A privacy-preserving federated learning protocol with a secure data aggregation for the Internet of Everything
Computer Communications(2024)
摘要
Although there are significant advantages to the popular use of connected devices on the Internet of Everything, there remain distinct concerns surrounding privacy. Federated learning has been one of the solutions suggested to remediate such issues and looks promising given the collaborative model it provides, which is shared across local gradients Grds without the exposure of raw data. However, the shared Grds threaten the local data with a privacy risk. The central server could produce summarized results. Besides, in federated learning devices with resource constraints frequently dropout. There are only two possible solutions now: efficiency or privacy preservation. Designing a verifiable secure aggregation on the scale of federated learning is still a difficult test. Here, an efficient privacy-preserving federated learning protocol is proposed, with a secure data aggregation for the Internet of Everything. Aggregator encryption effectively masks the local Grds of clients. The central server aggregates the obscured Grds without exposing the local data. At the same time, clients can effectively verify whether the aggregated result is correct. Secondly, the suggested protocol employs a group management mechanism to tolerate the dropout of clients without negatively affecting their further participation in subsequent learning activities. Security analysis shows that the protocol guarantees privacy-preserving federated learning’s security demand. The results of experiments carried out on datasets reveal the highly efficient practical performance of the proposed protocol.
更多查看译文
关键词
Internet of Everything (IoE),Smart systems,Secure aggregation,Machine learning,Privacy preservation,Security
AI 理解论文
溯源树
样例
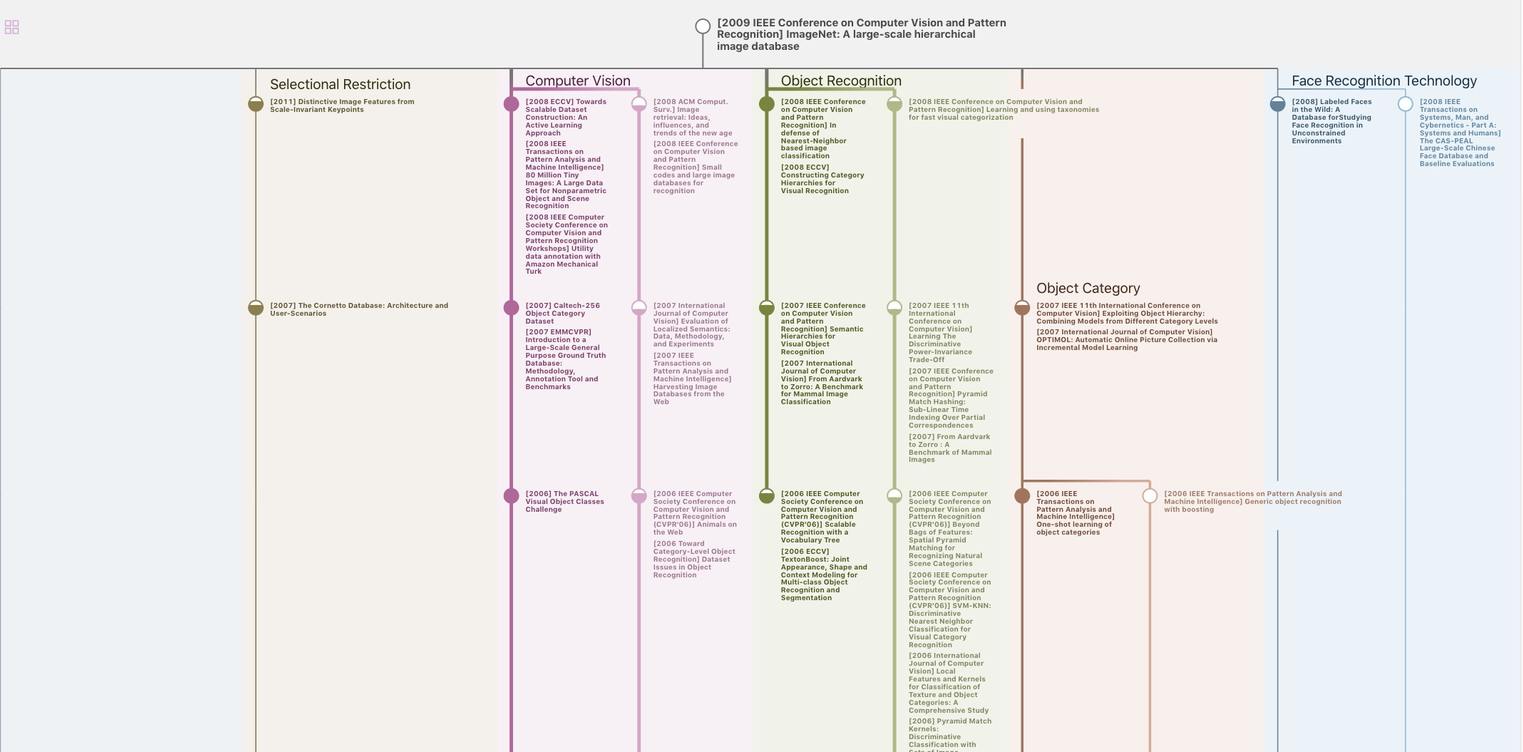
生成溯源树,研究论文发展脉络
Chat Paper
正在生成论文摘要