A Practical Guide for Noise Characterisation of Back Pressure Sensors: Towards Digital Twin for an Industrial High Horse Power Engine Test Cell
IEEE Sensors Letters(2024)
摘要
Extensive testing is crucial to ensure exhaust emission compliance for high-horsepower (H-hp) commercial engines. Back pressure sensors, integral to the exhaust system, are prone to producing noisy measurements due to the turbulent nature of the exhaust gas flow and other testing inaccuracies, mandating the use of a noise reduction filter. The time-consuming process of filter tuning, required to remove excessive process/measurement noise, often involves trialand-error. This practice, entailing numerous experiments with live engines, results in high financial costs and emissions due to challenges in extracting the ground truth signal from noisy measurements. Developing a digital twin (DT) of this system is proposed to expedite filter tuning, hence reducing cost and emissions output. Creating such a DT necessitates a method of back pressure sensor noise classification to accurately simulate the signal. This letter introduces a step-by-step procedure for characterising the H-hp engine back pressure sensor through statistical measures, leading to the development of the DT. This letter demonstrates the potential of this approach in an industrial case study, showcasing its viability for application in engine test-bed facilities and across industries. The economic calculation estimates a potential £184,400 reduction in diesel fuel costs and 321,600 kg of CO2 emissions by tuning the filter through the DT compared to current industrial practice
更多查看译文
关键词
Sensor applications,sensor signal processing,noise characterisation,filter,digital twin,diesel engine,back pressure
AI 理解论文
溯源树
样例
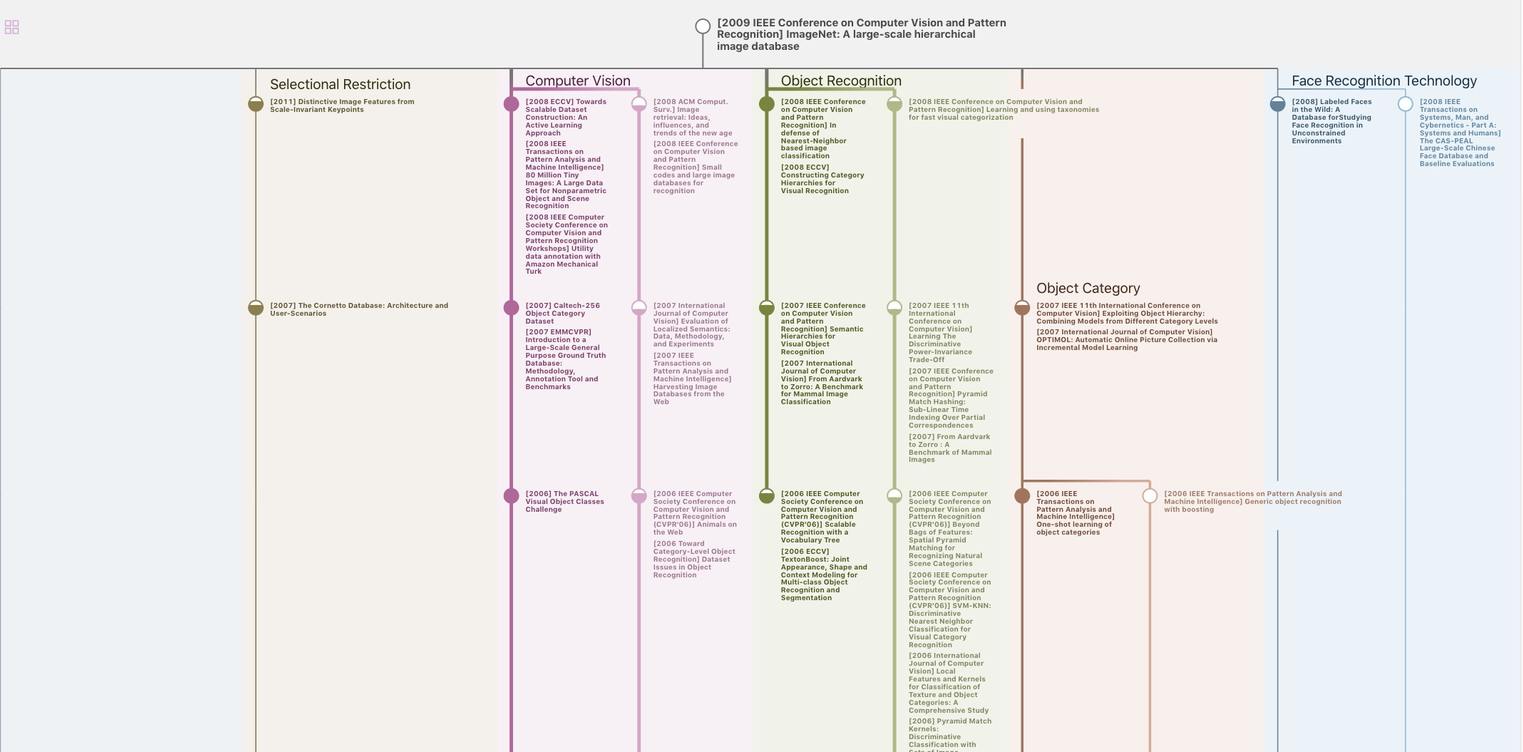
生成溯源树,研究论文发展脉络
Chat Paper
正在生成论文摘要