate the adaptation and perform image dehazing simultaneously. Furthermore, an ensemble dehazing algorithm based on the intermediate domain is proposed in a semisupervised manner. The reconstruction constraint and the enhanced ground-truths are employed to keep the visual fidelity and remove the dim artifacts of unsupervised dehazing results. Finally, we propose the domain-aware residual groups to deal with the distribution discrepancy between the synthetic and real hazy images. Extensive experiments of various real-world hazy images demonstrate that the proposed method outperforms the state-of-the-art dehazing methods and significantly improves the generalization in the real world.
更多查看译文
关键词
Domain discrepancy,ensemble learning,intermediate domain,semisupervised
AI 理解论文
溯源树
样例
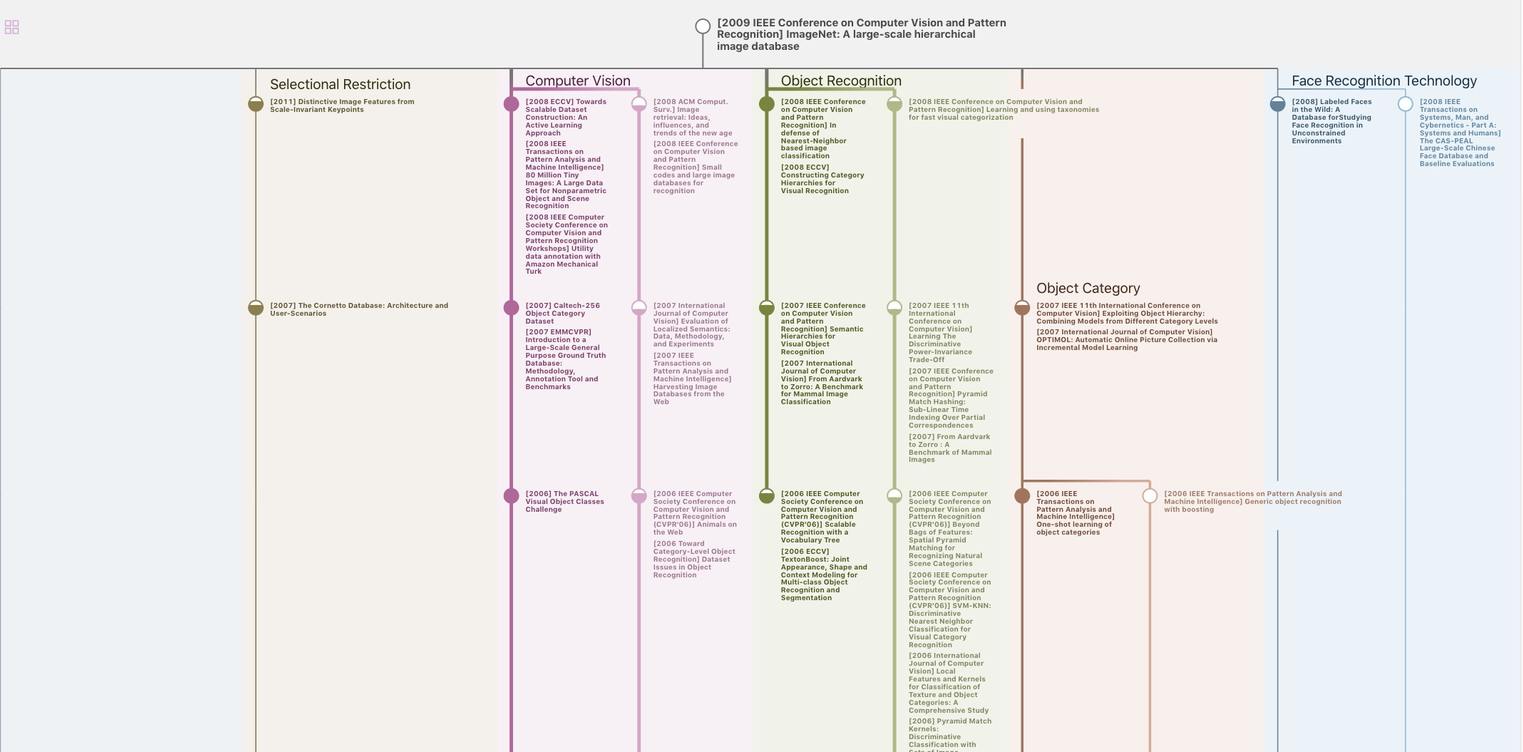
生成溯源树,研究论文发展脉络
Chat Paper
正在生成论文摘要