Advancing nearshore and onshore tsunami hazard approximation with machine learning surrogates
crossref(2024)
摘要
Abstract. Probabilistic tsunami hazard and risk assessment (PTHA and PTRA) are vital methodologies for computing tsunami risk and prompt measures to mitigate impacts. At large regional scales, their use and scope are currently limited by the computational costs of numerically intensive simulations behind them, which may be feasible only with advanced computational resources like high-performance computing (HPC) and may still require reductions in resolution, number of scenarios modelled, or use of simpler approximation schemes. To conduct PTHA and PTRA for large proportions of the coast, we therefore need to develop concepts and algorithms for reducing the number of events simulated and for more efficiently approximating the needed simulation results. This case study for a coastal region of Tohoku, Japan, utilises a limited number of tsunami simulations from submarine earthquakes along the subduction interface to build a wave propagation and inundation database and fits these simulation results through a machine learning-based variational encoder-decoder model. This is used as a surrogate to predict the tsunami waveform at the coast and the maximum inundation depths onshore at the different test sites. The performance of the surrogate models was assessed using a 5-fold cross validation assessment across the simulation events. Further to understand its real world performance and test the generalisability of the model, we used 5 very different tsunami source models from literature for historic events to further benchmark the model and understand its current deficiencies.
更多查看译文
AI 理解论文
溯源树
样例
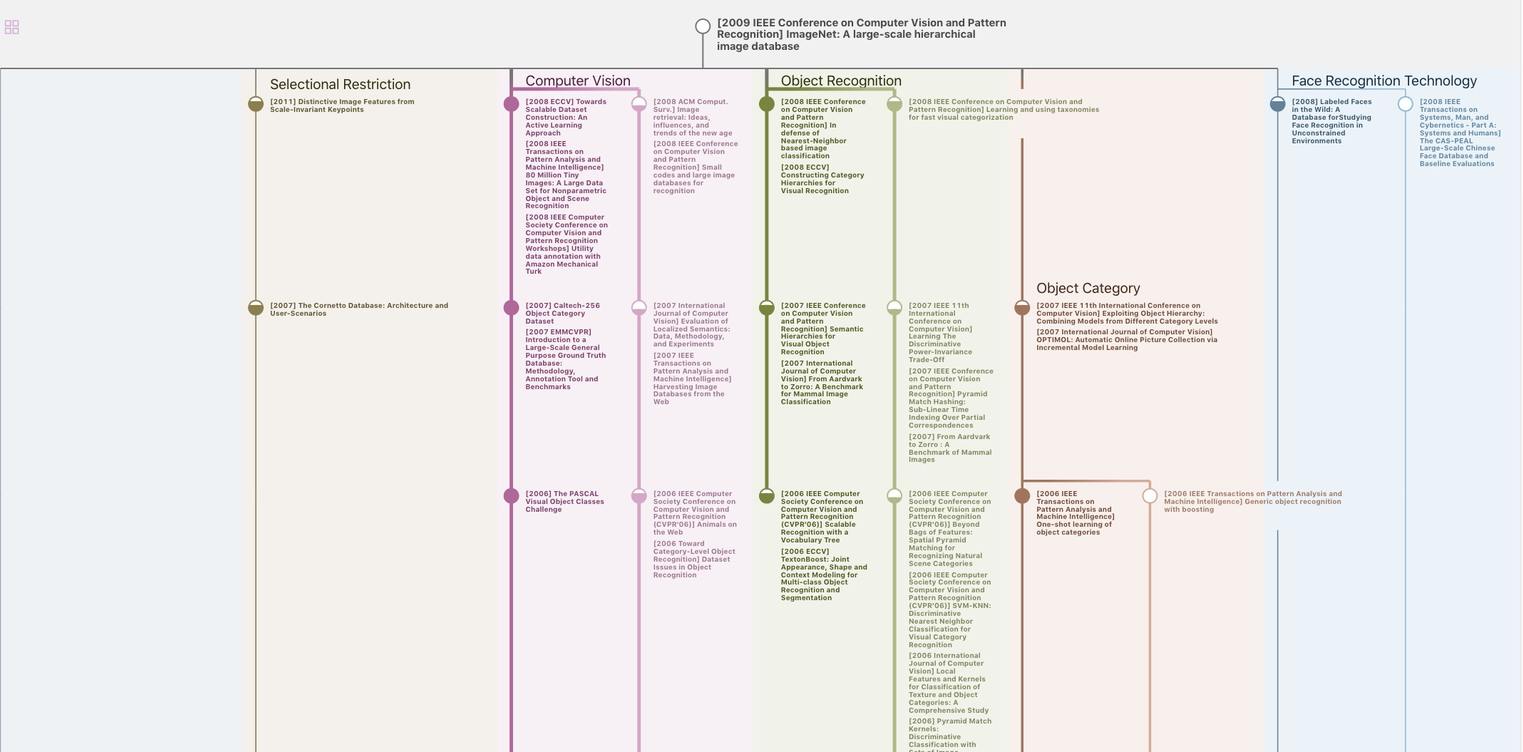
生成溯源树,研究论文发展脉络
Chat Paper
正在生成论文摘要