Observation Weights Matching Approach for Causal Inference
Pattern Recognition(2024)
摘要
This study introduces a novel method integrating pattern recognition models with causal inference methodologies to adeptly identify and manage overlapping regions between treatment and control groups. Our approach, Observation Weights Matching (OWM), addresses the intrinsic challenges in observational studies—specifically, the fixed sample size and the lack of complete overlap in pretreatment variables. Through ensemble learning, OWM effectively retains examples within these critical overlapping regions, systematically generating weighted data distributions that aid in the precise identification of these instances. By prioritizing hard-to-classify observations and employing a novel metric of critical values for matched samples, our approach optimizes matching performance and provides greater robustness in causal analysis. Through empirical and simulation studies, we demonstrate OWM's notable advantage over traditional matching methods, enhancing causal inference in observational research. Furthermore, we show that OWM provides richer balance scores than propensity scores, ensuring unbiased estimations and advancing the field significantly.
更多查看译文
关键词
Ensemble learning,LogitBoost,AdaBoost,Overlapping regions,Observation weights,Propensity score matching
AI 理解论文
溯源树
样例
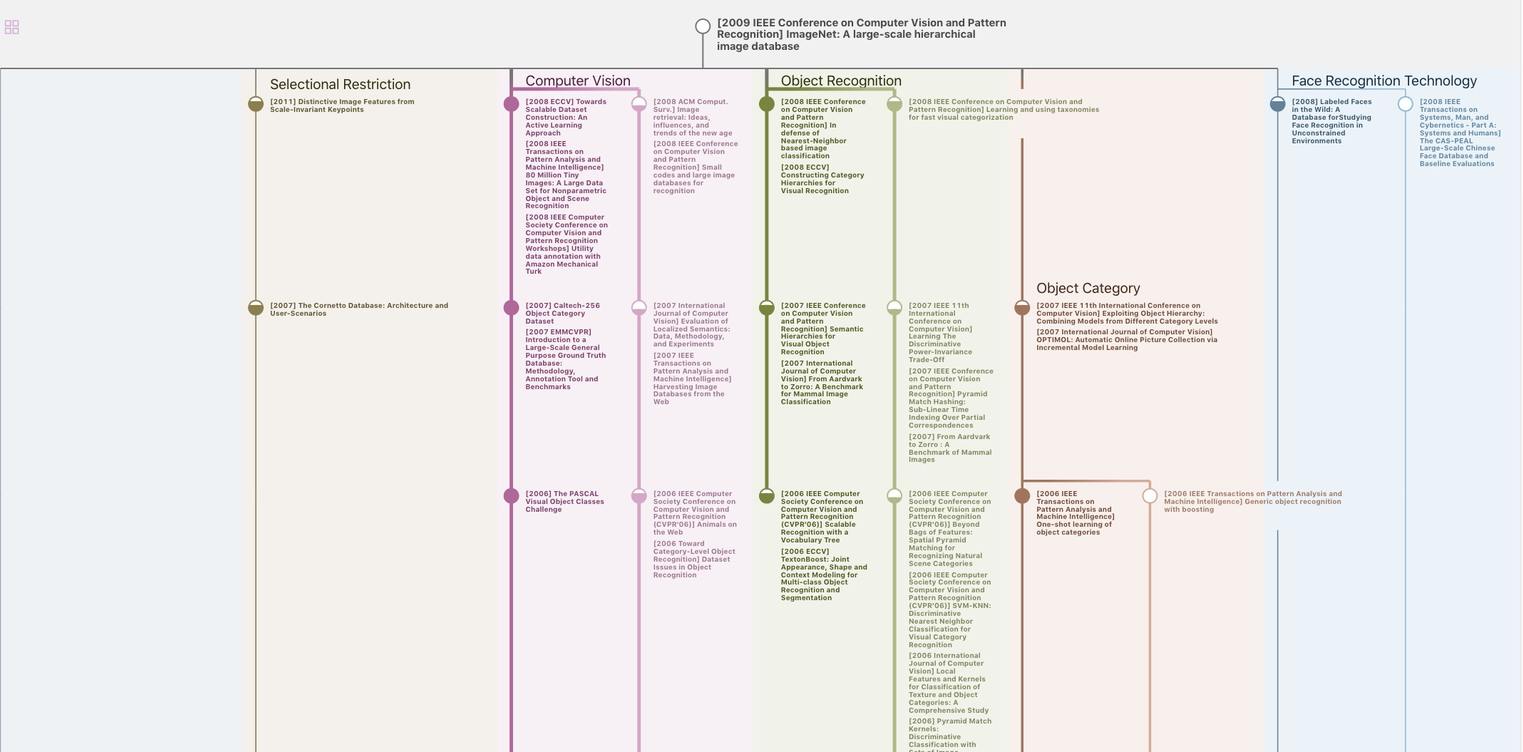
生成溯源树,研究论文发展脉络
Chat Paper
正在生成论文摘要