A Lithium Battery SOC Estimation Method Based on AEKF-Composite Neural Network Hybrid Driven Model
2023 IEEE 7th Conference on Energy Internet and Energy System Integration (EI2)(2023)
摘要
Lithium-ion battery State of Charge signifies the battery's remaining usable capacity and significantly impacts the stability and efficiency of new energy systems. Accurate State of charge (SOC) estimation is critical as renewable energy integration into the grid increases. This study explores a method for precise SOC estimation in lithium-ion batteries. We utilize the adaptive extended Kalman filter algorithm (AEKF), a model-based approach that adjusts noise covariance matrices based on measurement residuals, enhancing both accuracy and robustness in SOC estimation. Compared to the conventional extended Kalman filter, which assumes constant noise covariance matrices, the adaptive version demonstrates superior SOC estimation performance. Additionally, we introduce a self-correcting composite neural network prediction module, a data-driven technique that improves SOC estimation accuracy and robustness. It leverages historical data and model-based estimations to train a neural network capable of correcting estimation errors. Our proposed method combines the strengths of both model-based and data-driven approaches, and experimental results confirm its effectiveness and superiority.
更多查看译文
关键词
SOC estimation,AEKF,data-driven model
AI 理解论文
溯源树
样例
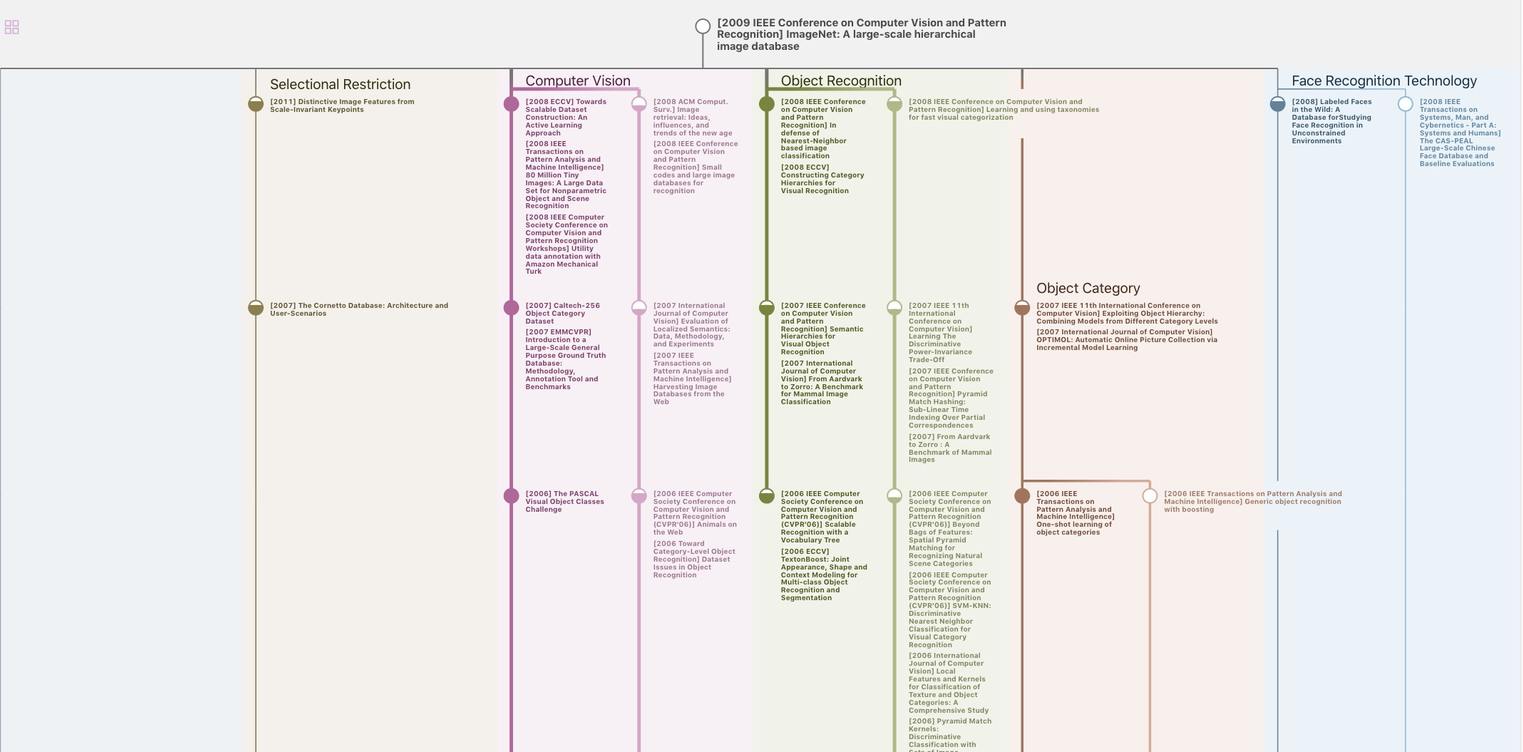
生成溯源树,研究论文发展脉络
Chat Paper
正在生成论文摘要