Automated identification of aquatic insects: A case study using deep learning and computer vision techniques
Science of The Total Environment(2024)
摘要
Despite huge attention in other domains, deep learning is only very slowly beginning to be applied in biodiversity research. Mayflies (Ephemeroptera), stoneflies (Plecoptera) and caddisflies (Trichoptera), often abbreviated as EPT, are frequently used for freshwater biomonitoring due to their large numbers and sensitivity to environmental changes. However, the unambiguous morphological identification of EPT species is a challenging, but fundamental task. Morphological identification of these freshwater insects is therefore not only extremely time-consuming and costly, but also often leads to misjudgments or generates datasets with low taxonomic resolution. Here, we investigated the application of deep learning to increase the efficiency and taxonomic resolution of biomonitoring programs. Our database contains 90 EPT taxa (genus or species level), with the number of images per category ranging from 21 to 300 (16,650 in total). Upon completion of training, a CNN (Convolutional Neural Network) model was created, capable of automatically classifying these taxa into their appropriate taxonomic categories with an accuracy of 98.7 %. For the extensive set of 68 tested taxa, our model achieved a perfect classification rate of 100 %. We achieved noteworthy classification accuracy with morphologically closely related taxa within the training data (e.g., species of the genus Baetis, Hydropsyche, Perla). Gradient-weighted Class Activation Mapping (Grad-CAM) visualized the morphological features responsible for the classification of the treated species in the CNN models. In the Ephemeroptera, the head was the most important feature, while the thorax and abdomen were equally important for the classification of Plecoptera taxa. For the order Trichoptera, the head and thorax were almost equally important. Our database is recognized as the most extensive aquatic insect database, notably distinguished by its wealth of included categories (taxa). Our approach can help solve long-standing challenges in biodiversity research and address pressing issues in monitoring programs by saving time in sample and data processing.
更多查看译文
关键词
Biomonitoring,Ephemeroptera,Plecoptera,Trichoptera,Artificial intelligence
AI 理解论文
溯源树
样例
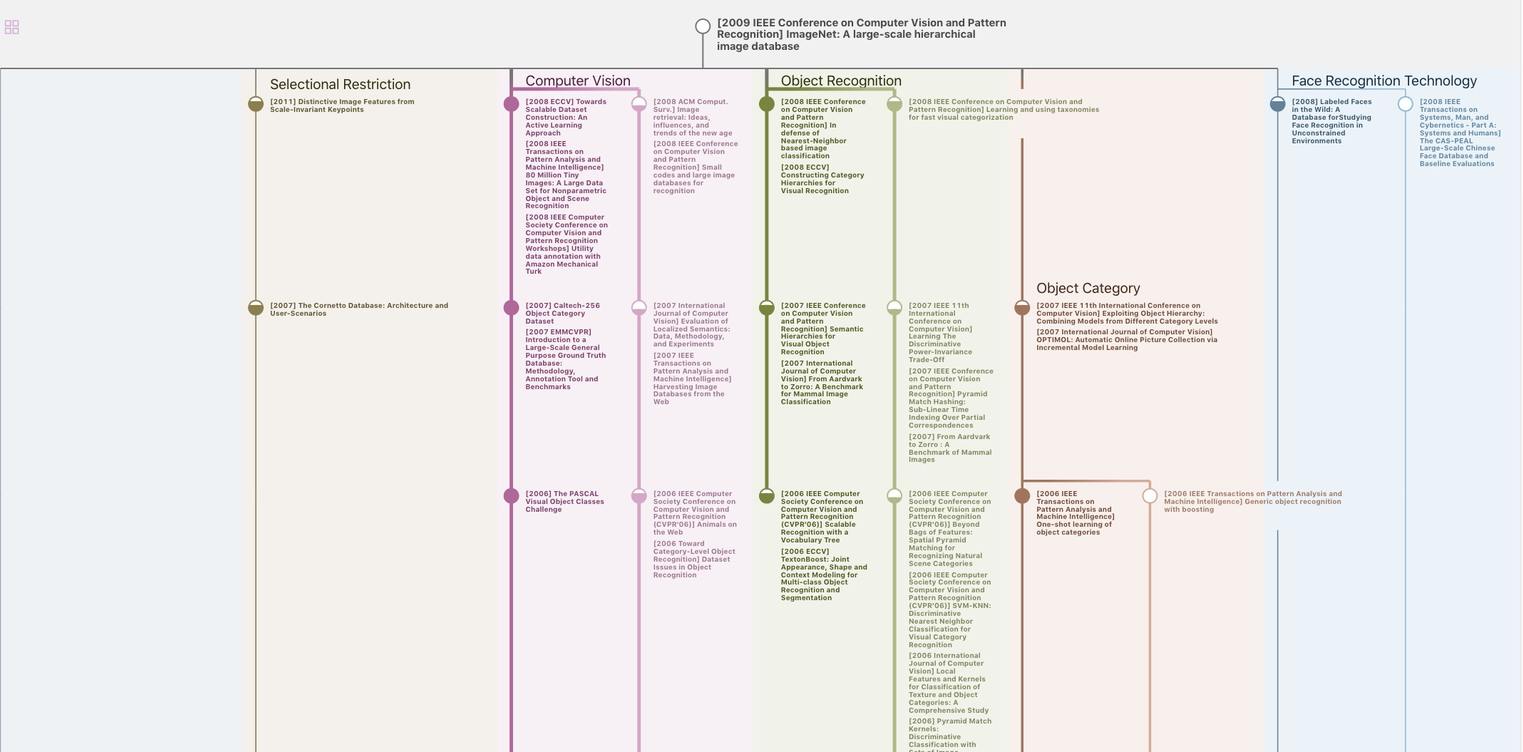
生成溯源树,研究论文发展脉络
Chat Paper
正在生成论文摘要