A Novel Multi-Scale Feature Map Fusion for Oil Spill Detection of SAR Remote Sensing
Remote Sensing(2024)
摘要
The efficient and timely identification of oil spill areas is crucial for ocean environmental protection. Synthetic aperture radar (SAR) is widely used in oil spill detection due to its all-weather monitoring capability. Meanwhile, existing deep learning-based oil spill detection methods mainly rely on the classical U-Net framework and have achieved impressive results. However, SAR images exhibit high noise, blurry boundaries, and irregular shapes of target areas, as well as speckles and shadows, which lead to the loss of performance in existing algorithms. In this paper, we propose a novel network architecture to achieve more precise segmentation of oil spill areas by reintroducing rich semantic contextual information before obtaining the final segmentation mask. Specifically, the proposed architecture can re-fuse feature maps from different levels at the decoder end. We design a multi-convolutional layer (MCL) module to extract basic feature information from SAR images, and a feature extraction module (FEM) module further extracts and fuses feature maps generated by the U-Net decoder at different levels. Through these operations, the network can learn rich global and local contextual information, enable sufficient interaction of feature information at different stages, enhance the model’s contextual awareness, and improve its ability to recognize complex textures and blurry boundaries, thereby enhancing the segmentation accuracy of SAR images. Compared to many U-Net based segmentation networks, our method shows promising results and achieves state-of-the-art performance on multiple evaluation metrics.
更多查看译文
关键词
oil spill detection application,multi-source remote sensing classification system,multi-scale feature fusion,synthetic aperture radar
AI 理解论文
溯源树
样例
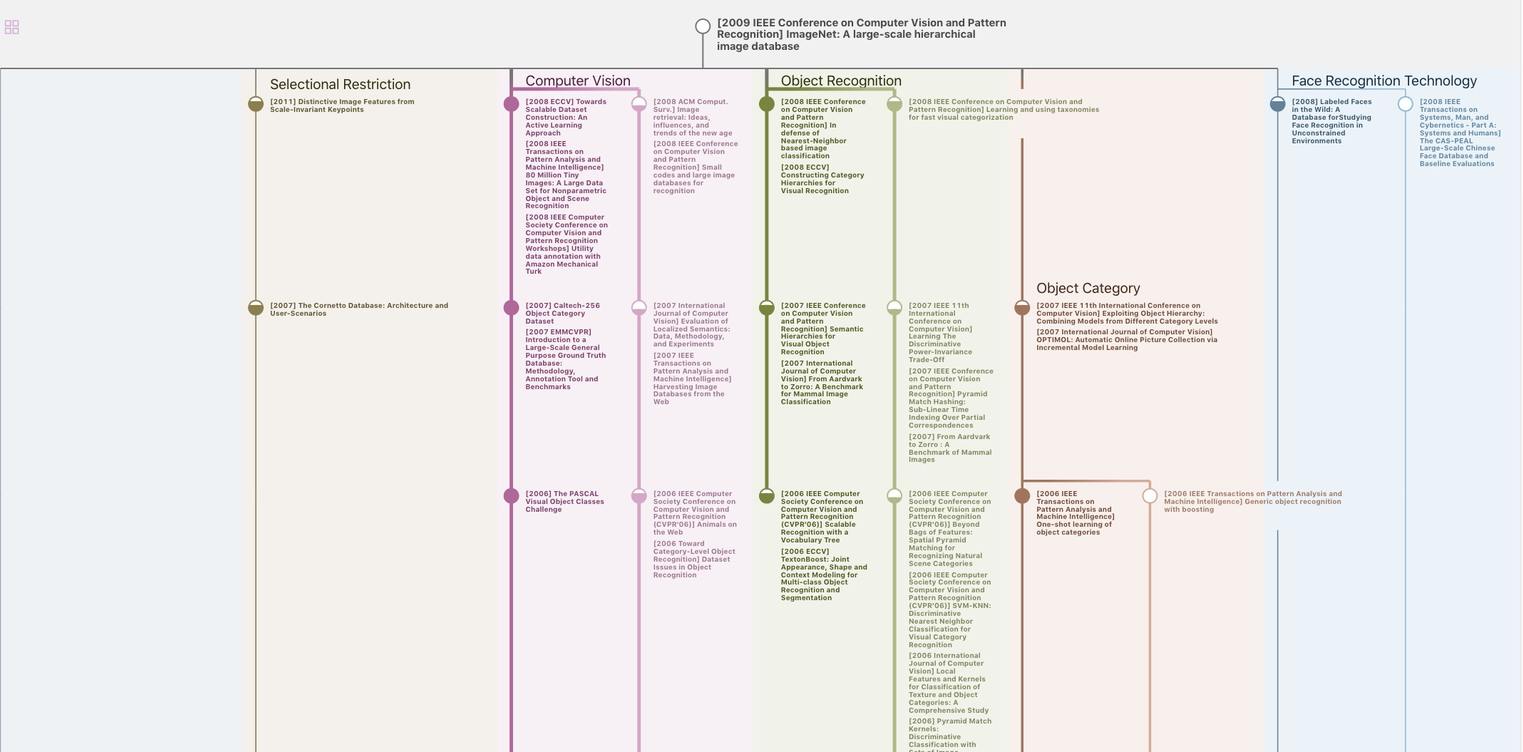
生成溯源树,研究论文发展脉络
Chat Paper
正在生成论文摘要